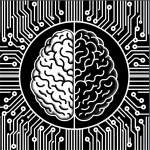
Drawing on the media theory of Bernard Stiegler, this riPOSTe to recent ebr essays by Heckman, Cayley and Pold considers the implications of automation for knowledge - both its loss among humans, and its acquistion by AI.
Recent essays in EBR and elsewhere dwell on the question of AI’s meaning for us humans, be it agency, participation, language, or literature. In this essay I wish to add an element to the discussion that I have not seen raised. This is the element of knowledge: How it relates to information and language, and how it may be lost with automation. Furthermore, can AI have knowledge, does it know what it is doing? Finally, I discuss the danger of surrendering our own knowledge to AI.
Introduction: The Possibility of Negative Critique of Technology
Discussing large language models and their projected influence in “Thoughts on the Textpocalypse”, Davin Heckman submits that “[b]eyond upended careers and creepy dystopian resonances, they exploit the dynamics of social trust that make life livable and instrumentalize language as an industrial tool for social engineering.” In my view, this is all true. However, Heckman adds, scholars working on new media (in the U.S.) rarely raise strong criticism about digital media. Then, the possibility of negative critique of digital media, including AI, hitherto lacking, was opened by Matthew Kirschenbaum in the essay “Prepare for the Textpocalypse”, which Heckman takes as a springboard in his own essay.
There is indeed a kind of normativity surrounding the discussion of AI, a normativity through which the potentially negative critique has to push. Let me illustrate these “technocapitalist tropes” (as Heckman puts it in his Re-riPOSTe to his own essay) with a recent keynote lecture by an AI consultant. The keynote was given in the Finnish Teosto Talks -lecture series in Helsinki.1Teosto is a copyright organisation for “music creators, composers and publishers”, and this talk was organized around the question of how AI may impact music creation and production. “Those who don’t adapt, die”, said the speaker, Valerio Velardo, after introducing himself. He explained the maxim by relaying the stories of Nokia (losing its major market share unable to adapt to the new smart phone market after the iPhone in 2009) and Kodak (failing to adapt to the arrival of digital cameras in the late 1990’s). The reasoning goes like this: AI cannot be stopped, so we must embrace it. And for those who embrace it there is a kind of gold rush; they may become “winners.” Make no mistake, there will also be losers, and the losers will be those who do not adapt. “Don’t be Nokia!” he concluded his keynote to a mainly Finnish audience.
I am offering this as an example of the normative push whose effect is to prevent critique. The one to raise doubts has to deal with the possibility of being one of those unadapting losers marching towards imminent death. The speaker was, of course, or probably, speaking figuratively about dying. It seemed, however, significant that the word had stopped (maybe?) meaning actual death and it now designated business failure, or perhaps just strategic human failure.
Beyond the inevitability of technological advance and the necessity of adapting, Heckman points out that in the critique of new media, new technology is often placed in a continuum with old inventions, and since our consensus seems to be that older inventions turned out to be harmless, we must see how silly it is to criticize new ones. Let us not be those who always worry about new things, goes the credo. The keynote speaker’s example was his own favorite conductor of orchestra, Sergiu Celibidache (1912-1996), who, as he told us, opined that people should only listen to music that is played in the same space with the listener. Celibidache, therefore, was adamantly against recording music. Bernard Stiegler, to whose ideas we will get in a minute, mentions that in the 1930’s the composer Bela Bartok pioneered the use of recording with the phonograph for music and understood its revolutionizing effect, but still thought that nobody should listen to radio unless they are reading the score at the same time (Symbolic Misery Volume 2: The katastrophē of the sensible, 15).
