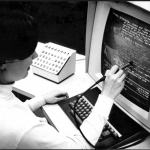
In his response to John Cayley, Dougherty takes the current concern with AIwriting as an opportunity to revive one of ebr's long-running threads; namely: the critical, contrarian riPOSTe.
John Cayley’s chief concerns in “Modelit: eliterature à la (language) model(l)” deal with the pressure of transformer-based models like the GPTs on our current conceptualizations of literature and the literary, eliterature, language, text, hermeneutics, and what Cayley refers to, within a legal framework, as “the moral rights of language makers.” His essay is fascinating and timely. It extends and elaborates upon the AI ruminations of other very recent contributors to ebr, as in Ian Demsky and his account of AI image experimentation in “My Month with Midjourney” (April 2, 2023): “The arrival of AI feels like a printing press moment, a mechanical reproduction moment, an analog-to-digital moment.” Or David Heckman’s “Thoughts on the Textpocalypse”(May 7, 2023), which riffs on Matthew Kirschenbaum’s dystopian Atlantic essay “The Textpocalypse”: “As we abandon the grave difficulty of community formations, we will grow accustomed to seeing slick but shallow pantomimes of competence as preferable and will yearn for the AI that can give us what we want.” Or David Thomas Henry Wright’s “Review of My Life as an Artificial Creative Intelligence (2022)” (May 7, 2023), which takes up the new book by Mark Amerika: “It is…mysticism that excites Amerika and should excite us all about the potential ‘potential’ literature when one dabbles in AI text generation.” As these various accounts suggest, there is so much to say and to think about this explosive moment in time, when AI of the transformer sort suddenly opens up a dizzying multiplicity of possible futures for human labor and aesthetic creativity—some good, some middling, some very bad.
Such transformer models are also called neural network-based, or text generation-based, models. Presumably, Cayley would feel comfortable with either of these labels, though he clarifies that the most common label for this class of AI, ‘large language model,’ is actually a misrepresentation. One of the central themes of his essay is that large language models (LLMs) are ignorant of “human linguistic practice”, by which one is meant to think of pragmatics: “what they do not have is data pertaining to the human embodiment of language. . . The LLMs are working with text not language.” Large language models are, in fact, large text models. If your concern is with the nature of text-generation based models as writing machines, with a decades-long history of digital-technological experimentation and achievement preceding it; or if you are interested in the poststructuralist-theoretical context of text generation-based model development, then Cayley’s observation, or his contention, is not necessarily constraining. The fact that GPTs have no data on human embodiment would be largely irrelevant. It is indeed fascinating to think about how GPT training and development reflects aspects of poststructuralist thought, regarding, for example, the interplay of signifiers, context-dependent meaning, indeterminacy of meaning, and absence of authorial intent. Cayley alludes to this in his reference to Derrida, and to what he calls “the poststructuralist rub”: “Linguistics did, and still does, transcribe…differences, and then takes them in empirical evidence, as linguistic data. As Derrida pointed out, structural analyses could thus only ever be referred to (an archi-)writing, that is to what we perceive and then read, in the world as, precisely, text.” But text, as Cayley continues, “proves itself to be ‘not all’… not all of whatever language is.” This is the rub. Textuality does not pertain, or pertain enough, to the literary because literature is a language art, and language is orthogonal to AI, even the chattiest of AI.
“Language as such is always already embodied. If it is not embodied, it is not language,” Cayley insists, in connection to discussion about Derrida’s once famous, or infamous, dictum, “There is nothing outside the text.” First, I want to say I respect this claim, No language without embodiment. Second, I want to say I am wary of it, mainly for its intransigence. So many ideas and concepts are up for negotiation now. We ought to keep our minds open as much as possible, in part for the sake of keeping transdisciplinary lines of communication open as much as possible. There may be good reasons for wishing to consider non-embodied or differently embodied systems like transformer models as constituting language systems. What we do know now which we did not know before is the almost shocking extent to which artificial systems can successfully manipulate language (in the colloquial sense) in meaningful ways, and this means something important for philosophers, linguists, creative artists, and students of language arts just as much as for AI researchers and computer engineers. I think of Derrida’s Nothing outside the text. It sounds so definitive, like No language without embodiment; yet what was important in its time was precisely its equivocality. The striking formulation and the logic of it were both partly conditioned and enabled by the development and progress, and the sheer preponderance, of mass communication technologies in the twentieth century. “There is nothing outside the text” captured something of the weight of all the electronic-medial forms of encoding and inscription whose ubiquity helped to make up the character of Western industrial/post-industrial life, and that helped to make up what the philosopher Catherine Malabou refers to as the philosophical motor scheme of the twentieth century, that of writing.
Everything was, or became, a form of writing: television, cinema, baseball, dance, computer programming, DNA. Everything became a ‘text,’ and this outcome reflected a prevailing “material ‘atmosphere’ or Stimmung (‘humor,’ ‘affective tonality’)” (Malabou 14). It was the atmosphere of semiotics, a broadening of the concept of language that affected many disciplines in the humanities, and also existed in a synergistic relation with the AI research of the day. Today, the language we use to talk about language, text, and technology is being rocked by new developments. If we may have considered twentieth-century mass media technologies as impinging on life, we refer to our latest communication technologies as forms of life, even though we may also recognize the inappropriateness of doing so. In her book Plasticity at the Dusk of Writing, Malabou claims that the epoch of writing is over: “plasticity, as a still uncertain, tremulous star, begins to appear at the dusk of written form” (15). Whatever Malabou may have meant by this, nearly twenty years after the initial French language publication of her book it is sensible to think of AI as being central to her theorization of plasticity. What comes after writing will involve – and does already involve – the reconceptualization of writing, and perhaps of language too. What Cayley interprets as the mis-identification of transformer models as large language models may be a nascent part of this reconceptualization. AI of the GPT sort is poised at the cutting edge of such possible transformation.
