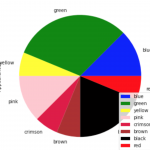
As the present gathering introduces Electronic Literature into the Digital Humanities, the DH at Berkeley Program brings the Arts/Humanities into Science, Technology, Engineering and Mathematics: turning STEM into STEAM.
Introduction
In this article, we advocate for a new kind of renaissance person, a humanistic data scientist capable of profound cultural analysis and critique. A person able to critique from deep within the technical infrastructure and someone who understands an age-old wisdom within the humanities—that knowledge is always a pursuit and never a completed journey. This journey requires an ever-expanding methodological repertoire to evaluate and understand (and seek solutions for) an increasingly complex and dystopic world. At the same time, our pedagogical approaches encourage experiential learning that engages all of the senses and ignites the imagination. If we can dream it, we can build it. A future where young scholars can learn how to deprogram from brainwashing propaganda, how to sort through fake news, and how to lift up their voices to become active participants in creating a new reality. This seems like a lofty goal, but the authors of this article feel that our very democracies depend on building savvy critical thinkers who can deconstruct the world around them. We currently see a highly problematic division between science and the arts/humanities in a false dichotomy.
A revitalized Science, Technology, Engineering, Arts/Humanities, and Mathematics (STEAM) approach to Digital Humanities (DH) drives our pedagogy and research with an emphasis on creative potential as a catalyst for scientific learning and to cultivate expansive mindsets that enable transcending disciplinary boundaries.
We discuss our self-sustaining DH at Berkeley Summer Minor and Certificate Program that grew out of a rich context embracing the artist and scientist within us. The DH at Berkeley Program is unique in that it teaches the foundational theories found across the arts and humanities in combination with qualitative and quantitative practices to provide comprehensive research methods and tools. Here we unpack our unique pedagogical philosophy and the ways we develop pedagogical content knowledge among our instructors.
Background
DH at Berkeley (“DH at Berkeley”) and D-Lab (“D-Lab”) forge collaborative venues that result in new methodologies informed from an interdisciplinary intellectual exchange. Since 2013, D-Lab has been the implementation laboratory for DH at Berkeley. Grounded from the lab, we build strong ties to departments, schools, and other centers at UC Berkeley, nationally, and internationally. We provide intelligent research design services that use rigorous computational methods via hands-on, active, and project-based learning. These include data acquisition and archiving, computational text analysis, machine learning, historical geospatial analysis, network analysis, and virtual reality projects. Collectively, we reach a broad audience through 1,400 consultations, 300 workshops, and dozens of courses every year. Our diverse scholars come from every facet of campus and beyond, and therefore influence one another by discussing their distinct methods and approaches, and ways of understanding. We are not interested in constructing critiques from afar; instead, we immerse ourselves in computational methods to interrogate them and raise concerns when people use these technologies, algorithms, and techniques without critical analysis of the damage they can cause through the perpetuation and amplification of bias. Our services strengthen faculty research and graduate and undergraduate programs in the humanities and social sciences and in the Division of Computing, Data Science, and Society (“CDSS”). We echo our colleagues from CDSS Human Contexts and Ethics and emphasize how ethics are “...social, shaped by history, instilled in institutions, and dynamically produced with our technologies” (“UC Berkeley History Department”).
We remove barriers for entry, so novices learn through cascading mentorship structures and cognitive apprenticeship to produce collaborative, cross-disciplinary research projects (“Projects,” Vygotsky 1978, Vihavainen et al. 2011). Our scholars begin at the periphery of our community and slowly move into the core of our work. To teach, scholars need to learn methods more thoroughly than they thought possible. They become proficient in a wide array of methods and can implement these methods within their original research. They can expand their understanding of what is possible and can stop using the same tools for diverse questions. The products of these exploratory inquiries are various and unique. For example, Professors Alex Saum-Pascual and Elika Ortega mounted an unprecedented multilingual electronic literature exhibition and course titled “No Legacy” in 2016 (Saum-Pascual 2018). Such efforts culminate in a yearly DH Fair attended by hundreds of campus and community members. In 2019, the Berkeley Art Museum and Pacific Film Archive (“BAMPFA”) featured a conversation between Professor Zeynep Tufekci and members of the Hate Speech Measure project (“Measuring Hate Speech”, “Berkeley News”). A DH Fair and lightning talk poster gallery took place online subsequently in 2020 (“DH Fair 2020: Online”, “DH Fair 2020: Posters”).
DH at Berkeley
Physically (and increasingly virtually), we collaborate with every campus department, school, and organization. Our community is large and representative of many different interests, including e-lit critical making (“DH at Berkeley - e-lit.”). The DH at Berkeley Summer Minor (for undergraduates) and Certificate Program (for everybody else) encapsulates the core DH competencies during two six-week summer sessions (Table 1). We discuss the two core courses (DIGHUM 100 and 101) and three of the electives (150A, 150C, and 160). DIGHUM 100 and 101 teach students theoretical and computational foundations to demystify systems of algorithms and networks that often seem indecipherable while 150A, 150C, and 160 dive deeper into particular methods and concerns of DH research. These courses are immersed in various lecture series and exhibits, providing participants with exposure to the vibrant intellectual and artistic life at Berkeley. Together the courses are meant to provide a foundation in computational methods and ample opportunities to deepen student understanding through applied projects using large-scale social media data and multi-faceted analysis. The methods and practicum courses are calibrated and paced in order to optimize learning while they are taken during the same six-week period.
Type | Course | Name | Course Repository |
---|---|---|---|
Core | DIGHUM 100 | Theory and Methods in Digital Humanities | Google Site: https://sites.google.com/view/summer-dighum100-berkeley/home & GitHub: https://github.com/admndrsn/awca |
DIGHUM 101 | Practicing the Digital Humanities (Practicum) | https://github.com/dlab-berkeley/DIGHUM101-2020 | |
Elective | DIGHUM 150A | Digital Humanities and Archival Design | Google Site: https://sites.google.com/view/dighum150-archival-design/ |
DIGHUM 150C | Digital Humanities and Textual and Language Analysis | https://icla2020.jonreeve.com/ | |
DIGHUM 160 | Critical Digital Humanities | https://github.com/tomvannuenen/digital-hermeneutics-2020 |
The DH at Berkeley Pedagogy
DH at Berkeley seeks to develop domain competence, beginning from peripheral participation down the path to becoming a domain expert. Through various examples of student work in this article, we show that patience and repetition are key as we find analogies to a painter practicing the same stroke or pattern, or a dancer performing the same sequence of moves thousands of times. We believe in learning by doing, active exploration andexperimentation without fear of failure. All the while, we provide scaffolding of information and coaching both to instructors and students. Cognitive apprenticeship is deployed through transparent problem solving think-alouds. We confront math phobia head on and show that computational methods are for everyone and that key to the process is becoming autodidactic. To that end, we emerse our students within communities of learners. We background skills and foreground competence. Foundational to our educational program is a dialectical approach, verbal discussion and debate, through online annotation programs of key literary texts that can be conceptualized as e-literature, for example using Hypothes.is and qualitative data analysis tools such as MaxQDA. These efforts either begin or culminate in designing, constructing, and curating multi-modal web archives. We invite our students to tell us what is working and what we are doing wrong in our own ongoing cycles of improvement.
We build inclusivity by breaking from normative computer science “bro” culture and high-brow arts and humanities culture centered on the Western canon that excludes non-dominant cultures and people. We explicitly teach our students how to actively work to reduce bias and raise critical awareness of issues that differentially impact underserved people, and that it is okay to not be familiar with computational tools and arts and humanities theoretical frameworks since not everyone grows up in environments with computers or exposure to classical Western culture and thought.