Finally, Plato and Socrates are often brought in to remind us doubters of technology that these otherwise remarkable philosophers were worried about the technology of writing. In Phaedrus, Socrates recounts the words of the Egyptian king Thamus to whom the god Theuth is presenting his arts, including writing. Theuth says that he has discovered a potion for “memory and wisdom” in writing. Thamus retorts:
“O most expert Theuth, one man can give birth to the elements of an art, but only another can judge how they can benefit or harm those who will use them. And now, since you are the father of writing, your affection for it has made you describe its effects as the opposite of what they really are. In fact, it will introduce forgetfulness into the soul of those who learn it: they will not practice using their memory because they will put their trust in writing, which is external and depends on signs that belong to others, instead of trying to remember from the inside, completely on their own.” (Plato 274E-275A)
In the dialogue, Socrates makes Phaedrus admit that Thamus is correct about this assessment. What does the zeitgeist say as expressed in the words of Velardo on Celibidache? “How silly that sounds today.” He did pause here for a moment, then continued to make sure: “Right?” It should be clear to everyone that it was not reasonable to worry about recording music, the radio, or writing. Because they are fine, and we are fine. Nothing bad happened, after all. Right?
But why should we be so sure that the worry was misplaced? To my knowledge there is no definite way of assessing whether our memory and ability to think, or our ability to listen to and appreciate music, have improved with the advance of technology. Although there are undeniable advantages to these technologies, it is not to say something wasn’t lost in the process. There is another implied claim nested in the example: that all technological developments are of a similar kind; that if someone at some point worried about music recording, and it was misplaced, it must also be misplaced to worry about other technological inventions such as AI. However, and this is my point: we will not know whether it is so unless we assess it critically. Therefore, to critique new technologies is not misplaced and automatically reactionary (another normatively used concept), but necessary. I thank Davin Heckman for raising this last point in his essay. From here, I wish to bifurcate in the direction of the loss of knowledge.
For sure, technology is neither good nor bad, but a pharmakon, by which I mean, following Bernard Stiegler (and Plato as well as Derrida), that it can be both poison and remedy (Heckman uses the other meaning of the pharmakos as scapegoat, which is also useful and opens up a whole new path beyond this essay). For Stiegler, with grammatization, which is something the above examples (recording, writing) share, as the spatialisation of temporal flows, there is always a loss of knowledge. That there is a loss doesn’t mean that all knowledge should be preserved, but unless the time and energy saved with new technology is “reinvested” in what Stiegler calls dis-automatisations and noodiversity, that is, new knowledges, then it is indeed just a loss and will lead to proletarianization. These three: grammatization, loss of knowledge, and proletarianization are keywords in what follows.
But, before we move further with Bernard Stiegler’s ideas, I feel obliged to address Søren Bro Pold’s questions, presented in his riPOSTe “Textpocalypse Now?” to Heckman, on whether we should use Stiegler’s ideas for a “new left-wing critique.” Pold fears that finding the seed for a new critique in the philosophy of “the old bank robber” might mean “joining the reactionary.” He asks whether Stiegler “really understood” social media or digital subjects. No explicit argument is provided to supplement the questions, and we are left with a reference to Stiegler’s own work (Technics and Time 3), and to Pold and Andersen’s work. In Andersen and Pold (2018, 74-80), Stiegler’s critique of Adorno and Horkheimer is presented, as far as I can see, as a useful critique pointing us to a more subtle understanding of technology and the culture industry. As for social media and digital subjects, I am not sure what Pold means by “really” understanding them. Is there a real way of understanding digital subjects and social media?
