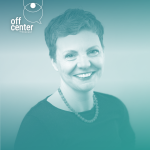
Rettberg and Rettberg discuss Jill's new book on machine vision and, in doing so, cover the mythological origins of surveillance, cybersemniotics, and the role of fear in the technologization of Western society.
SR: Today I’m here with Jill Walker Rettberg. Welcome, Jill.
JWR: Thank you, Scott.
SR: Jill, can you introduce yourself and remind the audience of who you are and why you’re here?
JWR: Yeah, I’m Jill Walker Rettberg, Co-Director of the Center for Digital Narrative, and I’m also a University of Bergen Professor and just published a new book, Machine Vision: How Algorithms Are Changing the Way We See the World.
SR: And that’s what I’ve invited you here to talk about today. This is a new book that you’ve been working on for a number of years. It’s the product of your European Research Council project that you’ve been running for, I think three or four years now?
JWR: Five and a half.
SR: Five years. Geez. I feel like I’ve been living with machine vision for a long time.
JWR: Yes, you have.
SR: This is a new book from Polity Press, and you really cover the topic of machine vision quite well, you explore it from a bunch of different angles. And I hope we can talk about some of the issues raised by these new technologies that involve different kinds of cameras that surveil us and that we use in different ways, and the systems that they’re connected to, that actually make judgments about human behavior.
JWR: Mhm.
SR: So why don’t we just start by talking a little bit about the history of machine vision, which you delve into in the first chapter of your book.
JWR: Yeah. I like to view technology as part of a long historical development, so I go right back to the first mirror, which was made of stone, 8,000 years ago. So the first mirror, that we found, anyway — it’s shaped sort of like a semisphere, so you can fit it in your hand, and it’s made of polished obsidian, that really shiny black rock. Archeologists found this in what is now Turkey today, and there’s a picture of someone holding it and it just fits your hand perfectly. Sort of like the way you might hold a phone today to take a selfie. So, I thought that line was really interesting, like these first early visual technologies, to the way we also use technology to look at ourselves today.
SR: So you’re interested in the idea of augmentation of our own sensory apparatus of vision?
JWR: Yeah. I mean, humans have used all sorts of technologies to see more of the world for a long time. There’s our mirror, but, you know, fire, for instance, was used to be able to see in the dark, and the ancient Greeks used these glass prisms to help them see detailed work, engravings and so forth, better, which over the millennia becomes, not just spectacles, but also things like glass telescopes that allowed Galileo to see the moon clearly and figure out the shape of it and the mountains and everything. So these different ways we’ve sort of extended our own vision have really been very significant in human culture.
SR: And I guess there’s been a lot of mythology around vision as well, that you touch on a little bit in your book.
JWR: Yeah. I start the book with the story of Argus, who was a giant. He had 100 eyes all over his body. And the story goes that Hera, Zeus’s wife, the queen of Olympus, was quite jealous of Zeus, who had many affairs, among others, one with a woman called Io. Hera was furious, so Zeus turned Io into a white cow, as one does, and Hera made sure Io was way, way far away from Zeus so Zeus couldn’t, you know, have his wicked way with her anymore. And Hera sent Argus, with all the eyes, to keep watch over Io. So even back then, this idea of surveillance and just always keeping watch as a way to sort of stay safe or protect or stop people from doing something, right?
SR: And that’s omni—
JWR: Omnivoyance.
SR: Omnivoyance, yeah.
JWR: Although that’s more, you know, the Christian tradition has the God who can see everything. Argus, I mean, he had a lot of eyes, but they were all over his body. So, some were in the back of his head, and the idea was that none of them ever fell asleep — it was like constant surveillance. But Zeus sends Hermes, the trickster god, with his pan flute, and Hermes played such beautiful lullabies that all of Aurgus’s eyes fell asleep, and so Zeus was able to run off with Io again.
SR: And he got the input/output.
JWR: The input/output?
SR: I/O is now—
JWR: Oh, ha ha! But that’s sort of a theme that I look at in the book, the sort of hope, the idea that you can use machine vision or surveillance to control people or to stay safe — but then it’s flawed, like Argus doesn’t actually succeed in keeping watch over Io.
SR: Right. So, I guess that’s kind of the argument that you’re making throughout the book, that we use these technologies, we rely on them, but you would maybe say that they fail us more than they help us?
