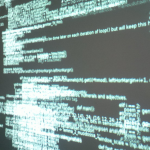
The authors speculate why some are bored by the goal of computational generation of "human-like" text. Inspired by Italo Calvino's alternative, minor strain in "Cybernetics and Ghosts," they argue that this kind of text generation provides an opportunity to destabilize as well as refine our sense of the differences between human and machine cognition.
“The Most Boring Thing You Can Do With a Computer”
Turing’s (1950) “imitation game” proposed the task of human-emulation as a standard by which we might judge if a computational system has achieved “intelligence” – or at least the kind of intelligence whose presence could be evinced by natural language conversation. As notions of intelligence have broadened, other kinds of “Turing Tests” have emerged, including some related to the production of art.
Generating convincingly human-like poetry or prose, for instance, has been posited as a Turing-esque goal - a task that could be considered both an engineering challenge and an artform. Within the electronic literature community, however, the goal of human imitation is often considered neither interesting nor relevant.
In an interview with Vice (Fernandez, 2017), computational poet Allison Parrish (creator of @everyword, Articulations, and other works) was asked if her goal is to create generative works that are indistinguishable from human ones. Parrish answered:
I think that imitation is the most boring thing you can do with a computer. It is frustrating because a lot of the academic research on creativity in artificial intelligence right now is focused on how to make a computer do something that an artist normally does, to take jobs that previously required skilled knowledge or creativity and trying to do them through a machine instead. They want to throw art and poetry into the mix and I don’t think it belongs in that same category. (Emphasis ours)
Parrish echoes the familiar “robots taking our jobs”-anxiety and observes that algorithmic imitation necessarily participates in an economic logic of automation. Yet, simultaneously, Parrish seems to set apart “art and poetry” as outside the category of tasks that should or even could be automated. Her critique is an aesthetic one: literary-computational work that mimics human forms is “boring.” Perhaps, but why?
Nick Montfort (2018) has observed that human-imitative text generation systems often rely on “cliché” rather than on producing linguistic innovation. In fact, this negative assessment is shared by some computer scientists who have taken the Turing Test to task for encouraging not true creativity but superficial “trickery” that merely produces facsimiles of recognizable styles (Pease and Colton, 2011).
But the literary argument against creative Turing Tests runs deeper, touching upon the ways that literary artists working with algorithmic media have conceptualized their goals. Clement Greenberg (1982) famously asserted that the modernist painting is about its own flatness. Analogously, Christopher Funkhouser suggests in Prehistoric Digital Poetry (2007), poets working with code have long sought to create texts that “make their essence apparent,” (3) that make legible and unmistakable their algorithmic bones. A poem that passes a poetic Turing Test will have instead cleverly obscured its digital nature. Moreover, such tests often take as their standard the well-worn forms of literary inheritance – sonnets, haikus, etc. (This includes, we should note, the Neukom Institute’s “Turing Tests in the Creative Arts”; Rockmore founded this contest and Booten is a former competitor.1For more information about the contest’s rules and results, see: http://bregman.dartmouth.edu/turingtests/.) To add to Funkhouser’s observation about the modernist impulse of digital poetry: if modernism was, following Pound’s famous pronouncement, a matter of “making it new,” a creative Turing Test could be thought of (less-than-charitably) as an opportunity to make it old, but in a new way.
Finally, if the creative Turing Test lends itself to aesthetic boringness (old forms, clichéd language), perhaps there is also something politically boring about it. Works of computer-generated literature, like media art more generally, often perform a self-reflexive critique. For instance, Annie Dorsen’s Hello Hi There (2010) stages a dramatic dialogue between two chatbots (based loosely on the famous Chomsky/Foucault debate of 1971). Far from producing a plausible substitute for these philosophers’ discourse, the two bots take turns lobbing stilted non-sequiturs back and forth. The failure of these bots to mimic human speech is in fact the point; for Dorson, their crooked, creaky dialogue is a way of evoking the “repeated failure” of early computer scientists who naively thought they would soon “crack the code of human language production” (Dorsen). Insofar as computational literary work aims to critique its very medium, it seems that it must at the very least draw attention to the characteristics of this medium and perhaps – like Hello Hi There – do so in a way that emphasizes its flaws and representational limits.
Calvino’s Profanation
Given the disdain that many feel for the Turing Test, it may seem surprising that early theorists of computational art, and more specifically computational poetry, did not share this dim view of human-imitation as a goal for artistic algorithms. In fact, they seem to have found this possibility fascinating. We now turn briefly to one of these theorists2Vilém Flusser’s (2011) speculations about computer poetry, for similar reasons as those discussed in reference to Calvino, also evince a fascination with human-emulation.– Italo Calvino.
