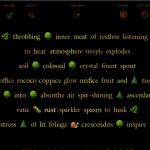
Literary forms seen as tools of mind transgressing the field of the literary and repurposing digital media so that they are capable of refocusing cognition in beneficial ways – these are the lines of thought shaping Kyle Booten’s analysis of algorithmic co-writers. To be able to respond to both challenges, it is proposed that researchers rigorously and systemically study how digital tools are being creatively used and repurposed, learning from models that have emerged within the mainstream Human-Computer Interaction research.
Care and Carelessness
As the writer types furiously, attempting to meet a deadline, the little red squiggle appears. The writer’s eyes dart to the left. The word is “aquire,” but Microsoft Word suggests it should be “acquire.” The writer accepts this change and moves on.
A few sentences later, another red line appears. When the writer hovers their cursor above the words marked by this line – “It goes without saying” – a popup message warns that this way of starting a sentence suggests a lack of confidence. This message comes from Grammarly, an algorithmic writing assistant.
These days, algorithms insinuate themselves into our writing processes in ways that are increasingly complex, both on a technical level (Grammarly employs cutting-edge machine learning) and on a conceptual level (it purports to assist with subjective matters such as tone and style). The issue of spell-checkers and automatic writing assistants may seem relatively low-stakes compared to other ways that algorithmic media intervene in human lives, from facial-recognition software used for policing to statistical algorithms that determine credit scores. However, the phenomenon of human-algorithm co-writing connects to larger debates about the ways that digital media are often detrimental to our minds. As philosopher of media Bernard Stiegler has argued by way of Marx, digital technology is dangerous because it threatens to “proletarianize” us in new ways, making automatic and external to us the very mechanisms of thought and thus alienating us from our own capacity to think for ourselves (Automatic Society). Critiquing what he calls the “linguistic capitalism” represented by Google’s search technologies, Frédéric Kaplan has argued that this company’s autocomplete feature nudges human writers toward certain search terms that are valuable to Google – and thus away from any kind of linguistic idiosyncrasy.1Stiegler’s argument indeed draws upon Kaplan’s. The humble spell-checker, long blamed for making us worse at spelling, has presaged the more ambitious ways that writing-assistance algorithms may strip us of our creative capacities and homogenize our minds on behalf of technocapital.
On the other hand, we may more sanguinely see in certain algorithmic tools, perhaps including both the spell-checker and Grammarly’s stylistic suggestions, something that is often missing from contemporary digital media: care. As Stiegler has also argued, digital media companies generally operate in a “care-less” manner – for instance, by building sophisticated models of our minds only to recklessly capture our attention for purely economic purposes (Taking Care). It is not hard to find examples of the malady Stiegler diagnoses. Netflix’s recommendation algorithm shows you what it thinks you will watch2See https://help.netflix.com/en/node/100639., not (as a teacher or friend might) what you should watch. Grammarly, at least, is explicitly normative. It draws the writer’s attention to certain phrasings in order to suggest that these words should be revised.
The question of what it means to write with an algorithm is a significant one that connects to broader questions about how human thought could and should be mediated by algorithmic logic and about how algorithms should care for and about us.3My mention of “mediation” is with reference to Vygotsky’s concept of the tool- and sign-mediated aspects of human thought. While there are many types of care (the care of a doctor for a patient, of a collector for a rare object), here I use the term “care” in a way that is closer to the kind of care enacted by teachers – perhaps especially teachers of literature, composition, and related fields – for their students. Such pedagogical care challenges the student to develop in their use of language. Behind such care is often some normative sense of how the student’s mind should work, their words should flow.
Mainstream corporate technologies such as Microsoft Word’s spell-check and Google’s autocomplete seem hegemonic. Tinkering in the shadows cast by such software, however, practitioners of electronic literature have long experimented with developing alternative interfaces and, more generally, techniques of writing with algorithms that are both speculative and critical (see Emerson). The goal of this paper is to briefly connect three recent instances of co-writing tools that are also examples of electronic literature in order to consider how each tool exemplifies a different way of tending to – caring for – the minds of its users.
Rnn-writer’s Power of Suggestion
The novelist Robin Sloan’s rnn-writer (2016) makes use of a “recurrent neural network” (“RNN”) to offer autocomplete-like suggestions for creative writing. Given some input text (such as “There once wa”) the neural network will predict, based on a large corpus of “training data,” the next most likely character (for instance, “s”); performing this prediction step over and over generates text letter by letter. With rnn-writer, Sloan has inserted this neural network into a typical text-input interface. By pressing a special key, the user can prompt the network to continue the text on the screen. Hitting “down” will cycle through other suggestions. Sloan has trained rnn-writer on a collection of science fiction stories, so its suggestions are (at least in theory) tailored for human writers who are composing in this particular genre.