JWR: Oh, I don’t think I’d go that far. I like technology — I mean, one of the things we worked with in the Machine Vision project was looking at narratives about machine vision, right? Which I think is what brings us closer to the Center for Digital Narrative, this idea that the stories people tell about technology are really significant.
SR: Yeah, I guess in some ways they kind of shape the way that technology is made. I always use the example of the iPhone and its relationship, I think, to the tricorder of Star Trek. And maybe this is wavering a little bit off topic, but often when I hear what the internet billionaires who are currently making AI systems, they’re also the same people who are warning us against the existential risk of AI. Some of them are saying “well, we need to be very wary of AI taking over the planet and deciding that humans aren’t useful.” And a lot of times, sure, maybe that’s a possibility, but I also wonder just how much science fiction these people have read, because a lot of the narrative seems to be based around not the actual implementations of the technology or the work and the mathematics that underlies it, but instead, these sort of dystopian fantasies that kind of guide the way that a lot of these internet capitalists are thinking.
JWR: Yeah. I mean, the trope of the mad scientist that creates artificial life or AI is really, really common in society, right? You’ve got Frankenstein, but you’ve also got even older stories like that, not just Argus, but Talos was a giant. There’s this idea that you have these machines that are stronger than humans that we create, but they’re going to destroy us is really, really old. And there’s always an Achilles heel or a screw that can be removed to kill the monster we created.
SR: Okay, so let’s move forward from spectacles, which, of course I rely on, and the kind of basic cyborg augmentation of vision that we’re all familiar with and move on to the history of photography and of video recording experiences and technologies that we’ve had up into the present. Because it seems to me that at this point you’re thinking about not only the sort of vision at the level of how we can use technology to see, record, remember, playback, experience or things that we see or enhance the things that we see — but where computers come in, and to a certain extent, where AI comes in and then somehow responds to what it sees. Is that the sort of new layer that defines contemporary machine vision?
JWR: Yeah, AI assisted vision. Although as we worked through the project, I mean, even the term vision is of course too narrow, because while, you know, if you think about a self-driving car, what it sees, there’s visual information that comes in, but there’s also other sorts of data flowing into it, right? Like, it’s geolocation, lidar, things like sensing distances or shapes or building models and stuff like that. So, there is a point where these AI assisted visual technologies are really converting, whether we see it as visual or not, they’re converting all these things into data.
SR: So maybe it’s really machine sensing?
JWR: Yeah.
SR: Maybe you should go back and change the title of your book to Machine Sensing?
JWR: The visual is incredibly important to humans. And one of the interesting distinctions is that we use visual things to help us create things — there’s a difference between representational images and operational images, where representation is, you know, the Mona Lisa or some pretty picture of something, whereas an operational image is an image that does something. So, like the images a self-driving car uses are operational. One of the things I found interesting when I was working on the topic is that if you go back, you realize operational images have been around for a long time — like when they came up with perspective drawing, it’s basically using an algorithm to convert the 3D world to a flat 2D drawing. That also leads to technical drawings, which are very specific diagrams that show exactly how to build something. And before they had those, every time somebody built a new thing, it was a craft, it was something they learned from another person. Once you have technical drawings that are really precise, you can build something without having a person teach you how to do it, right? So there’s a lot of ways in which technologies are sometimes more about, like, the software or the ideas than just about the physical machine.
SR: Yeah, yeah. I guess if we think about the way that the contemporary drone operator, the way that they’re being used, currently in the Ukraine war, where you might have someone who’s seeing from the first-person perspective of a drone, in certain cases, maybe even the way that they direct their head or the way that they touch an image on the screen can actualize real world effects, like dropping a bomb on someone or speeding a drone towards a target and blowing up a tank. That’s a very powerful level of operational image there. We think of that often when we talk about machine vision, about the sort of somewhat malevolent potentiality of these technologies. What are some of the positive uses of machine vision?
JWR: I mean, I love the playful uses. I love selfie filters and stuff like that. I love being able to video chat with someone on the other side of the world. It’s brilliant. I don’t like being stuck in a Teams meeting for too long, but it does open up the ability to access things that are distant, which is really evocative, right? And a lot of people have played with that, like one example: It’s well known from film history, Dziga Vertov’s Man With a Movie Camera, from like early Soviet cinema, where they’re just absolutely entranced with how this new technology, a movie camera, can see the world quite differently from humans. And they have this whole manifesto where they kind of argue that the movie camera needs to be freed, it’s enslaved by humans. There are sort of parallel thinkings about how AI is working today, right?