Because interests are so diverse, we do not believe in a “one-size-fits-all” approach and do not typecast these topics in dichotomous ways, such as “scientific” versus “creative”. To apply STEAM principles, we first start with student interests. If STEM is their start point, we balance that with contextual guidance that the arts and humanities provide expertly, thus adding the “A” to STEM to produce STEAM. Alternatively, students who start with a stronger arts and humanities approach receive technical training and a more robust foundation within computation and statistics. This tailored teaching approach ensures each student gets a mixture of creative and scientific exploration.
We help students morph their subject of interest into a research question that can be answered within a short timeframe by encouraging them to ground their interest in a specific discipline and by ensuring that students can realistically investigate and operationalize their ideas. Many students have a passion for a field, author, or method to inspire their research ideas. We guide novices through the ideation stage in “high-concept/low-tech” ways to build their conceptual understanding systematically. At the same time, more advanced theory and code challenges more proficient students. Students flow through iterative cycles of research questions, fine-tuning and planning to operationalize a framework to answer that question. One DIGHUMH 100 student, Jeffrey Cheng, proposed an analytical comparison of the biblical canon and apocryphal texts for his study. He initially examined thematic differences between the Old Testament and Apocrypha as well as specifics of diction and writing style (sentence length, word type frequency, etc.). After surveying tools and methods, Jeffrey chose a wider initial scope and used a combination of topic modeling and word embedding tools to compare the books. This allowed him to articulate a discussion based on the resulting clusters for each book that informed more specific questions for word-level analysis based on the statistical similarities of his initial phase (Cheng 2020).
Students learn how to create research “workflows” to conceptualize and organize their research processes. Ultimately, tools and methods are at the service of the epistemology (the theory of knowledge), the ontology (domain concepts and categories or domains of discourse), and the implied underpinnings of the question that the student is asking. Students move beyond data analytics and regurgitation of their coding training to include organization, contextual interpretation, and documentation at every stage of the research process. We provide students with example projects and walk them through common pitfalls and encourage transparent, open, and reproducible scientific practices.
Students often describe to us their need to conduct independent research despite never having been taught explicitly how to design and implement their research. We break down the research cycle and we teach them to think critically about organizing their research workflows for short-term course projects, but also how to envision their longer-term research goals and connect these to their educational and professional trajectory. This is exemplified by one of our students from Art History, Kenny Oh, who worked on a research project with Professor Elizabeth Honig on pattern recognition across contemporary works of art. He was later introduced to the latest tools for image recognition using neural network machine learning algorithms, and after graduating from UC Berkeley chose to pursue an advanced degree in deep learning methods for image recognition (Oh 2018).
In these contexts, we also distinguish between “skills” and “competencies”. “Skills” refer to the mere regurgitation of facts devoid of any context, such as how to code a random forest machine learning algorithm. Instead, we teach students “competencies” which are skills applied to highly-contextualized problems, such as how to code a random forest algorithm to examine gender politics in a corpus of texts and how to compare that algorithm to evidence from close reading and then write about the results and present them. For example in DIGHUM 150A, Chloe Akazawa designed a website archive (Art Up Close), which she curated to mediate a more interactive experience for art combined from different museums. While the primary result of the course was a website, the students had the chance to iterate on their deliverables by describing their process in blueprint form and with a video (Akazawa Blueprint 2020
, Akazawa Video 2020
). In the following section, we discuss how we provide professional development for our instructors in order to create the experiences described above.
Instructor Preparation
Instructors must receive professional development training and become familiar with their multitude of interdependent roles and responsibilities. This system creates professional development opportunities for instructors. Instructors better understand how to support individual student success through training, coaching, and peer-to-peer analysis of course content and delivery.
We first ensure that instructors embody values of inclusivity and emphasize the importance of continual communication with the students, such as by sending welcome messages and surveys before the class even starts to assure they can install the necessary software, gain access to the course materials, understand technological requirements, and begin to brainstorm about project ideas. Early orchestration of technology and a research-bound state of mind are integral parts of students’ engagement and success. Instructors also show how students can establish and refine their scientific identities, such as by joining online communities of practice and helping connect students to opportunities for growth through learning, writing and presentation, and graduate school, internships, and career pathways.
Instructors participate in mock teaching exercises and are supervised by staff to ensure they receive adequate practice. Here, instructors learn how to optimize Zoom and share best practices for using other forms of collaborative technology, deliver and pace content, explain and contextualize jargon terms, foreshadow pain points, answer questions, and encourage student ideas. They also learn the importance of group work, how to build student morale, inspire and motivate projects, build group cohesion, project management principles, hold office hours, all to build empowered community. Instructors review curricula in committee settings to ensure that concepts, topics, tools, and methodologies remain cutting edge and to explore the potential development of new courses and workshops. Each section of a topic is presented in a systematized way: introduction, brief lecture and coding walkthrough, and then challenge questions where students can test what they have learned in applied settings. This is followed by instructor-prompted group discussions that explore the concepts, explain syntax, and debug errors, placing special emphasis on inclusion and participation. Formative assessments are sprinkled throughout each session via flash questions and real-time polls, reflection of discussion participation, and written responses to assignments. Recording lectures, creating slides with coding walkthroughs, and facilitating online discussion boards are important components for students to be able to revisit the main arguments from each class in addition to scenarios when asynchronous options are necessary such as vast time zone differences. Pre- and post-course surveys are employed to evaluate student and instructor perspectives. These data help inform course development in iterative cycles. In the next sections, we detail each course and provide examples of student work.
DH Theory and Methods
In the DIGHUM 100 (Theory and Methods), we teach students discursive critical thinking skills through formalist, structuralist, and poststructuralist modes of analysis. Students first identify an archive or body of work and use exploratory data analysis to learn about it. They learn how an archive is defined and digitally curated, the boundaries and parameters that make their research defensible, and how to formulate a research question to investigate that archive, even if they select an already existing one. In many cases however, students are interested in world heritage that has not yet been assembled into archive form. For example, one student, Shereen Kasmir, extracted collections of texts from digital libraries such as the UC Berkeley Library and Project Gutenberg to explore the definition of happiness across different cultures and time periods. As her archive took shape, she described her future work for the project “to enlarge the corpus to get resources from people of different classes and wealth to see if that has a meaningful impact. Moreover, for contemporary opinions, I could also include personal testimonials from Twitter and Reddit to get more subjective data” (Kasmir 2020). In this way students are empowered to build their own conceptual archives with expansive datasets online, and design a workflow which is driven by an empirical question, informed by critical interpretation, and paired with the most fitting tools for data analysis. Additional software such as qualitative data analysis tools (MAXQDA), Tableau for data visualization, and Gephi for network analysis, are also introduced.
This course is synchronized with the DIGHUM 101 practicum course (see below) so that students learn step-by-step Python instructions for quantitative analysis in combination with qualitative, theoretical approaches. Python is an open-source and free (to the user; not to develop) programming language, has many pre-built functions for data acquisition, cleaning/subsetting, visualization, and analysis, and also allows the user to build their own pipelines for functions, workflows, and projects. Binder (“Binder”) was also configured to give students web-based access to all course materials when the students’ local installations failed or their computers crashed. As each student already had a Google account, in DIGHUM 100 we worked in Google’s Colab in order to create a coding sandbox, where students could learn and share the tools that could be used for exploratory data analysis, while also getting assistance on how best to fit the tool to their dataset and fine-tune it as they interpreted their results. Additional communication was facilitated in course management system called Piazza, which we implemented after receiving an overwhelmingly positive response from the students.