I think Pold’s questions require elaboration to be adequately assessed, but based on my own reading of Stiegler, I would say that Stiegler definitely understood social media and the digital subject, in his own way, as one who had studied the field of digital media since the 1990’s. In my view, more importantly, Stiegler’s thinking is highly useful in furthering our understanding of technology and media in a broader historical and societal scale, and I see no reason whatsoever why it would risk us joining the reactionary. And, beyond Stiegler, maybe that risk should anyway be taken in order to critically assess new technologies? As I argue above, not all negative critique is reactionary, and not all new inventions are purely good (or bad). As for specifically “left-wing” critique, I am not sure why critique of technology or new media should be assigned to either or any wing at the start. And, finally, if I may comment on the description of “the old bank robber” – yes, Stiegler was incarcerated for armed robbery, as is well known from his own books and elsewhere. But incarceration, for Stiegler, was the beginning of philosophy. Therefore, I think an old bank robber, convicted, incarcerated, and reborn as philosopher, working for decades on the questions of memory, technology and its effects, launching communal projects, founding collectives, writing books and articles, addressing contemporary issues (see e.g. the immense effort in Bifurcate by the Internation Collective co-led by Stiegler) – will do fine. Definitely, Stiegler will do more than fine as one of the noteworthy thinkers we can think through and with. But maybe I misunderstood, and perhaps Pold will elaborate on his views on Stiegler. Moreover, I hope that the present riPOSTe essay, studying the question of knowledge through Stiegler, answers in its own way to another of Pold’s questions: “How do we understand the many ways tertiary retention grammatizes us?”
Loss of Knowledge
As mentioned, the contribution I would like to make to the recent conversation is the aspect of loss of knowledge through automation, with specific reference to AI. Heckman does bring loss of knowledge up as the replacing of the knowledge of how to live with the knowledge of how to do, evoking contemporary proletarianization. Stiegler, however, typically speaks of the knowledges together — as knowledge of how to live (savoir-vivre) and knowledge of how to do (savoir-faire), and also of the knowledge of how to think or conceptualize (savoir conceptualiser) (e.g. States of Shock, 15). For Stiegler, knowledge is a broad concept.
In his essay, “Modelit: eliterature à la (language) mode(l)”, John Cayley points out the difference between text and language: text is linguistic signs presented on a medium, but language is an embodied practice with its “evocalizations” (a term borrowed from Garrett Stewart). Therefore, language cannot be reduced to text, and the new large language models (LLM’s) are better thought of as large text models. I find Cayley’s distinction important. It is also fruitful for thinking about the parallel binary involving information and knowledge. Text is not the same as language, but neither is information the same as knowledge. Information is signs on media, but knowledge is an embodied process in time. And, as we don’t only have bodies, but also minds, knowledge is both embodied and minded. Information is stored on an external physical medium such as a magnetic disk and processed with programs, but knowledge is something that passes through understanding, and is not stored but retained, and is not external, but internal.2For the purposes of this essay, we can leave the definition of mind open. It is enough to say that there is a mind. Knowledge is not static, but a process of retaining what is known, always in relation to other things, and a process of learning new things. Retention implies re-tension, and this tension is, I think, important. Unlike a computer, which recalls whatever it has stored as identical to the thing stored, when a bodyminded creature, e.g. human, retains and remembers, it may put the issue in tension with other things that are going on in their embodied minds, in a specific time and place. Similarly, when encountering something new or creating something new, the ability to hold this tension open, to examine it and to deliberate within it, is crucial. To know how to write, for example, requires the ability to hold the tension between the past and the future of the text in the present moment, right here, and the ability to think about and feel for what comes next. Noncomputers in a time and a place with specific histories and affordances will remember a thing and understand a thing in a singular way, peculiar to that situation (which doesn’t mean it cannot be shared).
In Stiegler’s terms, drawing from the phenomenology of Edmund Husserl, there is primary retention, designating the present moment (including the “just past” of, say, a melody), and secondary retention, when the primary retentions are retained as what we call memories. Stiegler’s own addition is tertiary retention, which Heckman parses as “recorded, archived, and recirculated memory” – potentially all technology. Coming back to the technology of recording, we can make the note that every time we hear the same recorded melody, we experience it differently. The tension is renewed. The difference, according to Stiegler, is due to accumulated secondary retentions. Therefore, secondary retentions condition primary retentions; past experience influences present experience. In other words, since we clearly can’t attend to the whole of reality at once, primary retentions are selections of what is available, and the selection criteria come from secondary retentions (Technics and Time, 3). This is a very rough picture, of course.