SR: Yeah. That we need to free the AI—
JWR: Oh yeah.
SR: From all this human interference that’s getting in the way.
JWR: Well, it allows us to see in slightly different ways, and that’s also one of the basic points of art, this sort of defamiliarizing of everyday life, so you see things a little bit differently.
SR: Well, and what about autonomous vision systems? I don’t know if that’s the right word for it, but the systems that see things, sense things and then make, you know, I think what Kael would call cognitive judgments about them, and then interpret that as a kind of meaning and then take some action in the real world. What are some of the benefits and problems of that whole cycle?
JWR: Well, one of the examples I use in the book is Alfred, our robot vacuum cleaner, who is an example of, you know, a pretty simple creature — I keep calling him a creature, I’m zoomorphizing him; not anthropomorphizing, not treating him as a human, but zoomorphizing him.
SR: Well, he is a butler.
JWR: He does clean our floor, it’s lovely. And he is a machine. But Alfred, he inputs certain sorts of data, right? He — maybe I shouldn’t say “he”, it’s going to confuse the listeners. It’s a vacuum cleaner. Alfred senses visual information — there’s a little camera, right? And also lidar, it bounces these rays off of objects to find a 3D space and creates a sort of map of the apartment. If Alfred bumps into, you know, the leg of a sofa or something, it responds to that and moves back.
SR: And it found my hat. You used that example in the book: My favorite hat had been missing under the couch for, I guess it was probably six months, and we got a message from Alfred one day that it was obstructed, and it sent us a picture, and that picture was of my favorite hat. So I’m eternally grateful to Alfred for that.
JWR: Alfred is super helpful. But Alfred doesn’t know what a hat is. Alfred just sends photos of obstacles.
SR: Yeah, but it says, “hey Scott, your hat is missing.”
JWR: But Alfred helped me think about what Katherine Hayles calls cybersemiotics. So, semiotics is like studying signs, and most people might have heard of it because of studying literature or just understanding, like writing is a sign, or a picture is a sign, or even smoke as a sign that means there’s a fire. Biosemiotics is thinking about how animals also interpret signs in different ways. For instance, a tree would interpret the data of falling temperatures — it gets colder in autumn, right? The tree senses cold and shorter days and interprets that as, “oh, winter is coming.” And so it withdraws the sap from its branches and leaves fall. So you could say that the tree is reading a sign, and so Katherine Hayles takes that on to cybersemiotics, which is how machines then also interpret signs. And Alfred obviously does interpret signs, you know, it saw the hat on its camera.
SR: And it could certainly be programmed to say, “this is a hat” and then send a message that says, “this is actually a hat”, rather than a sock or a shoe.
JWR: See, our colleague Jason Nelson at the Center for Digital Narrative keeps saying he’s going to make a robot poetry machine based on a robot vacuum cleaner. So, I’m really looking forward to that.
SR: Yeah, soon as we have the room to do it — and hopefully there’ll be no liability involved.
JWR: What, are you thinking people will trip over it?
SR: Yeah, people tripping over this robot that’s spouting sonnets at them and then suing the university. I’m not super worried about that, but—
JWR: I think it would be cool.
SR: It could happen. Well, let’s talk about surveillance and machine vision. Of course, there’s different levels of ways that we could surveil using machine vision. An extreme one, I guess, would be the sort of Chinese social credit apparatus, which is the idea that they’re sort of tracking people, not only through things like the use of credit cards and criminal records, but also physically in spaces or, for example, using cameras to look at how children are paying attention in class and then giving them credits or demerits on the basis of their attention.
JWR: Based on their facial expressions.
SR: Right. “You know, you seem to be thinking about something else. Therefore, you get a C-minus.” What a dream for teachers, huh?
JWR: Yeah, maybe not. So, in Australia, they installed surveillance cameras in classrooms. They got a federal grant to pay for this, and the idea was that it would simplify attendance taking, you know, where the teacher writes down which students are present every morning. Except it turned out the teachers didn’t much like it. I mean, one reason was they kept having to troubleshoot it when it didn’t work. But the main reason was that that thing where you take attendance of students, it’s not necessarily a waste of time: It’s a point where the teachers are able to, like, look into each student’s eyes and see how that student is doing today. And I think this is often a problem with technologies and machine vision: We think it’s going to save time and we want it to do things that seem boring, but actually are really important for that kind of social interaction, that feeling that we’re part of a community, that other people see us.