This approach illustrates both creative and critical thinking as well as being founded on scientific methods. Students conceptualize research questions based on their own interests and refine them by developing sub-questions through a process of thematic revision and conceptual workflows. They identify themes and discern sources of evidence for each of these themes to craft arguments that emerge from both scientific and creative artistic pursuits. In many instances, the evidence they seek to answer their research question exists in a complex system of relationships that can be examined using a network visualization and analysis tools such as Gephi (“Gephi”), which has a gentle learning curve for importing data, defining network characteristics (such as nodes and edges), and plotting relationships between data points.
From this foundation, students develop a short “elevator pitch” of the data source, question, results, and their interpretation that is presented through different media: it involves a slideshow, website archive demonstration, video, GitHub repository, and code in Python Jupyter Notebooks (“Python Jupyter Notebooks”). For example, Eunice Chan’s (Chan 2020) work focused on a diachronic study of pop music in a project titled, Lyrics Through Time. She used Wikipedia to select the top songs from the Billboard list since 1950 to see how music changed over time, noting that “music both shapes and reflects the social and political climate of the time.” She used Python Jupyter Notebooks to compute term frequency-inverse document frequency (TF-IDF) scores for each of the songs and generated word clouds and line charts to track changes in word choice over time. She also applied a series of theoretical approaches, beginning with historical, then Marxist, and lastly with feminist criticism. Consequently, these different frameworks informed her conclusions that use of female pronouns in lyrics declined over time and were replaced with more possessive personal pronouns, reflecting a possible trend towards capitalist consumerist themes. For another project, Eunice also used Python’s “keras” library (Chollet 2020) to construct deep neural networks to classify almost 20,000 European paintings from the WikiArt (“WikiArt”) archive into twenty-five different European traditions from painters over 400 years. Temporal bias caused her to refine the research question, focus on a smaller subset of six countries, and investigate performance differences in her models. She noted that different neural networks classified the paintings differently based on nuances of their mathematical structures - a calculus foray into art history.
A different student, Teresa Goertz, used Python’s “zhon” library (“zhon”) to examine zhiguai, classical Chinese records of strange events, from the Ming (1368–1644) and Qing (1644–1911) periods that depict non-fictional, sometimes supernatural occurrences about human nature, creation, spirituality, the natural world, and culture that are not easily explained by logic. Not only was this student tasked with learning the Python programming language, but completed this task in a second natural language. This student investigated what types of beings other than humans existed (living or dead) in hundreds of short stories and how themes differed across collections. Despite the supernatural themes, the morals of the stories often focused on the circumstances of humans: self-awareness, challenges faced, love won and lost, and society writ large. She published her work by building an Omeka exhibit to guide readers through the narrative features of the Gui and Guai and also described her workflow (Goertz 2019). Other students looked at diverse topics that blended computational methods and humanities: bodily representation in French literature; themes in Blood versus Crip West Coast gangsta rap lyrics; which jobs have been impacted the most by income inequality in the last twenty-five years; the appeal of right-wing politics; vitriol in the now quarantined “r/The_Donald” subreddit thread; advertising in Korean leather handbag markets and cultural uniformity; sentiment analysis of religious texts (i.e., how positive or negative the attitudes within the texts are); theoretical relationships between media and democracy; geospatial maps of the relationships between human population and Ozone levels in California; and postcolonial appropriation of pre-colonial architecture in photographs.
All projects require students to learn through creation, thus they are able to retain complex theoretical ideas through the use of empirical studies. In each of the student examples, we see a progression from the theoretical design of their project to the creative space (e.g., Python, Omeka, and GitHub) in which their project is built and curated, and lastly, the dissemination of this work to a public audience through a presentation and video. This final step brings the students back full circle to the critical theory they began with: in order to frame their research questions, interpret their results, and to be mindful of the diverse identities in the audience. Finally, students learn that working in groups is a fundamental aspect of research. Inboth DIGHUM 100 and DIGHUM 101, students are tasked with both individual and group projects. Students are divided into groups of two, three, or four students to investigate bias, diversity, and inclusivity in DH, institutional racism and police brutality, and big data. Students are paired up in heterogeneous groupings across disciplines in order to spark student creativity, which also leads to many opportunities for peer evaluation and multiple presentations.
Practicing the Digital Humanities (Practicum)
DIGHUM 101 (Practicum) is the foundational coding experience of the DH at Berkeley Summer Minor and Certificate Program and students here learn coding basics.This course is composed almost exclusively of Python coding in Jupyter Notebooks (along with basic Bash and GitHub described below) but still emphasizes writing practice for research prospecti and presentation of results. In addition to preparing students for their programmatic tasks to complete assignments and projects detailed in the sections above and below, students here were expected to compose and explain a functional Python Jupyter Notebook to their classmates, give group slideshow presentations on bias and diversity in DH, and record short videos that reflected their learning experiences. Students also learn how to use basic Bash programming (“Bash”) to share their data, code, results, and individual and group presentations on GitHub (“GitHub”), the world’s foremost code storage and version-tracking collaborative tool (Akazawa 2020, Jiang 2020, Ku 2020, Parekh 2020, Subramanian 2020).
In this course, students use these skills to acquire text datasets from literature, social media data, and other media in order to use Python with confidence. Basic coding is emphasized early on, before students learn how to import and visualize data with an emphasis on text preprocessing. These modules are followed by the basics of supervised and unsupervised machine learning methods. Here, our instructors shine because they insert themselves into the “beginner’s mind” to remove as many assumptions and as much bias as possible to help students grasp complex concepts in simple ways. Natural language analogies can help. For example, to help illustrate Pythonic functions and arguments, we can use the sentence “Evan threw the ball”. Because functions perform actions on things, we argue that the verb “threw” is the function in this sentence. The “thing” that the action was performed on is the function argument - “the ball”. We then illustrate Python’s print statement similarly. In the function print(“Hello, Digital Humanities”), “print” is the function and “Hello, Digital Humanities” is the character string argument. This also works with error message interpretations. Because the Spanish and Italian languages share vast lexical similarities, speakers of one language might find themselves in contexts where they could understand the other despite having no knowledge of that other language. However, in the absence of complex algorithms trained to do so, computers do not understand context and our instructions must be exact. If not, the computer responds with an error message and we show students how to evaluate the traceback procedure to where the code broke so it can be fixed.
This process ensures that students learn how to become autodidactic learners. We teach students how to become self-aware of their abilities to build code scripts, sleuth error messages, identify and correct logic gaps in writing and presentation, learn to interpret visualizations and results, and how to fail in a constructive manner. This teaches them to emulate and improve upon these problem-solving processes when we are not there to coach them. We remove the math phobia and fear of algorithms and instead teach that these computational methods are for everyone - not just for those who are interested in STEM or computer science - and that humanists and artists can also access the most powerful scientific tools to help answer important questions. Students also learn to use various online resources, such as help pages and walkthroughs for topics such as data visualization, machine learning, and natural language processing (e.g., Hunter 2007, Pedregosa et al. 2011, Honnibal and Johnson 2015), and online forums and spaces to ask questions, such as StackOverflow (“Stack Overflow”). Students can then bring these skills to explore more complex topics in the elective courses.
Digital Humanities and Archival Design
DIGHUM 150A combines theoretical and practical training to design, construct, and curate archives as websites. Students first learn the basics of archival design and different means for archival curation. We teach students how to mediate the relationship between knowledge classification systems and the audience’s capacity for interacting with memorable mental models (i.e visualizations and narratives). This includes the digitization and curation of these archives into online open data sets using creative commons licences (e.g., CC-BY).