Cayley’s strong commitment to the bond between language and embodiment is pre-emptive: it is designed partly to contain the spread of the transformer models’ cultural influence. So long as the meaning of language is delimited in the manner it is here, then the researchers and engineers who developed transformer technology are very possibly disqualified as viable interlocutors with language theorists like Cayley. Fair enough, one might claim. Cayley’s essay is about what constitutes literature and the literary. It’s his field. How often do computer engineers consult with literature professors regarding their business? But Cayley is writing about ChatGPT and literature, and it is a strategic oversight when he excludes the engineering-computationalist perspective from his introductory framing: “When considering the question of what literature is, pragmatically, I assume that there are two constituencies which particularly concern us: students (broadly conceived to include ‘scholars’) and practitioners.” In this case, I believe that the tech creators’ make up a third constituency which particularly concerns us, given how their labor has already proven so disruptive to the definition and conceptualization of the literary.
Cayley makes an argument for not thinking that transformer models should disrupt our ideas about language, and my addendum is that therefore we should not fear them as much as he seems to for how they might debase literature. Such fears are magnified through their insinuation in Cayley’s essay with bigger, more “existential risks of artificial super-‘intelligence’…” The following passage features the collapse of aesthetic and existential registers onto one another, in such a way that the intrinsic danger of transformer technology is amplified. Cayley explains that transformer models do not include data on the sequences of acoustic images, or sound images, that human readers experience in the process of reading, and that help to correlate text to aspects of the physical world for the human reader. But they could!
A robotic, humanoid version of…[such data] is, in principle, available to them as text-to-speech. . . . If such data is ever enfolded into the models’ vector spaces, I will be initially surprised, and then even more concerned about the future of literature, depending on who advises the use of data like this, from aurality, in the LLMs’ discourse. I devoutly, if somewhat despairingly, hope it will be humanists who do so because, as opposed to the default position of current engineers, they may agree with me that style and (authorial) voice are, amongst other features, ontologically essential to whatever language is.
Cayley has decided already that he will despair, because it won’t be the humanists doing the training. It will be the engineers, some of whom, of course, may consider themselves humanists too, yet may not share Cayley’s authorial vision of language. Likewise, they might not be troubled that their remarkable engineering feats have failed to capture the ontological essence of language. I don’t know if I consider myself a humanist, but I know I am not an engineer. And I feel some empathy for engineers, given the benighted way in which Cayley represents them here. The problem concerns the janus-faced invocation of the humanist, who may have his heart in the right place, but also is quite hung up on authority, and assuming the mantle of the true champion of style.
Sticking with the engineers for another moment, I will point out that the hermeticism of the transformer models worries some of them too. Just like Cayley and many others, they would like to understand how GPTs decide what they will communicate, or what the next word in the sentence will be. They would like to know how the complex, high-dimensional relationships between inputs and outputs that transformers learn are captured in the model’s parameters. However, unlike most people who would like to know, or who would at least like to know that it is knowable, the engineers have a shot at being able to reverse-engineer transformer models to find out. The Mechanistic Interpretability community believes it can do this. Chris Olah writes: “with sufficient effort, statements about model behaviour could be broken down into statements about circuits. If so, perhaps circuits could act as a kind of epistemic foundation for interpretability.” I am willing to believe that engineers offer the best hope for understanding the decision-making processes of this form of AI. It is highly unlikely that literature professors will come to the rescue.
Meanwhile, literary artists have already begun probing the creative possibilities of collaboration with transformer models and other forms of AI. Since Cayley discusses at the very start of his essay Scott Rettberg’s intriguing claim that GPT-assisted image creation is a form of e-literature, I would have thought he might have had more to say about how writers are seeking to use ChatGPT for creative ends. His position may have precluded him from saying anything positive about it, and there is probably very little to say positively yet. One of the highest profile experiments thus far is Aidan Marchine/Stephen Marche’s Death of an Author, recently published by the podcast production company Pushkin Industries. By Marche’s account in his Afterword to the novella, his murder mystery is 95% AI-generated, though he decidedly does not report it was therefore easy to craft. He describes his collaboration with AI as a “dialogic process.” Reviews were mixed, and my own opinion is that Death of an Author is both an enjoyable and sometimes annoying read. It is in every way a credible effort at machine/human collaboration for the sake of literary experiment, and for the sake of trying to accomplish something quite remarkable. There is reason to believe that still better things will come.
References:
Malabou, Catherine. Plasticity at the Dusk of Writing: Dialectic, Destruction, Deconstruction. transl. Carolyn Shread. Columbia University Press, 2010.
Marche, Stephen. Afterword, Death of an Author. Pushkin Industries, 2023.
Marchine, Aidan. Death of an Author: A Novella. Pushkin Industries, 2023.
Olah, Chris. “Interpretability Dreams.” Transformer Circuits Thread, May 24, 2023, https://transformer-circuits.pub/2023/interpretability-dreams/index.html.