SR: That you’re being seen, yeah.
JWR: Well, yeah, but being seen in a good way, right?
SR: Yeah, maybe in a good way. If you’re a kid in class, you know, some kids want to make eye contact with the teachers and—
JWR: Some would prefer just a surveillance camera?
SR: Or prefer to just not be seen, or perhaps even prefer, you know, not to even be in class, and yet also have that not noticed by the teacher. Unfortunately, I think that’s the case. But okay, so I think we can agree that, at least in my view, coming from a sort of liberal democracy where privacy, at least used to be, somewhat valued, this sort of idea of the social credit system is bad surveillance. But then something you get into in your book is security surveillance. You write about things like the Ring camera.
JWR: Yeah. So the Ring doorbell is one of these cameras that people have on their front door that records what’s going on, and it can be set so that the owner gets an alert whenever someone comes to the door. And when we were living outside Chicago last spring—
SR: Oak Park.
JWR: In Oak Park, lovely town. A lot of the neighbors had Ring doorbell cameras, and there was also an app, which is actually not legal in Norway, but it’s legal in several countries: Neighbors, which is an app where you can upload your Ring doorbell videos and share them with your neighbors. So I tried the app, of course, and one of the first videos was of a carjacking. It was really very unpleasant to watch this carjacking, especially because it’s in an app — and this is one of your neighbors, and you can just hear just like the terror in the voice of the father as his wife walks out of the car with a baby in a baby carrier, and this guy just sort of jumps into the car and is threatening them. It’s a scary situation, right? Turned out it wasn’t actually very close neighbors, it was like ten miles away. But it feels so close.
SR: At the same time, having said that, there was a carjacking a half block from our house while we lived there.
JWR: Well, luckily I didn’t see the video of it, which is part of the point. And we know from all sorts of research on media and stuff, crime has actually dropped in the last 40 years — even though there was an uptick in the U.S post-pandemic. Crime has dropped significantly in the last decades, but people think there’s more crime and more crime is reported in the media. And lots of studies have found that if you see more crime in the media, including social media, you think that your neighborhood is more violent and you lose trust in your community. Fear is a really intense emotion.
SR: At the same time, there were more carjackings in Oak Park in the last several years than there were for a long period before that.
JWR: I haven't checked those numbers, but maybe there were. But I’m worried about this sort of fear as a driver for getting more technology and assuming that the technology will then solve all societal problems.
SR: Yeah. And this is something that we obviously have some slightly different opinions on. You actually exploited, or used, a story of when I was attacked in your book, in Chicago on an L platform. This was on the south side of Chicago, there were security cameras on the platform, but unfortunately, at the time of the attack, those weren’t actually used. Whoever had access to the video didn’t recognize that an attack was taking place, and then three subsequent attacks took place over the course of two hours. And so there’s maybe an example of, you know, where a vision apparatus or a vision assemblage, as you say in your book, failed. At the same time, they were eventually able to apprehend the suspects who did the crime as a result of the fact that that video existed. And I guess we sort of have some different perspectives or different views of the complications involved in this scenario: On the one hand, a world blanketed in security cameras and Rings on every doorstep might be a world that we don’t want to live in, in this sort of dystopian environment of continuous surveillance. On the other hand, when I was a crime victim, I was more frustrated that the technology was not used appropriately or thoroughly, rather than the fact that the technology existed. In other words, I thought that it would have been much more useful if someone was watching that camera at the time that it occurred. Or even if, let’s say that a machine vision system was watching the camera and identified this, when two people tackled someone on an L platform right near the edge, as suspicious behavior. Because I actually think that is suspicious behavior. And well, okay, so if this had been flagged and a message had been sent to the police, maybe it would have done something. I didn’t actually call the police and they didn’t come for an hour and a half.
JWR: Yeah, exactly.
SR: But yeah, I sort of have a little bit of a perspective on this, that maybe in certain situations this surveillance apparatus is of use.
JWR: Yeah. There are situations where it’s of use. But I think the main problem is that we think it’s good enough without all the other things. I mean, the real problem on the L platform was that they don’t have conductors, they don’t have people working there. The only person who was at work at that station was downstairs ignoring everybody, right? So you’ve already got this sort of erosion of trust in the community, and the surveillance isn’t helping.