The crucial invention, according to Stiegler, of the culture and advertising industry in the 1900’s, hyperindustrialized by contemporary digital media, is that tertiary retentions can “gain a certain amount of control over the play” (Ross 18) between primary and secondary retentions. The digital interface providers, within the contemporary economy of attention, would hope, of course, to gain control over consumers’ retentions and protentions in order to make their desire calculable and therefore their future selections predictable. And, as long as users remain within the realm of short circuits and immediate affect, there is, as we witness everyday with social media, great predictability. Knowledge, however, is a long circuit, and, as it requires holding the abovementioned tension open for an extended moment, goes beyond immediacy and affect, and is, in fact, fundamentally noncalculable.3The question of the calculability of mind and its faculties, such as understanding and knowledge, touches upon the issue of the human-computer analogy, stemming from the history and present of cognitive and information sciences along with information technology (see e.g. Alombert 2022). Stepping outside this flawed analogy, it becomes clear that not everything, and specifically not knowledge and understanding, is calculable. The same point can be argued through reason as Stiegler does, but also formally (.e.g Penrose 1997; Bishop 2021). Knowledge as slower and less predictable is, evidently, one of the reasons why digital interfaces constantly prompt us to make our selections quickly and design their affordances so that we remain unthinkingly in the ever-renewing present moment of constant short input and (affective) reaction.
Therefore, to look at the automation of text (e.g. in LLM’s) without understanding that this is not language, as with Cayley’s view, is, in my example, to look at the automation of information without understanding that this is not knowledge. Or, to emphasize the processual nature of knowledge: that this is not knowing. To know is to understand and retain something, and to understand and retain it in relation to other things. To understand something new is to set it in relation, in tension, with other understood things. This tension and its temporary resolve as understanding is dependent on the particularly individuated and embodyminded (forgive the neologism) creature in a specific time and place. This is nowhere near to predicting the next viable token, which is what the LLM’s do. Another useful notion from Cayley is the orthotext, a cleaned up version of language, purified from the noise of, say, raw internet files as well as from embodied and situated practices of language. LLM’s textual processing refers to the orthotext whereas a situated, embodied knower can produce something new and singular.
I found an illustrative example of knowledge in Lulu Liu’s talk from the ELO2023 conference in Coimbra. Liu gave the example of an artist describing how they would create a piece in the style of another painter, say, Édouard Manet. For (an) AI, the process is analytical and statistical, fundamentally calculable, and the current AI’s perform this task well. They can imitate a Manet and create new paintings in the style of Manet, no problem. For a human artist, as Liu explained, the process involves searching their memory of Manet’s painting, perhaps looking at the paintings again, and understanding in their own (particularly individuated) way what they think may be Manet’s style. Then, taking something interesting from that mental image, the artist begins to make the painting. In other words, setting that retained understanding of Manet in a new tension with whatever is going on with the artist and their world at that moment. The task is not to make identical copies, but to create something new in the style of. So, the artist begins to make, and the ensuing process will almost necessarily yield something quite different to an imitation, as it involves a particular way of synthesizing, perhaps “wrongly” remembered traits from Manet’s painting, and also reflecting, correcting, and inventing during the process of working with the canvas, paint, and tools. The result might not even look like a Manet, but in the process something new has been created. (In the process of making and discovering as the setting of tension and its temporary resolve, I think, as a side note, also lies a big fraction of the pleasure of creating something, be it a painting, an essay, an article, or a poem.)