Largely due to ongoing efforts to digitize textual manuscripts, text in digital format, whether they are structured files, PDF, TXT, CSV, or semi-structured files, JSON, XML or HTML, is becoming progressively more ubiquitous in virtually every field of research and captures human existence in ancient and modern forms alike. From the Perseus Project to Wikipedia, digital text is becoming sufficiently large enough for computers to ‘leverage’ and ‘learn’ in order to translate between the multitude of languages and ontologies we use to describe our different worldviews. Big data exists not only in terms of size, but also in complexity as digital archives have become more accessible, and are increasingly used in building contextual, quantitative, qualitative inferential, and predictive research via unsupervised, semi-supervised, and supervised models (Gentzkow, Kelly, and Taddy 2019). Within DH at Berkeley, the content students engage with foregrounds world heritage in its many forms - cultural, linguistic, textual, verbal, musical, and architectural - all of which are artifacts embedded in rich datasets, many of which are being lost in analog format. We teach that arts and humanities scholars have a moral obligation to work toward the digital preservation and conservation of these artifacts and enable a wide community to participate in these practices using open-source tools and methods when possible.
Lectures cover not only the theoretical foundations of archival practices, but also provide a comprehensive survey of the different website platforms available for the students to design their own archive. Assignments in the course build progressively to assist each student in learning digital curation
and enable the description of their archives in website, poster and video form. The results of each student are shared openly during the course for peer review, which promotes more intuitive group collaboration, and their outcomes are largely determined by their own interests and abilities in web design. For example, Sharon Hui built a website, “Statistics in Pokémon” (Hui 2020), which combined her knowledge of statistics with her love of Pokémon. Her archive shows the development of the Pokémon games through time and features examples of data analysis with applied statistics for the Pokémon datasets she assembled (“Hui Blueprints 2020”, Hui Video 2020
).
Textual and Language Analysis
DIGHUM 150C teaches application of natural language processing techniques in Python to problems of literary studies. Students close-read a Victorian novel and two collections of modernist short stories and apply these methods to literary-critical questions encountered in the readings. They use principal component analysis from Python’s scikit-learn library for machine learning to investigate word counts of the narrators of Wilkie Collins’s novel The Moonstone and create a stylometric (literary linguistic style) comparison of their narrative modes to determine affinities between their styles. Later, they categorize the vocabularies of Katherine Mansfield’s stories using WordNet (“WordNet”) and the Natural Language Toolkit (NLTK; Bird et al. 2009) to produce an overview of her stories’ thematic distributions. Students’ final projects resemble traditional seminar papers for a college English course, except that they are supplemented with computational text analyses to support their literary-critical arguments.
In this course, Siyi Wu’s research showed the ratio of female-to-male gendered pronouns in The Moonstone according to the narrator of the given section (Figure 1). The only female character, Miss Clack, is shown to use the most female pronouns even though this ratio remains below 1.0. Research by Flaviano Christian Reyes shows the distribution of color words in Katherine Mansfield’s highly colorful modernist short story, “The Garden Party” (Figure 2).
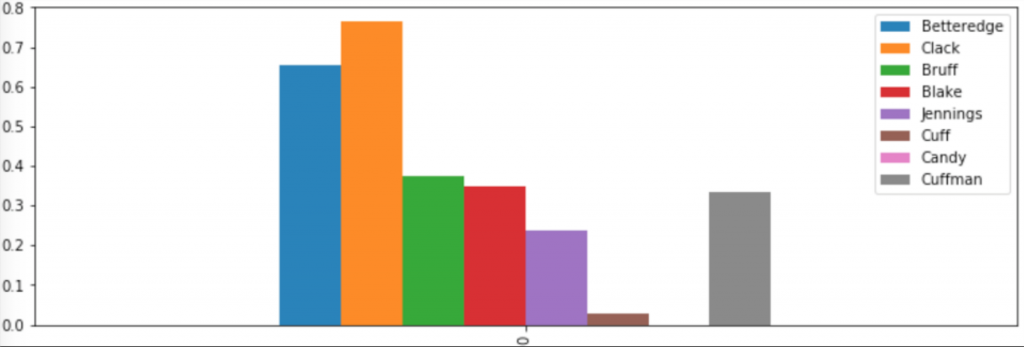
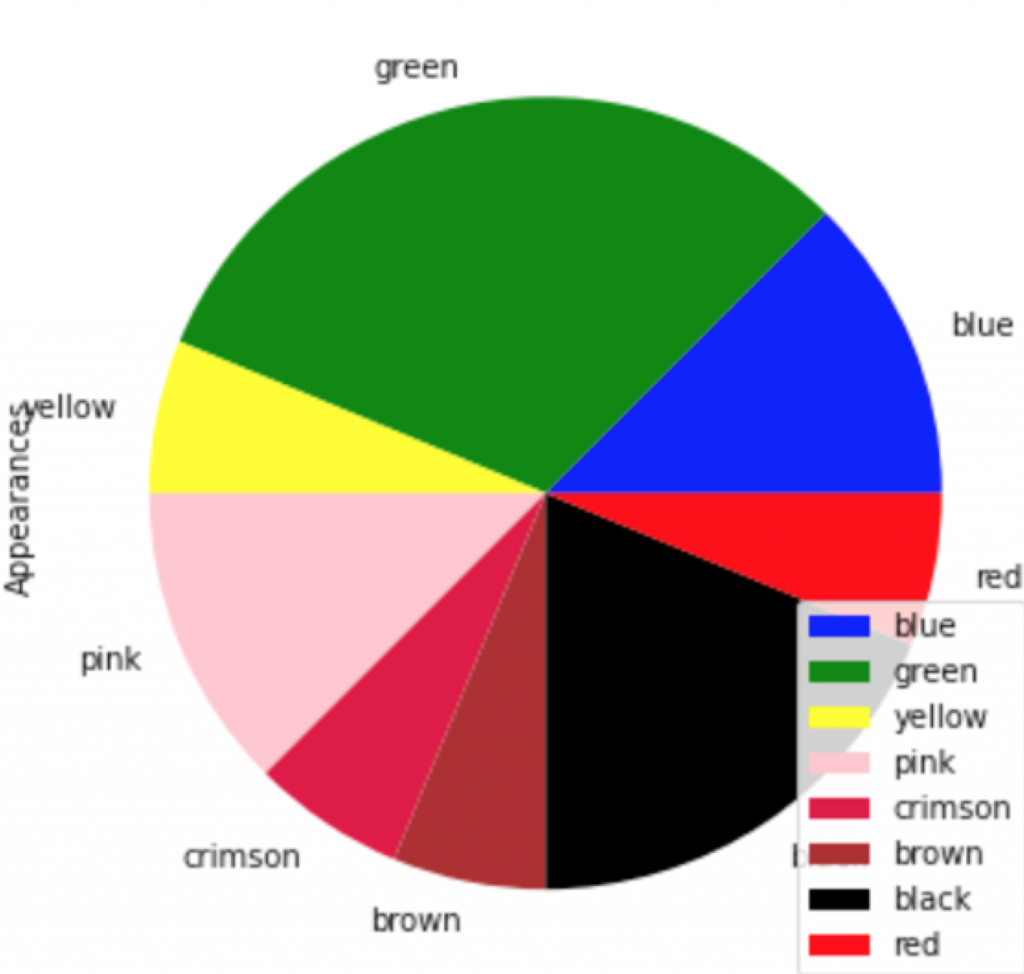
Through a dialectical approach (logical discussions and debate) to these two primary methods, students find new ways of understanding literary texts which feed into each other: computational text analysis leads to further close reading, which in turn leads to further computational study, and so on. Students from this course have gone on to graduate study at Columbia University, and the University of Washington, in disciplines such as data science and educational technology.