SR: I mean, I think the basic social problems there had more to do with disparity of income and lack of opportunity and a sense of hope.
JWR: Yeah.
SR: And poverty.
JWR: Technology isn’t going to fix deep societal problems.
SR: Right. Although I wonder, in your book, you seem to argue that this is a prevailing opinion, that people think that once you put in security cameras, the problem’s solved. Do you really think that’s the case?
JWR: I think politicians certainly come up with it every time. Lori Lightfoot, who was the mayor of Chicago then, kept trying to push more cameras, more surveillance. They had ShotSpotter in Chicago, which is a system that if it hears, I mean, obviously it’s not hearing, but if it detects a sound that sounds like a gunshot, it sends an automated alert to the police. And they had an independent report on how this was working, that found it wasn’t working, it wasn’t reducing crime. In fact, it was causing extra work for the police and also had other problems. And even so, the politicians urged to increase that; to keep going. They’ve just suggested this in Sweden, where they’re worried about increased violence. Oh, 2,500 surveillance cameras, that’s going to fix it, not looking at the societal problems.
SR: Yeah. I wonder if people always argue that it’s going to fix it though, or if they think of it as a tool.
JWR: It’s just an easy fix, right? The tech fix, the idea that you can just buy safety instead of actually dealing with the root problems.
SR: Yeah. No, I understand both perspectives on that. Both sort of the urge to equip law enforcement with tools, given that there are technologies available, and the sort of problem of politicians, or, you know, to a certain degree, police forces, believing that this will just solve all the problems. Clearly, it’s a sort of a Band-Aid that in certain cases can be useful, and in certain cases might not be, but this belief in the infallibility of machine vision is deeply flawed.
JWR: And I think that belief is this really strong cultural urge. I mean, we started with the story of Argus and Hera, thinking that this giant that could see everything would take care of the situation. But the idea of the all-seeing God as well, the idea that God sees everything, is something we see in many religions, right? There is this sort of longing, longing for something to take care of us, that I think we often long for technology to take care of us. There’s a novel, a young adult novel, that I talk about in the book as well: Thunderhead, who’s this AI — so it’s a future society where there’s an AI that’s super benevolent, very, very kind, called Thunderhead, that just takes care of everyone. There’s cameras absolutely everywhere. Thunderhead just makes sure everyone is absolutely happy. Except in Texas, where the people didn’t want surveillance, so that’s a no-surveillance state.
SR: Yeah. And then of course you have things like, this is an example of benefits and problems of machine vision: If you think about the January 6th insurrection where you had hundreds of people attacking police officers and attacking the United States Capitol, destroying the building in various ways and kind of attacking Democracy — now, almost all of that was filmed, and not only by security cameras, but also by individuals in the building, who are doing the crimes, right? Taking videos and posting them to right wing social media. Later on, of course, these become a tool in prosecuting those people who chose to attack the Capitol. Probably a net societal gain there. But on the other side, of course, you have the right wing media, pushing for all the footage from all the cameras to be released and then sort of putting out videos that show the incident from a very particularized perspective, right? So that you can cut and edit footage to make it look like something happened that didn’t happen.
JWR: Yeah.
SR: So I guess this is just an example of the complexity of this sort of surveillance apparatus that, on the one hand it does provide enhanced security or enhanced law enforcement, at least. It didn’t stop the attack from happening, but it does seem to have had somewhat of an effect of discouraging people from casually engaging in that type of insurrectionist violence. You haven’t seen those sorts of incidents occurring, for example, at the arraignment hearings for Donald Trump, even though people thought they would be. There’s probably somewhat of a deterrent effect. But anyway, this is a question, too. The belief in surveillance doesn’t necessarily restrict itself to governments or even to corporations conducting surveillance, there’s also a kind of self-surveillance that’s occurring.
JWR: Yeah. I mean, we have cameras in our homes, some of us do. Are you thinking of things like selfies and posting?