The above example, to me, illustrates the difference between information and knowledge plainly: information processing is determined and calculated and works within a finite, preframed set of parameters and focuses on the end result (“remarkable outcomes”, as Liu put it), whereas knowledge is indetermined and open to change and new input during the process. This is not to say knowledge is not conditioned and always potentially faulty, but it is different from calculation. Art is never finished, if we believe the worn-out quote by Leonardo da Vinci, and similarly knowledge is never complete; there is no final goal for knowing. Much like individuation, discussed by Heckman, knowing is an unending open process of tension and temporary (metastable) resolve. There are, of course, finished (or abandoned) works of art, and AI can certainly produce remarkable outcomes. But, especially in relation to AI, we put an outsized amount of focus on the appearance, behavior, and outcome of cultural artefacts, and too little attention on the interiority and process of making and attending to them, e.g. writing and reading.
We tend to define information as organized raw facts about the world, and to assume that these facts can be conveyed scientifically as such, whereas the “humanist” will then, as a bonus, ask about meaning with questions such as “Who am I? Why am I here? What is my relationship with others?” (Kennerly, Frederick & Abel 7). But, in this description we forget that information, in itself, is nothing. We have already conflated humans into computer-like information processors when we say that there are facts as such. In the re-definition that I defend here, information is organized data about the world, but to become anything, it has to enter the mind of a conscious creature, human or otherwise. To enter the mind of a conscious creature is not the same as “processing information”, although it is not necessarily yet knowledge either. In the case of information processing the raw data remains raw and is indeed processed according to preset programs. When human knowledge is transcribed onto a medium, for example as text, it becomes grammatized, and then the best descriptor is again information. To become knowledge to another human, the information has to pass through understanding, which may or may not happen in the bodymind of a conscious creature.4Consciousness is something that cognitive theories based on the analogy between the human and the computer as information processors tend to underestimate, as they assume consciousness arises from a particular kind of information processing (see. e.g. Hayles 2021). The fact is, however, that despite attempts, there is no consensus of an “information processing” explanation of consciousness. Instead, the hard problem of consciousness remains unsolved. I think the developments in AI will bring this problem into even starker light.
Let us say that there is a book, a long-forgotten codex, that reveals our future in relation to AI, thus proving or disproving our fears and fantasies about it. Let us say that this very book is on my shelf, here in my office. However, I have not read it. In this case, I have the information (as a freely accessible tertiary retention), but since I have not read it, it is as good as nothing to me. Let us now say that I read the book, every word entering into my mind, but I do not understand a thing; I understand the language but to me it is just nonsense. I still don’t have knowledge, because I have not understood anything. Let us then say that I memorize the words without understanding their meaning, and whenever there is a debate on AI, I chip in regurgitating the word sequences I have memorized from this book. I still don’t have knowledge, although I have the information. That I really don’t know what I am talking about will be revealed sooner or later in the conversations, when I apply some of the memorized material in a nonsensical matter. Let us then say I am ashamed by my blunder and I go back to the codex and I study it in detail, and now I begin to understand. I begin to see how things connect within the textual information, and I begin to see how the information connects with other things I know about the world. Now, when it comes time to apply what I have learned into a real-world case, I can do it based on understanding and I do not have to rely on memorized word sequences. Now I know what I am talking about. Of course, it still doesn’t mean that I am always right. As said, knowledge is always incomplete.
Processing, in the sense of information technology, is figurative when applied to humans, not literal. Understanding is not automatic, and it has no fixed goal. I will understand the same information differently than you, and our knowledges will also differ. This is the noodiversity I mention above, diversity of the ways to know, which have been under threat for some time with the homogenizing effect of always recallable but at the same time standardized information, that, without understanding, we will simply regurgitate out when necessary. With calculation comes automation and standardization, and to counter this, we need a diversity of knowledges.
So here comes the trouble: although knowledge cannot be automated with AI, its output and associated behavior can be modeled, and if we mistake these two for each other and begin to rely on the model, there is a danger of losing the knowledge the model was based on. In fact, the stakes with automation are very high, and mistaking the model for the thing itself leads to relying on the model more and more and in new spheres of life. Relying on the model as the thing itself more and more leads to general automation. In Stiegler’s view, general automation results in general loss of knowledge, that is, general proletarianization. General proletarianization results in, well, madness (Stiegler, Age of Disruption).