Critical Digital Humanities
DIGHUM 160 aims to bring the hermeneutic tradition in humanities - the study and methods of interpretation - together with the quantified approaches offered by DH. In the course, students use politically charged and controversial datasets from news aggregation website Reddit. They confront hermeneutics with computational methods from textual and data analysis to explore the discursive ways in which facts and views are drafted and vetted and the kind of linguistic strategies that community members on platforms like Reddit employ to convince or refute each other.
We provide an overview of different humanist theories of interpretation developed in the past century, ranging from focus on defamiliarization by the Russian Formalists to the poststructural emphasis on différance for the purpose of pragmatic understanding when interpreting big data. Deploying hermeneutics to read online discourses means, in some ways, to read them as though they were literary. Students begin by reading posts and comments generously and rigorously and learn to avoid the pitfalls of authorial or affective fallacies; neither authorial intent nor the emotional effects on a reader are central to understanding a subreddit’s discursive structure. Through close reading and annotating, students try to understand the heterogeneity of the data that will later be viewed from a distance and attain an overview of a community’s dominant themes and concerns via language modeling methods such as word embeddings and topic modeling. This results in a vacillation between close and distant reading and between the culturally, ideologically, and contextually familiar/unfamiliar. Reading Reddit hermeneutically means reflecting on two orders of interpretation: community members negotiate the space of the permissible, and students - as researchers - approach these discursive norms with their own preconceptions and intents. Students move between close and distant reading to imply identifying procedures to select samples of social data.
In her digital essay for the course, Teresa Goertz explored the r/changemyview Reddit community. Here, contributors post their thoughts and have other users change their mind, provided arguments of the commenters are convincing to them. Discourse on this community blurs not only the lines between expert and personal experience but also between facts and opinions. As r/changemyview makes use of tags that help users filter specific types of posts, the student filtered out submissions marked with the flair “Delta(s) from OP”, which indicates that the OP (meaning “original poster” - the person who originally posted the submission) awarded one or several so-called “Delta(s)” to a commenter who convinced the OP to change their view (Figure 3). She used TF-IDF and topic modeling to locate a particular kind of “convincing argument” to guide her selection of materials to close-read and analyze interpretively.
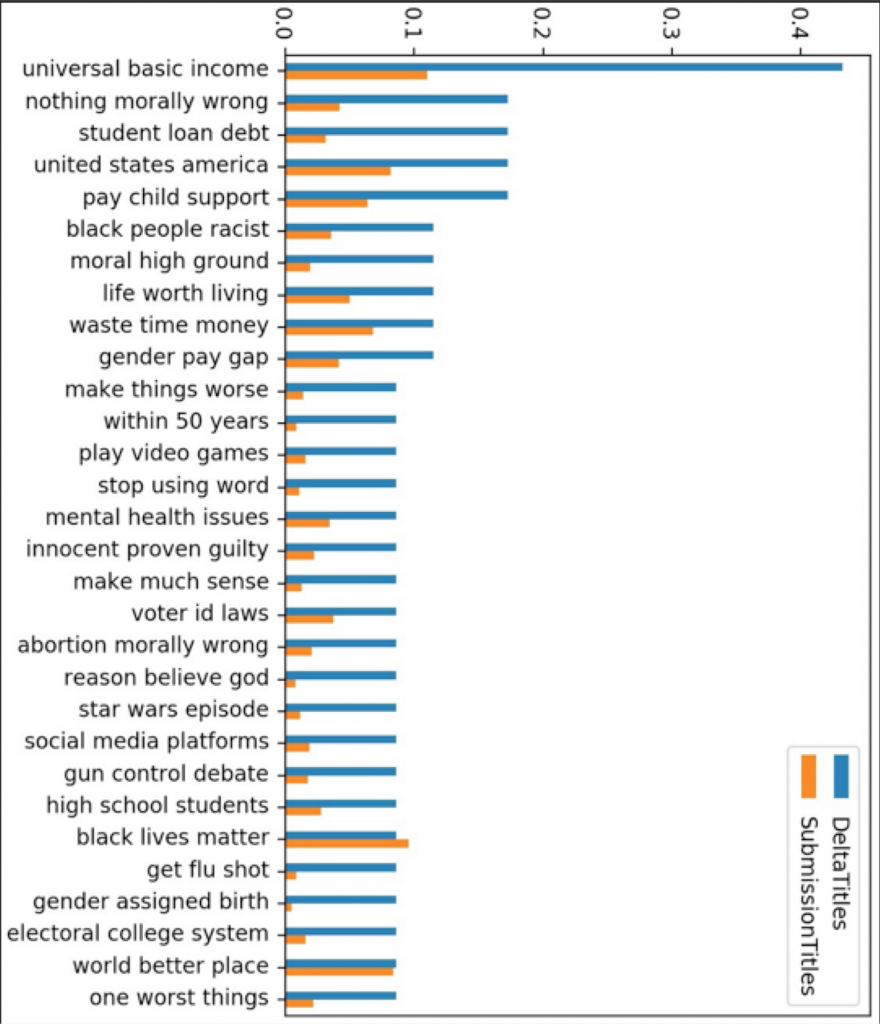
Eunice Chan also looked at r/AmItheAsshole, a community on Reddit formalizing the moral evaluation of people’s social decisions. Users can post the details of a difficult or uncomfortable social situation they were in while the community tries to come to a decision about that user’s role. For eighteen hours, the community can respond to a post with a judgement: YTA (You’re The Asshole) or NTA (Not The Asshole). In her essay, Eunice investigated whether the appearance of particular offensive terms and topics were linked to the outcome (Figure 4).
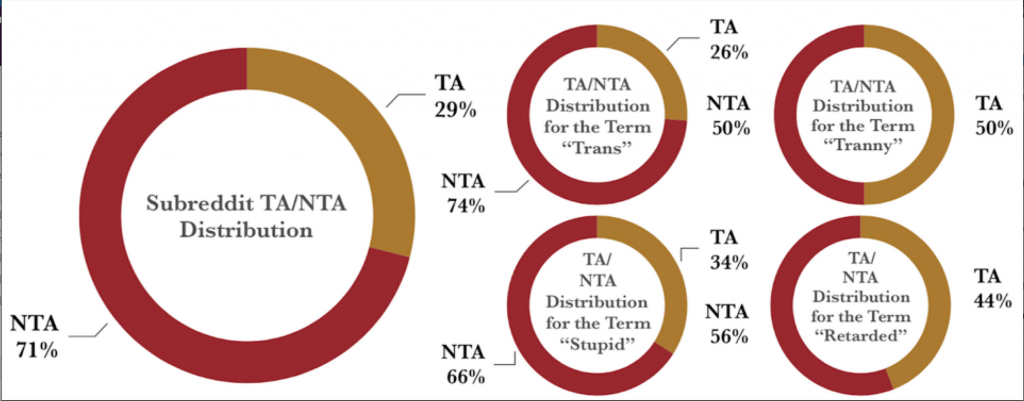
In sum, whether students conduct their research and construct their products through data assembly in the archives course, analyze words in the literary and text analysis course, or discourse analysis in their critical hermeneutics course, students learn to engage in the process of critical analysis using the application of data science tools to humanities topics. Through basic understandings of literary form and practice students begin to understand biases and mechanics of the digital world around them as theoretical and computational components merge.