SR: Well, this was also the case when I got attacked: when I saw the footage later, I noticed that there was another person on the platform who was filming the whole incident, right? And it appeared that the act of filming it — to be shared within a social network, whether it was on social media or not, or on a messaging application — that was part of the point of the attack, to surveil the attack and then share it as a form of entertainment. And I’m just wondering, this whole idea that we need to film everything and that we need to take selfies whenever there’s sort of a special occasion, and I always wonder, who are those images for? Sure, we can share them momentarily on social media, but actually we’re sharing them with a computer network. The computer network tends to hang onto those images longer than the humans do, and we tend not to print them anymore, we tend not to put them on the wall. It’s something about the act of just surveilling.
JWR: I don’t know that I would use the term surveillance for all those things, though. I think there’s different urges. I mean, the act of capturing or taking a photo is also a way of sort of remembering it in itself. Even if you never look at the photo, it’s like you’re stopping, you’re looking, right? It’s a bit like making a note in a diary or something like that. It can be more about the process than about the product, I think.
SR: And you’re stopping and you’re looking and then you’re uploading it to a cloud, and oftentimes you’re uploading it to a service that uses AI and image recognition to say this is the person that was recorded on this date. To me, that sounds a little bit like a form of surveillance. However, here’s another example: Today I was on Facebook and a video from, I think eight years ago, popped up of the first time my child, Benjamin, rode on a roller coaster, and his response after the roller coaster ride. And this is one of these Facebook memory things, where Facebook is sort of remembering for me, and that’s definitely machine vision, right? That’s a system that I voluntarily gave my data to that did some image recognition, somehow marked this as important and then remembered for me. That’s weird and also wonderful too, right?
JWR: I mean, what I’m really interested in are these relationships between humans and the technologies — and other things in society, too, right? And then thinking about how we interact with algorithms, and we do things partly because we know the algorithm is going to respond in that way.
SR: Yeah. You write, in another chapter, about being seen in the algorithmic gaze and sort of resistance to machine vision. Can you say a little bit about that?
JWR: Yeah. I mean, one aspect of the algorithmic gaze is this sort of dream of being looked after, right? Which I guess you touched upon a bit, and I mentioned Thunderhead. There are the fictions about the totalitarian 1984 style surveillance state, but also about this sort of loving surveillance, the beneficial, that just takes care of everything and makes sure everyone’s safe, so that’s part of it. But also I talk about how this algorithmic gaze, or when you have AI involved in things, it’s normalizing, right? It’s a normalizing gaze. And for instance, if you train an AI, like generative AI on, you know, 100,000 pictures of men and women and all sorts of people, it’s gonna generate faces that look more masculine, more feminine than the training data did. It finds the statistically likely patterns and it increases them. So it’s normalizing, it sort of cuts away the outliers and brings you towards this middle thing. And I think one of the things that happens when you take a photo of yourself, it’s actually just sort of subtly normalizing your face, maybe smoothing the skin just a little bit, increasing the contrast, doing things. And I think this is sort of training us to see the world a bit differently.
SR: Mhm.
JWR: There’s the example of how cameras optimize the photos automatically, and they’re actually optimized for Californian sunshine, usually. So there were examples when they had the wildfires in California and the sky was orange, but people’s cameras still sort of normalized the sky, so they looked gray instead. And the other day I was skiing here, so everything’s very white, but it doesn’t show that everything is very white. It sort of tries to normalize it and so it ends up looking kind of murky gray, which is really weird.
SR: Yeah, that is weird. Or the sort of night vision capabilities where, yeah, sure, pictures were always grainy, but now the iPhone is sort of adjusting them to be a kind of scene that’s not seen by the human eye.
JWR: Yeah. Or like the northern lights — there’s never been so many northern lights in Bergen since phone cameras got the night mode, and often they look a lot better on the camera than in the actual sky.
SR: Well, maybe we live in a better world thanks to our machine vision enhancements. You know, to come back to this idea of sort of resistance, of people wearing different kinds of camouflage to fool machine vision or having these different sorts of strategies as a kind of protest. At the same time, I think we see a lot of these in art projects, but we don’t see it a lot in actuality.
JWR: Maybe not. And artists are really good at hacking machine vision, at showing ways you can trick it and protest. You know, in New York they have had mask laws for over a century so that people can’t hide their face when they’re in a protest or something like that, but you do see in protests that people actually wear things on their face to stop the surveillance cameras spotting them. That does happen, but apart from that, it is perhaps mostly the art projects, which I think are largely about just pointing out where it breaks, right? There are so many ways that machine vision can be tricked, and my colleague Linda Kronman, who was also working on the Machine Vision project, has written quite a lot about hacking machine vision in digital artworks, and she just finds so many examples of this sort of trickery. So in one of the chapters, I talk about the trickster figure in all these science fiction stories and so forth, because there are lots of examples of humans maybe not breaking the machine vision or using that kind of camouflage, but just evading it.