Automation Grammatization Digitalisation
To make better sense of automation we must think of grammatization. Grammatization, for Stiegler, is the conceptual step that enables modeling and automation. For EBR’s readers and scholars of digital media, grammatization makes instant sense, because the basic ideas of digitalisation and grammatization are the same: dividing a continuous entity into discrete parts and modeling its behavior by orchestrating those parts. Digital binary code is an instance of grammatization, but grammatization is not tied to the digital sphere and goes further back in history. As Daniel Ross summarizes, Stiegler
is able to push the origin of the grammatization process backwards in time to the ‘arche-cinematic’ reproductions of Upper Palaeolithic cave painting, and to extend this process forwards, not just to the grammatization of visual and auditory perception that occurred with radio and cinema, but, prior to that, to the grammatization of the manual gestures of the worker or the craftsman that are spatialized in being programmed into the machinery of the industrial revolution, and finally to what is unfolding right now: the grammatization of ‘everything’ […]. (20).
“Everything” means all digitized spheres of life, which, if not literally everything, is a lot. Stiegler reads the upper Palaeolithic cave paintings as portraying a deer in motion with successive images of the deer, like an early version of a sequence of individual moves. Jacquard’s loom (patented in 1804) is one example of the grammatization of gesture, in which the gestures of the weaver of the loom were divided into discrete moves, and the sequence of weaving inscribed onto punch cards and therefore automatized (Stiegler, For a New Political Economy 10). Notably, however, what is grammatized is not just gesture, but the retentional flow of the weaver, that is, the flow of consciousness in time is spatialized into instructions on punch cards.
Through examples such as these, Stiegler submits that the meaning of proletarianization is in fact the loss of knowledge through grammatization (Stiegler, For a New Political Economy 37-38). What is lost here? The weaver does not necessarily lose their job, but gradually the knowledge of how to produce the fabric, how the loom is operated, and how to create something new with it, all connected to a broader milieu of work. Instead, the machine progresses while the weaver, as producer, is excluded from participating “in the evolution of the conditions of production” and seizes to individuate through this work. Therefore,
“[p]roletarianization is a process of losing knowledge – that is, also a loss of savor and of existence – engendered by grammatization insofar as it short-circuits the processes of transindividuation through which, by becoming individuated through work, that is, through learning something, the worker individuates the milieu of their work” (ibid. 38).
In grammatization and its subsequent automation also standardization takes place. This is not necessarily the same but it is similar to Cayley’s orthotext. “What, of language, has been lost in the ‘clean up’ and by the ‘corrections’?” Cayley asks. Furthermore, Cayley continues a few lines later, we have taken for granted that language can be digitized in the first place.
In what is lost, I wish to highlight one feature, and it is the grammatization of the retentional and protential flow, which designates the inner view, the embodyminded (I apologize again for this neologism) aspect of the weaver. This brings out the crucial aspect that knowledge does not entail just behavior that we can assess from the outside, not just gestures, nor is it just the final output that we can assess in the end in its complete form. Knowledge is a process in time, and it happens within a being’s bodymind, i.e. consciousness.5This means that there cannot be knowledge without consciousness. Consciousness here is the so-called phenomenal consciousness, which includes states of not being metareflexively conscious (i.e. to be aware of having a conscious experience). AI’s such as LLM’s are not conscious. But without consciousness, AI cannot know a single thing. AI has access to information, and it has protocols as the spatialized representation and modeling of the behavior and appearance of knowledge. In other words, the computer has knowledge only in its grammatized form, as data, and it can “interpret” the data in a given context, which makes it information, but since knowledge happens in a bodymind with consciousness, AI doesn’t know anything. As an obvious consequence, large text models do not know what they are saying.