Building Creative Minds
Students develop key habits of mind for creative and inventive interdisciplinary scholarship:
- Domain competence: the ability to start down the path of becoming an expert in a particular field, and to be able to extrapolate skills learned in this field to others
- Patience and repetition: analogies of a painter practicing the same stroke patterns or a dancer performing the same sequence of moves thousands of times
- Active learning: learning by doing, exploring, experimenting, failing, and trying again
- Apprenticeship, scaffolding of information, and coaching: teachers who think aloud through the coding process and their mistakes to teach each other and students to do the same
We also emphasize interpersonal skills for collaboration and teaching. Kelley and Kelley (2013) believe that humans are naturally creative but require highly personalized outlets and guidance to be successful. Creativity is coachable if it is human-centered and rooted in conscious intention because empathy “makes our observations powerful sources of information” (21). Empathy is defined as “a vicarious, spontaneous sharing of affect, [that] can be provoked by witnessing another's emotional state, by hearing about another's condition, or even by reading.” (Keen 2006 208). We all come from different life experiences, learning backgrounds, and technical competencies and these courses emphasize the need for students to step out of their comfort zones and learn about the experiences of others to better understand their own. We believe that in STEAM contexts, digital tools help foster compassion and social responsibility - not fragmentation and individuation. Empathy teaches learners the importance of building emotional and psychological bonds (Preston and de Waal 2002).
Through confidence training, we teach students that they can change the world through new approaches and solutions based on creative exploration and expression of themselves. This requires creators to tap into their dualistic artistic/scientific selves and to challenge previously held belief systems and goals by changing how we see the world and ourselves. Students try something, fail, and learn from that failure so that repetition of this process sharpens their creativity and scientific sense of self. Constructive failure is when we help a student flip their mindset from a fixed one (“I cannot figure this out because this is all I know”) to a growth one (“Even though I do not know this, I can figure this out because I can use my previous knowledge of old problems and apply it to solving this new one”). We also use urgent optimism (Kelley and Kelley, 2013) to encourage students to take a bias towards action and experimentation and to sharpen their intuition to more efficiently discern important concepts, features, questions, issues, problems, and needs. This helps keep them engaged in their projects.
Students begin to relearn what they have been taught to think about themselves and their potential by learning to differentiate between “divergent” (exploration of multiple, unusual, and original solutions to a problem) and “convergent” (finding the “right” solution to a problem, such as in posing positivist research questions) thinking (Baer 1997). Creative cognition and development entail a combination of divergent and convergent thinking. One needs a divergent attitude to generate ideas from an initial prompt, but also needs a convergent-integrative approach to synthesize these elements (Van de Ven 2019) and offer closure to the response. These concerns are relevant to DH, where they can help navigate the debates between adapters of computational methods and defenders of “traditional” humanist approaches. STEAM research training is as much about development of ideas as it is about producing empirical evidence for those ideas to help clarify that research and methodological problem-solving skills promoted by STEM cannot be formulated without imagination and creativity (something that arguably drives all scientific disciplines). Elliot Eisner’s (2003) ideas about formation of the mind and the complex processes that require the cultivation of visualization and imaginative storytelling exemplify the distinction between the brain and the cultivation of mind:
That premise is that humans do not come into the world with minds; they come into the world with brains. Minds are forms of cultural achievement. Brains are biological resources. The task of education, socialization, and acculturation is to transform brains into minds. Minds come into existence as individuals secure varied forms of experience in the course of their lives and, through those forms of experience, learn to think. In schools, that experience is shaped by many factors; two of the most important are curriculum and teaching practices. Curriculum and teaching are at the heart of the process of creating minds, and it is in this sense that the curriculum and the teaching that mediates it are mind-altering devices (Eisner 1994). What we decide to include in our curricular agenda and how we choose to teach it have a profound effect on how students learn to think and what they are able to think about. (341)
We do not make all students of the same mind and instead help each student grow into their own creative and scientific minds to think past parroted talking points and realize that everyone is different and that there truly is a lot wrong with the world beyond our own perspectives.
Implications for e-lit
Berkeley has long assessed the dominant ideas and power structures through critically reading the texts produced and in that culture (“The Berkeley Revolution”). DH at Berkeley and D-Lab bring the same attentive analyses to the ever-widening scope of available digital texts. Our efforts involve different theorizations of and experimentations on literary and colloquial texts and have implications for the understanding of e-lit, including textuality, preservation, software, and code. Instructor training, student creativity, and the exploration of large text corpora using data science methods within DH critical theory perspectives produces many opportunities for the production of e-lit. In DIGHUM100 and 101 for example, Matthew Mason used a reproducible Python Jupyter Notebook and computational text analysis to study Renaissance Macaronic poetry. He created descriptions and interpretations of poetry based on quantitative language models and generated a series of questions that combined statistical assessments of fit and representativeness of the data within this genre of poetry (Mason 2020).
Digitization means more and more text is read as digital text, and an increasing amount of methods, as performative engagements, can be brought to bear on their digital materiality.
Espen Aarseth (1997) has used the concept of “ergodic literature” to highlight how mechanically organized texts (such as video games) are characterized by the “nontrivial effort [that] is required to allow the reader to traverse the text” (1). This also means that, with increasing options for digital traversal and transformation, reading texts becomes an interactive and collaborative endeavor. For example, Andres Duarte’s DIGHUM 100 study described the burden each student faces when mitigating the scale of a digital corpus such as Project Gutenberg for all potential relations between these sources (Duarte 2020).
One of the ways in which our program allows for new pedagogical strategies to emerge has to do with what Andrew Piper called “social reading” (Piper 2012), or having the permission rights and ability to see what others are reading, to transfer readings to others, and to discuss these readings with others. A combination of social reading and “social annotation” (writing in the textual margins) is crucial for developing both close and distant reading skills. While traditions of annotation predate the birth of print (Jackson 2001 5), we are interested in the real-time and collaborative nature of open, neutral, and lasting annotating that web applications can afford to explore “writing in the margins” as a pedagogy of integrative annotation. DIGHUM 150C and 160 both use hypothes.is (“Hypothes.is”), an online tool that students use to annotate their reading. They are taught to create concise, well-written, observant, and precise annotations, which are viewable and can be responded to by their classmates. Hypothesis allows for a conversation layer anywhere on the web and enables students to annotate literature sources (such as the 19th-century British novel The Moonstone, taught in Textual and Language Analysis (DIGHUM 150C) and social data (such as the Reddit community interactions in DIGHUM 160).
We view the fact that these forms of data differ sharply - one being a relatively linear narrative, the other a database of fragmented conversations (Manovich 1999) - as inviting creative and compounded forms of textual and analytic engagement. Students who take both courses can transfer reading strategies from one domain to the other. By looking at social media community members’ rhetorical strategies, students can brainstorm ideas about how an author presents strategies in their novel. By following a conversation in a novel, they might gain insights into how an online conversation thread is developing. As their annotations are globally visible, the approaches they develop can be followed, and followed up on, by different instructors.
DH might be characterized by a “fundamental mismatch between the statistical tools that are used and the objects to which they are applied”, as Nan Da recently posited (Da 2019 601). InDIGHUM 100, Marc Vasquez realized that this mismatch has created deep divisions between analytical disciplines, such as between quantitative or qualitative methods (Vasquez 2018). In some ways, questions that rely on the quantification of text (the statistical reduction of its inherent plurality) are inherently short-sighted. We think it is always a highly valuable kind of failure however, one that not only offers rich new insights into questions about literariness, style, discourse,race, gender, etc., but that also highlights processes by which students in the arts and sciences can enrich each other’s perspectives. A STEAM approach incorporates spaces and practice-based forms of pedagogy where scientific and humanistic research and digital storytelling coexist, where new collaborations between technologists and authors can manifest, and that can produce innovations in pedagogy, analytics, and (computational) literature.