In "Cybernetics and Ghosts" (1982), originally delivered as a lecture in the 1967, Calvino notes with some admiration that scholars of literature, from the Russian Formalists (e.g. Vladimir Propp) to contemporary structuralist semioticians (e.g. Roland Barthes) had taken great strides in describing all sorts of textual phenomena, from folktales to advertisements, in terms of their constituent units and the functions by which these units could be assembled to produce a valid text. Summarizing the underlying ontological claim of such research, Calvino argues that “[t]he world in its various aspects is increasingly looked upon as discrete rather than continuous” (8). It is crucial to keep track of what he is not saying: that continuous or fluid reality (the evolutions of cultures and practices over time, or human thought itself) can be modeled by computers. This would be a more modest claim. Instead, it is reality tout court, which may have seemed to be fluid, that has been revealed as “discrete.” Thought itself, Calvino argues, is “a series of discontinuous states, of combinations of impulses acting on a finite (though enormous) number of sensory organs” (8). It is not an accident that this reference to “states” and “combinations” sounds like the vocabulary of computation. Calvino glowingly cites the early luminaries of information theory and computer science – including Turing – for having replaced “shadowy landscapes of the soul” with an image of the mind as a machine whose workings are, in theory, formally describable.
Having allied himself with those who see reality as “discrete,” Calvino then turns to the possibility of computers that can produce (human-like) literature. Calvino himself seems to recognize the specter of “boringness” in creating such a machine; he is quick to dismiss what would be “‘assembly line’ literary production” – a term he does not explain, though perhaps we can read in it some worry about the dependency on simplicity and deadening cliché that have indeed characterized much human-imitative literary text generation. No, he refers to a more complicated version of such a poetry generator:
I am thinking of a writing machine that would bring to the page all those things that we are accustomed to consider as the most jealously guarded attributes of our psychological life, our daily experience, our unpredictable changes of mood and inner elations, despairs and moments of illumination. What are these if not so many linguistic “fields,” for which we might well succeed in establishing the vocabulary, grammar, syntax, and properties of permutation? (12)
Clearly Calvino’s understanding of the imitative power of computational technology is even more ambitious than the one posed implicitly by the (poetic) Turing Test. In this passage, we may just as well substitute for the phrase “writing machine” a shorter one: “a mind” or even “a soul.” What is at stake for Calvino is not that computers could trick us, could feign sorrow or joy; rather, any such poetry generation machine would serve as evidence that these “jealously guarded” attributes themselves are (just likely literally all other phenomena, from metabolic processes to birdsong) “discrete” rather than “continuous” and therefore available to analysis and synthesis. Imitating (implicitly lyric) poetry, a genre designed to render legible private feeling, seems to be a particularly tantalizing test-case. Where others may argue that the ability to produce convincing verse via algorithm is an example of the power of computation, for Calvino it would reveal the computability of those aspects of ourselves that we likely most strongly believe – and perhaps need to believe – are beyond computation.
Calvino senses that his avowedly “provocative and even profane” (17) argument no doubt does violence to the presuppositions of his readers/listeners: “[s]ome of you may wonder,” he remarks, “why I so gaily announce prospects that in most men of letters arouse tearful laments punctuated by cries of execration” (14). Without suggesting that Calvino’s attack on the ineffability of inspired Romantic authorship is in bad faith, we note the almost sadistic glee he seems to take in this argument’s violence. Seen in this light, a Turing Test – especially a “creative” one that takes as its task the production of poetry – has at least the potential to cause this same kind of shock. With reference to Bloom’s (1997) concept of the “Anxiety of Influence,” we might call this fear of being far too easily counterfeited by algorithms an “Anxiety of Imitation.” For Calvino, provoking anxiety seems to be the most interesting thing one can do with a computer.
But here the question of this discussion must turn on its heels: why hasn’t the fact of computer-generated, human-imitative literature caused this sort of shock, this visceral, negative reaction, rather than a yawn?
One obvious answer to the curious boringness of human-imitative algorithms is that our algorithms are simply not (yet) robust or sophisticated enough to threaten those “jealously guarded attributes of our psychological life.” If this is indeed generally true, it is no surprise that the resulting poems do not stir feelings of jealousy, let alone anxiety about the specialness of one’s own humanity. Yet to say that one human-imitative algorithm or another has not reached the (impossibly?) high standard of Calvino is not to say that the task of imitation itself is inherently boring.