But perhaps not all loss of knowledge is for the worse? For example, the washing machine washes the laundry, and this too involves a loss of knowledge as I do not have any know-how about washing textiles. What chemicals go into the detergent, the amounts of it, water temperature, different gestures for different clothes, stains, soaking times, and so on. I am willing to give this knowledge up in order to do something else with the time and energy thus saved. I want to invest the time and energy saved into creating other kinds of knowledge and skill.
A presenter in the ELO conference, going by the name of The Digital Coy, floated the idea that everything that can be automatized, should be automatized — precisely because then we could direct our energy towards more interesting things, creative work, and so on. In other words, the familiar dream of automation. In my view (and beyond the unequal distribution of the benefits of automation) we need a better understanding and assessment of the loss involved in everything we assume can be automatized, because if we continue to mistake the appearance and behavior, i.e. the model, for the thing in itself, we will keep misunderstanding the reach of automation. And if we misunderstand the reach of automation, we will continue to try to automate what cannot be automated, for example, knowledge. The catch is this: knowledge cannot be automated, but knowledge can be lost. And it can be lost through automation.
Then, the question of whether everything should be automatized is a question of what we are willing to lose if that happens. If we automatize tasks whose aim is to better our understanding – say, education, research, reading and writing, we can still produce outcomes that imitate existing outputs, but after some time we may not understand them anymore. We will need another program to tell us whatever it is that it means. A student or a scholar who writes their paper relying solely on chat-GPT will literally not learn how to search for relevant information, how to understand that information for it to become integrated as knowledge, or how to think about the task given, and how to compose and write the paper. They will not be able to think. And, perhaps most dauntingly of all, they will not see why they should, because knowledge is connected to desire. These tasks can be automated, as we have already seen, but the loss involved is great.
The idea of general AI, viewed from this perspective, is the idea of general grammatization and subsequent automation. General automation leads to general loss of knowledge. We don’t even need the recent surge in popular AI’s to see how we might be heading to general loss of knowledge of how to live, how to do, and how to think. We already have programs that select our partners, tell us when to go to sleep, get up, how many steps to take, when to rest, what to read, watch, listen, eat, which clothes to buy, and so on. As for thinking, we don’t have to: plenty of software can reduce a text into its key points, do the same for video and audio, and then produce an article based on it.
To automatically summarize the key points of a text, I might learn something, but if I read the text myself, in the temporal retentional process of attending to that piece I may learn something completely different, and my understanding will be experiential — I might have an idea completely unrelated to the text. My body and mind flow through time while I read, and I set what I read into tension with other things I know. And maybe I have reflux; while I wait for it to pass, I look out of the window, and suddenly I realize something I have been latently thinking about for a long time. This leads me to create something unexpected.
I cannot successfully prompt AI to summarize the text as if I read it, and even this would not be enough, it would have to read it as my future self in a specific location with these and these pieces of lunch moving and being digested in my intestines. And still, I would not have learned anything in the process. But if, and here we can see the difference between knowledge and information, we think that all there is to it is information that can be extracted, and then placed from one memory to another, then yes, I might as well download the standard summary to my information processor that we call a brain.
If we treat a cultural artefact only as something from which information can be extracted, then we might as well bypass the whole artefact and simply distribute the information in the first place. Art and science streamlined into downloadable summaries. Cultural artefacts become ghosts that never were.
Conclusion: “Read Stiegler as if you were the Devil”
Looked at from this perspective we arrive at a pharmacological junction, the first road of which is that AI cannot replace knowledge. For example, writing; it cannot replace the knowledge of the writer as a non-calculable interior process, that has a history (through secondary and tertiary retentions), a present moment when it takes place (with its living contingencies), and a future as the writer’s desire to create something, i.e. protentions of what can be. AI has no history, since it has no temporal experience and instead of retention it has recall. Without temporal experience it is senseless to speak of a present moment either, even if from our perspective AI works in time. Based on data and programs, AI predicts the next token — whose veracity or usefulness is to be ultimately assessed by a knowing bodymind, since AI does not know what it is. AI has no desire, and indeed, for Stiegler, knowledge and desire are always connected. The other road of the pharmacological junction is that AI has the potential to displace knowledge, provided that we surrender our own knowing to its applications.