Conclusions
We teach students to combine artistic creativity and computational tools in interdisciplinary ways and promote environments where they are encouraged to follow research interests and explore ideas, topics, and worldly problems that are meaningful to them. A humanistic data scientist is the new renaissance person, one who explores their humanist research pursuits using modern computational tools, techniques, and methods and who is constantly learning and growing and broadening their repertoire for understanding and engaging with the world. This does not imply that close reading is ignored or forgotten, but that it is reevaluated within contexts of other lines of questioning afforded by digital tools. Grappling with the world means engaging with it through the senses and imagination and dreaming of the wildest possibilities while deconstructing the dystopian reality we are living. As both the global COVID-19 pandemic and police terrorism rage on, we uniquely prepare STEAM scholars to combat this new “normal” era of propaganda, disinformation, false equivalencies, and cyber insecurity not only because they learn the technical competencies to study these topics for themselves, but specifically because they have the strong arts and humanities background to situate their computational expertise within new domains of false discourse. Students learn to access constant social media information feeds along with newspaper archives, geospatial maps, novels, textbooks, technical manuals, video, audio, and other media to investigate these topics.
Critical theory provides a way to frame reality from multiple perspectives beyond reductionist and positivist approaches. We teach pluralism and teach students multiple critical theoretical perspectives to improve cultural analytic research and creative production of various forms. We help students unmask and demystify underlying complex systems to reduce the number of blind spots and introduce students to a different way of human-centric learning and knowing. STEAM scholars must be critical about deciphering the constructs that comprise our lives and use empathy and human connection to avoid overlooking sensorial inputs and emotion. Empathy is not owned by the arts and humanities or STEM but is critical to the success of both. Overemphasis of technical coherence might also overemphasize the quality of the learning experience if we view education as a space for self-creation (Eisner, 2002). Thus, a grounding in critical theory is necessary to understand both the research process and empathy alike.
Ultimately, research topics are conceptualized by the students and, with guidance from the instructors, are seen through to completion according to the terms, competencies, and goals of each student. Our spaces provide an example of a combined Digital Humanities/Data Science lab that not only enables interdisciplinary practices and incubates new ideas and forms, but that also benefits emerging disciplines such as e-lit that extend humanities research using digital methods.
Acknowledgments
Alex Saum-Pascual, Scott Rettberg, Alexander Sahn, Jae Yeon Kim, and Aniket Kesari provided comments that improved the quality of this chapter. We also thank the students whose work was featured here. We are grateful to the Creative DH Frameworks Working Group.
Works Cited
Aarseth, Espen J. Cybertext: Perspectives on Ergodic Literature. Johns Hopkins University Press, 1997. Accessed 7 July 2020.
Akazawa, Chloe. “Individual and Group Final Projects - DIGHUM 101.” GitHub Repository, 2020,
github.com/chloeaka/Digital-Humanities-Project. Accessed 1 Sept. 2020.
“Akazawa Video 2020.” Akazawa, Chloe. Google Drive, 2020, drive.google.com/file/d/1Nn3rEaQyZsBxCFoVYJlSOCjrnj2jkS2E/view. Accessed 1 Sept. 2020.
“Art Up Close”. Akazawa, Chloe, 2020, artupclose.wordpress.com/. Accessed 1 Sept. 2020.
“Art Up Close Blueprint 2020.” Akazawa, Chloe. Google Drive, 2020, https://drive.google.com/file/d/1gUaGubLdqVp-J9ZGzQ2l9En5MwdSPKz2/view. Accessed 1 Sept. 2020.
“Berkeley News.” Public Affairs, UC Berkeley, Berkeley Talks: We Need a Digital Infrastructure That Serves Humanity, Says Techno-Sociologist, 2019, news.berkeley.edu/2019/08/26/berkeley-talks-zeynep-tufekci/. Accessed 7 Sept. 2020.
Baer, John. Creative Teachers, Creative Students. Allyn and Bacon, 1997.
“Bash (Unix Shell).” Wikipedia, Wikimedia Foundation, Accessed 12 Sept. 2020, en.wikipedia.org/wiki/Bash(Unixshell). Accessed 1 Sept. 2020.
“BAMPFA.” Berkeley Art Museum and Pacific Film Archive, 2020, bampfa.org/. Accessed 8 Sept. 2020.
“Binder.” The Binder Project. mybinder.org/. Accessed 1 Sept. 2020.
Bird, Steven, Edward Loper and Klein Ewan. Natural Language Processing with Python. O’Reilly Media Inc, 2009. Accessed 7 July. 2020.
Chan, Eunice. “Lyrics Through Time.” Omeka RSS, 2020, lyricsthroughtime.omeka.net/and Google Drive https://docs.google.com/presentation/d/1LLISzsrhe-RgUtbryzktosnq2jYk3RV4mty9BUoa6wY/edit#slide=id.p. Accessed 1 Sept. 2020.
Cheng, Jeffrey. A Modern Analytical Comparison of Biblical Canon and Apocrypha. Google Drive, 2020, drive.google.com/file/d/10vSVKshT65CyqX-7ZSQE_2sFETvTlQ3D/view?usp=sharing. Accessed 1 Sept. 2020.
Chollet, François. “Keras.” Keras - Deep Learning for Humans, GitHub Repository, 2020, github.com/keras-team/keras. Accessed 8 Sept 2020.
“Courses.” UC Berkeley Digital Humanities Summer Minor and Certificate Program, 2020, summerdigitalhumanities.berkeley.edu/courses. Accessed 1 Sept. 2020.
Da, Nan Z. “The Computational Case against Computational Literary Studies.” Critical Inquiry, vol. 45, no. 3, 2019, pp. 601–639., doi:10.1086/702594. Accessed 7 July 2020.
“DH at Berkeley.” Digital Humanities at Berkeley, 2020, dh.berkeley.edu/. Accessed 7 Aug 2020.
“D-Lab.” UC Berkeley D-Lab, 2020, dlab.berkeley.edu/. Accessed 7 Aug 2020.
“DH at Berkeley - e-lit.” 2020. Retrieved September 26, 2020, from https://digitalhumanities.berkeley.edu/tags/electronic-literature
“DH Fair 2020: Online.” Digital Humanities at Berkeley, 2020, dh.berkeley.edu/dh-fair-2020-online. Accessed 7 Aug 2020.
“DH Fair 2020: Posters.” Digital Humanities at Berkeley, 2020, dh.berkeley.edu/dh-fair-2020-posters. Accessed 7 Aug 2020.
Duarte, Andrew. “A Meta Analysis of The Project Gutenberg.” Google Drive, 2020, drive.google.com/file/d/1C1B0aC3kqMEphs9Bn5-tOGCE2Jr1WCYE/view. Accessed 1 Sept. 2020.
Eisner, Elliot W. The Arts and the Creation of Mind.Yale University Press, 2002. Accessed 7 July 2020. Accessed 7 July 2020.
Eisner, Elliot W. “The Arts and the Creation of Mind.” Language Arts, vol. 80, no. 5, 2003, pp. 340-344. Accessed 7 July 2020.
Gentzkow, Matthew, et al. “Text as Data.” Journal of Economic Literature, vol. 57, no. 3, 2019, pp. 535–574, doi:10.1257/jel.20181020. Accessed 1 Sept. 2020.
“Gephi.” The Open Graph Viz Platform. Graph Exploration and Manipulation, 2020, gephi.org/. Accessed 7 July 2020.
“GitHub.” GitHub - Build Software Better, Together, 2020, github.com/. Accessed 7 July 2020.