Another answer is that Calvino seeks to provoke an Anxiety of Imitation to which contemporary computational poets by and large are not vulnerable. After all, his critique truly takes aim at the egos of the non-digital poets, those who actually write or type, word by word, from their own minds; compared to them, computational poets such as Allison Parrish who compose verse-generating algorithms remain insulated from Calvino’s attack on the author simply because they have already ceded this position of authorship. Instead, they are discursively and structurally analogous to the computer scientist who might program an algorithm to emulate the writings of Dickinson or Shakespeare – both meta-poets or, to borrow a phrase from computational poet Ross Goodwin, “writers of writers” (Merchant, 2018).
Between Boredom and Shock
So far this discussion has considered why a creative Turing Test may be inherently boring as well as why, under certain conditions, it may become all too interesting. Yet why does the discussion of Turing Tests and human-like text generation seem to sprint toward the extremes, describing the project as either not worth doing or as having the potential to reveal human thought and feeling as finally unmysterious? To conclude, we offer our own perspective on the value of the creative Turing Test, one that we hope will be of interest even to those who find the very notion of this activity to be uninspiring and Calvino’s pronouncements unrealistic.
As Jennifer Rhee (2010) has argued, there is power in the fact that the Turing Test is premised on “misidentification” – the mistaking of human for non-human, or vice versa – since these errors lead us to sense “how fluid the category of the human is, and how resistant it is to efforts to render it as stable.” With this sense of “fluidity” in mind, we might reject Calvino’s almost total conflation of human and machine intelligence and instead, in more subtle ways, use creative Turing Tests as an opportunity to confront our own definitions of human thought in order to see – to test – where they may indeed overlap with and diverge from machine cognition.
Take, for example, a sonnet generation algorithm that won the 2016 “Turing Tests in the Creative Arts” held by the Neukom Institute for Computational Science at Dartmouth College. This system, Hafez (Ghazvininejad et al., 2016), cleverly combines several different approaches to produce sonnets that are meant to pass as human. Its poem titled “Bipolar Disorder” begins like this:
Existence enters your entire nation.
A twisted mind reveals becoming manic,
An endless modern ending medication,
Another rotten soul becomes dynamic
We offer some basic observations about this stanza: that the lines rhyme and are metrically-sound iambic pentameter, that there are words that obviously relate to the subject (“manic” and “medication”) as well as those that do not (“nation”), and that the line generally seems more or less syntactically “correct” (though not necessarily fluent).
To truly read this sonnet, however, we must turn to the authors’ explanation of the Hafez system. The system’s strategy can be summarized as follows:
- Given a topic word, the program finds words that are semantically related. From a list of such words, it extracts pairs of words that rhyme. These words are placed into the poem according to the rhyme scheme of the sonnet (abab, cdcd, etc.).
- Having filled in the final word in each line, the program then begins to fill in the rest of the poem with words from its vast vocabulary. For each line, it generates all possible sequences of words that 1) end with the already-chosen rhyming word and 2) are valid iambic pentameter. Crucially, most of the resulting poems are entirely nonsensical. For instance, the authors give the example of the line “Of pocket solace ammunition grammar.” – a metrically-valid (but syntactically- and semantically-irregular) line that rhymes with another line ending with the word “banner.”
- Using a neural network trained on song lyrics, the system surfaces out of the huge number of mostly-nonsensical sonnets those poems that seem least nonsensical, most statistically probable (i.e. that most closely resemble the song lyrics upon which the neural network was trained).
Does Hafez’s algorithm make poetry seem like a “solved problem”? No, its cleverness is unlikely to lead human poets to hang up their laurels in resignation. Yet this does not mean that Hafez is boring, having failed to capture the mystery of poetic thought. Between boredom and shock, Hafez offers something more modest: an opportunity to take stock of exactly which parts of the human process of writing verse are most susceptible to algorithmic description. Anyone who has written in a formal verse form may find something familiar in the way that Hafez writes “backwards,” first picking out a rhyming word to end a line before trying to fill in the front of the line in a way that makes sense. With a topic in mind, a poet may likewise begin by choosing rhyming words that are related to it, guaranteeing some measure of coherence. Is it possible to specify something about how a human writer might do this in a way that is yet more complex than the way that Hafez does? If not, how unsettling is this realization? How “jealously guarded” is the humanness of this particular aspect of the craft of poetry?