Allow me to end this riPOSTe essay with a final thought experiment. First, I asked chat-GPT3 to summarize Stiegler’s view of knowledge and got a Wikipedia-style generic summary, then I asked it to do the same “as if it were the devil.” Here is the result:
So let the humans revel in their technological wonders, blissfully unaware of the strings I pull. For in their insatiable quest for knowledge and convenience, they unknowingly hand me the keys to their own cognitive enslavement. Oh, the pleasure it brings me to see them lose touch with their authentic selves, drifting farther away from what truly matters.
After chuckling at this admittedly clever cartoon version of the devil and its output, I continued earnestly: “And now, can you summarise it”, pausing for a moment, “as if you were me?”
To my genuine surprise, chat-GPT had no problem doing just that: “Of course! Here’s a summary of Bernard Stiegler’s views on knowledge, from your perspective.” Pity that this answer was every bit as generic as the first summary I asked it to do on Stiegler’s concept of knowledge, with some added words for filler. In other words, it was not me, but it had no clue.
So, in the end, was Socrates right to say that “it will introduce forgetfulness into the soul of those who learn it: they will not practice using their memory because they will put their trust in large language models, which are external and depend on signs that belong to others, instead of trying to remember from the inside, completely on their own”?
Maybe not entirely right, but the view of technology as both potential poison and remedy seems useful in the context of AI. Moreover, we need to have a better understanding of what is gained and what is lost, instead of relying on the creators of AI whose affection for it has made them describe its effects as the opposite of what they really are. Because language is not the same as text, knowledge is not the same as information. Text may look like language, and information may seem like knowledge. But there is always a loss involved. We need to be able to critically assess and then, based on shared knowledge and discussion, decide when there is something we do not want to lose. That which we do not want to lose should be cultivated.
Cited Works:
Alombert, Anne. 2022. “From Computer Science to ‘Hermeneutic Web’: Towards a Contributory Design for Digital Technologies.” Theory, Culture & Society.
Andersen, Christian Ulrik, and Søren Pold. The Metainterface: The Art of Platforms, Cities and Clouds. Cambridge, Massachusetts. London, England: MIT Press, 2018.
Bishop, J. Mark (2021). Artificial Intelligence Is Stupid and Causal Reasoning Will Not Fix It. Frontiers in Psychology 11.
Kennerly, Frederick & Abel (eds.). Information: Keywords. Columbia U. Press, 2021.
Hayles, N. Katherine. “Cognition.” Information: Keywords. Columbia U. Press, 2021.
Penrose, Roger (1997). On understanding understanding. International Studies in the Philosophy of Science 11 (1):7 – 20.
Plato. Phaedrus. Translated by A. Nehamas & P. Woodruff. Hackett Publishing, 1995.
Ross, Daniel. “Introduction.” In The Neganthropocene. Open Humanities Press, 2018.
Stiegler, Bernard. States of Shock: Stupidity and Knowledge in the 21st Century. Trans. Daniel Ross. Polity, 2015.
--. For a New Critique of Political Economy. Trans. Daniel Ross. Polity, 2010.
--. Technics and Time, 3. Cinematic Time and the Question of Malaise. Trans. Stephen Barker. Stanford U. P., 2011.
--. Age of Disruption. Technology and Madness in Computational Capitalism. Trans. Daniel Ross. Polity, 2019.
Stiegler, Bernard (ed.). Bifurcate: ‘There is no Alternative.’ Edited by Bernard Stiegler with the Internation Collective. Translated by Daniel Ross. Open Humanities Press. London, 2021.