“Gui and Guai.” Goertz, Teresa, 2020, guiguai.omeka.net/exhibits. Accessed 1 Sept. 2020.
Goertz, Teresa. Gui and Guai: Exploring the Strange in 18th c. China. Google Drive, 2019,
drive.google.com/file/d/1Bxp91uvkGljRjUekawUPp6bnsAkj6isD/view. Accessed 1 Sept. 2020.
Honnibal, Matthew and Johnson, Mark. “spaCy: An Improved Transition System for Dependency Parsing”. Proceedings of the 2015 Conference on Empirical Methods in Natural Language Processing in Lisbon, Portugal. Association for Computational Linguistics, 2015, pp. 1373-1378. https://www.aclweb.org/anthology/D15-1162/. Accessed 7 July 2020. Accessed 7 July 2020.
Hui, Sharon. Statistics and Data in Pokémon. GitHub Repository, 2020, swhui.github.io/StatisticsinPokemon/. Accessed 1 Sept. 2020.
“Hui Blueprints 2020”. Hui, Sharon. Google Drive, 2020, drive.google.com/file/d/1L3HgWP7rJ4gNvuLmVYYzZJRa3BAtToSU/view. Accessed 1 Sept. 2020.
“Hui Video 2020”. Hui, Sharon. Google Drive, 2020,drive.google.com/file/d/1Zbvq9PELwjkhKoOgWDdnXIZRIb105h4k/view. Accessed 1 Sept. 2020.
Hunter, John D. “Matplotlib: A 2D Graphics Environment.” Computing in Science & Engineering, vol. 9, no. 3, 2007, pp. 90–95, doi:10.1109/mcse.2007.55. Accessed 7 July 2020.
“Hypothesis.” Web Annotation Project. 20 July 2020, hypothes.is/. Accessed 7 July 2020.
Kasmir, Shereen. “What Is Happiness?” Google Drive, 2020, drive.google.com/file/d/1vOHacQf9r77y3ynhDH0vkMZXVh2Hpe9l/view?usp=sharing. Accessed 1 Sept. 2020. Accessed 7 September 2020.
Keen, Suzanne. “A Theory of Narrative Empathy.” Narrative, vol. 14, no. 3, 2006, pp. 207–236.
JSTOR, www.jstor.org/stable/20107388. Accessed 17 Sept. 2020. Accessed 7 July 2020.
Ku, Bonmu. “Individual Final Project - DIGHUM 101.” GitHub Repository, 2020,
github.com/jubenmao/dighum. Accessed 1 Sept. 2020.
Jackson, H. J. Marginalia: Readers Writing in Books. Yale University Press, 2001. Accessed 7 July 2020.
Jiang, Sarah. “Individual and Group Final Projects - DIGHUM 101.” GitHub Repository, 2020,
github.com/sjiang9168/DIGHUM101-Individual-Project. Accessed 1 Sept. 2020.
Kelley, Tom and Kelley, David. Creative Confidence: Unleashing the Creative Potential within Us All. Crown Publishing, 2013. Accessed 2 July 2020.
Manovich, Lev. “Database as Symbolic Form.” Convergence: The International Journal of Research into New Media Technologies, vol. 5, no. 2, 1999, pp. 80–99, doi:10.1177/135485659900500206. Accessed 3 Sept. 2020.
Mason, Matthew. Why Use Computational Text Analysis to Study Renaissance Macaronic Poetry?
Google Drive, 2020, drive.google.com/file/d/1U-Dqv5h-RL8n_oCmeDDH1-YMFaJvGqQV/view. Accessed 1 Sept. 2020.
“Measuring Hate Speech.” UC Berkeley Measuring Hate Speech Project, 11 Oct. 2019, hatespeech.berkeley.edu/. Accessed 8 Sept. 2020.
Oh, Kenny. Brueghel Family Archival Methods: Practices of Computational Viewing.Google Drive, 2020,
drive.google.com/file/d/1ZhIaE7qD0mdcVUs8EtBxJDBtHWQCjw3J/view. Accessed 3 Sept. 2020.
Parekh, Rishabh. “Individual Final Project - DIGHUM 101.” GitHub Repository, 2020,
github.com/rishabh-parekh/dh101final. Accessed 1 Sept. 2020.
Pedregosa, F, et al. “Scikit-learn: Machine Learning in Python.” Journal of Machine Learning Research, vol 12, 2011, pp.2825-2830. Accessed 1 July 2020.
Piper, Andrew. Book Was There: Reading in Electronic Times.University of Chicago Press, 2012. Accessed 10 March. 2020.
Preston, Stephanie D., and Frans B. M. de Waal. “Empathy: Its Ultimate and Proximate Bases.” Behavioral and Brain Sciences, vol. 25, no. 1, 2002, pp. 1–20., doi:10.1017/s0140525x02000018. Accessed 17 Aug. 2020.
“Projects.” Digital Humanities at Berkeley, 2020, digitalhumanities.berkeley.edu/projects. Accessed 7 Sept. 2020.
“Python Jupyter Notebooks.” Python Anaconda Distribution with Jupyter Notebooks, 2020, www.anaconda.com/products/individual. Accessed 7 Sept. 2020.
Saum-Pascual, Alex. No Legacy || Literatura Electrónica. 31 Jan. 2018, http://www.alexsaum.com/no-legacy-literatura-electronica/. Accessed 1 Sept. 2020.
“Stack Overflow.” Where Developers Learn, Share, & Build Careers. 2020, stackoverflow.com/. Accessed 7 September 2020.
Subramanian, Anusha. “Individual Final Project - DIGHUM 101.” GitHub Repository, 2020, github.com/anusha0712/Sex-Ed-Survey. Accessed 1 Sept. 2020.
“The Berkeley Revolution.” A Digital Archive of the East Bay's Transformation in the Late-1960s & 1970s, revolution.berkeley.edu/. Accessed 7 June. 2020.
“UC Berkeley Division of Computing, Data Science, and Society.” 2019, data.berkeley.edu/. Accessed 1 Sept. 2020.
“V-Lab.” UC Berkeley History of Art Department - Visualization Lab for Digital Art History, 14 July 2020, arthistory.berkeley.edu/resources/visual-resources-center/. Accessed 12 Aug 2020.
van de Ven, Inge. “Creative Reading in the Information Age: Paradoxes of Close and Distant Reading.”
Journal of Creative Behavior, vol. 53, no. 2, 2019, pp. 156–64, doi:10.1002/jocb.186.
Vasquez, Marc. “Citing the New Critics: A Citation Network of Seminal New Critical Essays.” Google Drive, 2020, drive.google.com/file/d/1UUDEobzJJN6TH4D2lvpekjozmHh6F8/view. Accessed 1 Sept. 2020.
Vihavainen, Arto, et al. “Extreme Apprenticeship Method in Teaching Programming for Beginners.”
Proceedings of the 42nd ACM Technical Symposium on Computer Science Education - SIGCSE '11, 2011, doi:10.1145/1953163.1953196. Accessed 7 July 2020.
Vygotsky, L.S. Mind in Society: The Development of Higher Psychological Processes. Harvard University Press. 1978. https://www.jstor.org/stable/j.ctvjf9vz4. Accessed 7 July 2020.
“WikiArt.” WikiArt - Visual Art Encyclopedia, 2020, www.wikiart.org/. Accessed 1 Aug 2020.
“WordNet.” Princeton University. 2010. wordnet.princeton.edu/. Accessed 1 Sept 2020.
“zhon.” zhon PyPI Python Library. pypi.org/project/zhon/. Accessed 1 Aug 2020.