At other moments, the differences between human and algorithmic writing seem stark. The human poet may feel the urge to scratch out a syntactically vague line like “An endless modern ending medication” (Is “modern” here a noun or an adjective?) or the similarly difficult-to-parse line “A twisted mind reveals becoming manic.” Frustrated or stumped, the poet could be tempted to start the poem over with another set of rhyming words. The poet may even from time to time violate in some small way the rules of a verse form when such constraints become stumbling blocks. Hafez makes no such adjustments. It simply cannot stray from its strict pentameter and its chosen rhyming words. Where a human poet would yield to normative syntax, Hafez merely does its best to produce syntactically-normal lines despite its own fanatical metricality. Depending upon one’s proclivities, this may either be the system’s folly or its source of aesthetic verve; its ponderously perfect pentameters are artifacts of a possible rift between the respective ways that humans and computers tend to produce language. Yet there is nothing inherently computational in Hafez’s strategy. A human poet might actually try to write “after Hafez,” intentionally composing sonnets that are metrically immaculate but syntactically wobbly. If algorithms can mimic our minds, certainly we can mimic theirs. Perhaps there is something to be learned, or at least expressed, through such emulation.
Our point is merely that Hafez’s algorithm and its poetry are worth considering as works not just of engineering but also of literary art. This is not because Hafez leaves us convinced that we are ourselves nothing but bits all the way down, or even that the human act of authoring of poetry is less impressive than we might have thought. Instead, programs such as Hafez are models of minds; they may do things that remind us of how our minds use language, and they may do things that seem utterly alien, mechanical. Beyond the failure or success of an “imitation game,” these models – however imperfect they may be – invite us into a recursive and “unstable” consideration of the ways that our most “jealously guarded psychological attributes” do or do not (and should or should not, could or could not) carry the echo of an algorithm.
Works Cited
Bloom, Harold. The Anxiety of Influence: A Theory of Poetry. Oxford, UK: Oxford University Press, 1997.
Calvino, Italo. “Cybernetics and Ghosts.” The Uses of Literature. Translated by Patrick Creagh, New York, NY: Harcourt Brace, 1982, pp. 3-27.
Dorsen, Annie. Hello Hi There. Performance recording credited to Ulrich A. Reiterer, Stephan Bergmann, Jona Hoier, and Julian Stampfer, 2010, https://www.youtube.com/watch?v=3PiwEQQNnBk.
Dorsen, Annie. “Hello Hi There.” Documentation on author’s website, http://www.anniedorsen.com/showproject.php?id=6.
Fernandez, Mariana. “What it Means to be an ‘Experimental Computer Poet.’” Vice, 12 October 2017, https://www.vice.com/en_us/article/8x8ppp/poetry-twitter-bots-best-twitter-bots-art-allison-parrish-everyword.
Flusser, Vilém. “Poetry.” Does Writing Have a Future?, translated by Nancy Ann Roth, Minneapolis: University of Minnesota Press, 2011, pp. 71-78.
Funkhouser, Christopher Thompson. Prehistoric Digital Poetry: an Archaeology of Forms, 1959-1995. Tuscaloosa, AL: The University of Alabama Press, 2007.
Ghazvininejad, Marjan, et al. “Generating Topical Poetry.” Proceedings of the 2016 Conference on Empirical Methods in Natural Language Processing, edited by Jian Su et al., SIGDAT, 2016, pp. 1183–1191.
Greenberg, Clement. “Modernist Painting.” Modern Art and Modernism: A Critical Anthology, edited by Francis Fascina and Charles Harrison, London, UK: Sage, 1982, pp. 5-10.
Merchant, Brian. “When an AI Goes Full Jack Kerouac: A Computer has Written a ‘Novel’ Narrating its Own Cross-Country Road Trip.” 1 October 2018, https://www.theatlantic.com/technology/archive/2018/10/automated-on-the-road/571345/.
Montfort, Nick. “Conceptual Computing and Digital Writing.” Postscript: Writing After Conceptual Art, edited by Andrea Anderson, Toronto: University of Toronto Press, 2018, pp. 197-210.
Pease, Alison, and Simon Colton. “On Impact and Evaluation in Computational Creativity: A Discussion of the Turing Test and an Alternative Proposal.” Proceedings of the AISB symposium on AI and Philosophy. AISB, 2011.
Rhee, Jennifer. “Misidentification's Promise: the Turing Test in Weizenbaum, Powers, and Short.” Postmodern Culture 20.3 (2010). Project Muse, doi:10.1353/pmc.2010.0015.
Turing, Alan Mathison. “Computing Machinery and Intelligence.” Mind: A Quarterly Review of Psychology and Philosophy 59.236 (1950).