SR: Yeah.
JWR: It’s pretty easy to evade cameras.
SR: Yeah. Well, fairly easy. I mean, I think you can evade the cameras you know about fairly easy, but there’s such a saturation at this point that it’s probably difficult. I’m doing the devil’s advocate thing, but let me ask you: Let’s say that you have a loved one in their 80s, who’s perhaps in physical decline and beginning to fall a lot. Let’s say they’re getting close to 90 and they still want to live in their home. And here you have a technology, let’s say that if you fall, your watch notifies someone, maybe even it turns on the camera that’s connected to your Amazon network, gives you a call and notifies you, says “hey, here’s a picture of your mother, she appears to have broken an arm.” Is that a bad use of machine vision?
JWR: No, not if the person is happy with it. I mean, I think consensual surveillance is fine. But it’s when it’s not consensual that it starts getting problematic, or if I put up a surveillance camera in my mother’s house instead of going to visit her now and then. I mean, you could easily imagine bad uses for it, I think. But there’s lots of cases where technology can help.
SR: Yeah, well, I would say that, for example, that in certain it might cases enable people to live in their homes much longer than they would be able to without having that sort of helper machine vision system. So, it seems that we can’t make any kind of blanket conclusion, right? I don’t think you do in your book.
JWR: Right. My conclusion is titled “hope”, so that’s my blanket conclusion. And I actually did that very deliberately because as I was working with this, it is really easy to see problems. There’s bias in many of these technologies, there’s ways in which it’s removing community and increasing distrust and fear, which I think is really problematic. But there’s also so much beauty and amazing things that people are doing, and there’s fun and there’s play and there is hope. I think in the humanities, we’re really well trained at critiquing everything. And I think it’s really important to also find the hopeful things and help build better places to sort of actually see the good in the world.
SR: Of course. It’s a beautiful and scary world that we live in. And hopefully we don’t lose sight of either one of those things, that, on the one hand, we should be using these technologies in various ways, but that we need to do so from a critical, analytical, reflective viewpoint. I think you kind of make that point very well in your book. So congratulations on getting this out there. Now, we’re sort of able to move on to other topics, we talk about other things in the house now.
SR: It’s been a pleasure having you as we move forward into the second part of the Off Center series, and indeed the second part of the Center for Digital Narrative. We should maybe mention that we’re just about to move into the building for the Center for Digital Narrative.
JWR: I think we might need to do an audio tour or something once we have moved.
SR: Oh, no.
JWR: Aw.
SR: I don’t think we’ll do that, just because, geez, what are you going to do? Like “this is a big room. Here’s where we keep the coats. Oh, there goes Jason’s poetry robot. If you could see it, you would be amazed.” I don’t know, I’m just getting excited about thinking about where we put the foosball table. Okay, well, thanks a lot, Jill Walker Rettberg.
JWR: Thank you very much.
Listen to the full episode of Off Center.
References
Bogost, Ian. 2020. Your Phone Wasn’t Built for the Apocalypse. The Atlantic, September 11, 2020. https://www.theatlantic.com/technology/archive/2020/09/camera-phone-wildfire-sky/616279/
Hayles, N. Katherine. 2019. "Can Computers Create Meanings? A Cyber/Bio/Semiotic Perspective." Critical Inquiry 46(1). https://doi.org/10.1086/705303
Orwell, George. 1949. 1984. Secker & Warburg.
Rettberg, Jill Walker. 2023. Machine Vision: How Algorithms are Changing the Way We See the World. Polity Press. https://www.politybooks.com/bookdetail?book_slug=machine-vision-how-algorithms-are-changing-the-way-we-see-the-world–9781509545223
Shusterman, Neal. 2018. Thunderhead. Simon & Schuster.
Vertov, Dziga, Mikhail Kaufman and Yelizaveta Svilova. Man with a Movie Camera. 1929. 68 minutes.
This research is partially supported by the Research Council of Norway Centers of Excellence program, project number 332643, Center for Digital Narrative and project number 335129, Extending Digital Narrative.