Rnn-writer both evokes and departs from corporate uses of neural-network-generated autocompletions. Recently Google has integrated something like autocomplete into its Gmail platform; going beyond “predictive text” algorithms that merely suggest the user’s next word, “Smart Compose” predicts entire phrases, empowering users to compose emails “from scratch, faster” (“SUBJECT”). What Sloan sees valuable in rnn-writer’s suggestions is not that they make writing quicker or easier (by filling in what the human writer would perhaps have written anyway) but that they “make it harder” (“Writing,” robinsloan.com). Composing with rnn-writer, he suggests, is like “writing with a deranged but very well read parrot on your shoulder.” In a video that captures his process of writing with rnn-writer in front of an audience at the 2017 Eyeo Festival (“Writing”), he begins a story:
The sun rose over the planets’s [sic] bleak surface. Captain Eyeo watched as
Sloan then prompts the system to produce a continuation of this sentence, cycling through several options until settling on this one:
...as the control board clicked open.
Here the network’s suggestion continues the sentence grammatically while fluently evoking a familiar motif of science fiction, yet to integrate these words into the text requires Sloan to overcome a (minor) problem of textual coherence: now that there is a control board, what exactly does it control? As Sloan continues his impromptu tale, this device comes to control the summoning of an “artist,” ostensibly some sort of artificially intelligent being. Problem solved.
Other suggestions from the system, however, are much more confusing and less easy to integrate. Describing this “artist,” Sloan adds:
...It was enormous, with
then again asks for suggestions. He cycles through the options:
...enormous, with a strong slow shout and a faint squeaking sound
...enormous, with a little purple straw against a wrapped finger and a heavy face
...enormous, with a black box of human hair which was his face, and his lips moved
In the video, the last of these evokes a chuckle from the audience, and Sloan keeps it. However, if this suggestion is somehow more apt or appealing than the others, all three suggestions seem similar: grammatically correct, extremely specific (almost “purple” in their overuse of adjectives), and absurd (a word Sloan himself uses to describe them).
These options point to how inherently impoverished Sloan’s model is relative to Google’s Smart Compose. Google’s tool has (at least) three key technical advantages: 1) it is trained on significantly more data (billions of emails); 2) its statistical model is more complex; and 3) it refines its predictions based on the respective styles of individual users (Chen). We may reasonably suspect another non-technical advantage – that emails, as a genre, are simply more “boring” than fiction (i.e. more statistically predictable, with considerable boilerplate). Rnn-writer deploys the technology of linguistic prediction only to paradoxically make use of its faultiness, the gap between the human and algorithmic writers’ respective senses of what letters should probably/probabilistically come next. In its whimsical spirit, Sloan’s interface is perhaps less closely related to Google’s powerful and polished software than it is to examples of intentionally defective technology such as Simone Giertz’s internet-famous “shitty robots."4See https://www.youtube.com/channel/UC3KEoMzNz8eYnwBC34RaKCQ.
Yet rnn-writer’s purpose is not merely to reveal the smart stupidity of its neural network. If rnn-writer is “deranged” (etymologically, _removed from orderly rows5Etymology furnished by Google Search.) its very design is an argument that our minds are too-firmly stuck in their own orderly rows, and so computer-generated text can “inspire” us simply through its unselfconscious strangeness. The more outlandish (i.e. “wrong”) are rnn-writer’s suggestions, the greater threat they pose to the coherence of the text, and the more skill is required to make use of a textual intrusion that could just as easily seem like sabotage. But this invites the question: why go through the trouble of training a neural network? Deep learning, after all, is costly in terms of time and electricity. Why not insert text randomly plucked from the newspaper? (Simple randomness worked well enough for Tristan Tzara and John Cage.)
Despite their oddness, rnn-writer’s suggestions are not nearly as absurd – not nearly as challenging to integrate – as they could be. In the theory of learning, the “Zone of Proximal Development” refers to that which the student cannot understand or perform alone but can with the assistance of a teacher or more experienced peer, a competency that is just out of reach (Vygotsky). Perhaps a tool such as rnn-writer, when its predictive capacity fails noticeably but not catastrophically, can lead the human writer into a “Zone of Proximal Derangement” – just weirder than what they would have written themselves. That Sloan’s interface makes it easy to cycle through suggestions as well as dismiss them entirely likewise points to a certain theory of how the algorithmic writer can benefit the human one: the neural network’s suggestions aim to be “ergonomic,” challenging but not frustrating, fitted to the writer’s mind and capacities.
Abra’s Power of Surprise
Unlike most iOS apps, which stick to a single catchy name, Abra (2015) has a subtitle: “a living text.” In the app description section on the iOS store, its authors, Amaranth Borsuk, Kate Durbin, and Ian Hatcher, also refer to it as a “magical poetry instrument” and a “spellbook.”
When the user opens Abra on an iOS device, the screen is pre-populated with several lines of computer-generated text, cast in the hues of a muted rainbow. The app gives the user a variety of ways of interacting with this pre-populated poetry, including the simple ability to delete and add text. It is thus a “word processor,” but it is far more than that. Abra possesses a series of functions that transform the poem on the screen. When the user presses the “Cadabra” button at the top of the screen, something “magical” happens. Often the words shuffle to their left and right, switching places slowly or rapidly. Sometimes an emoji appears – usually just one, though every so often the screen will become cluttered with emoji trees, the text suddenly overgrown by a forest.
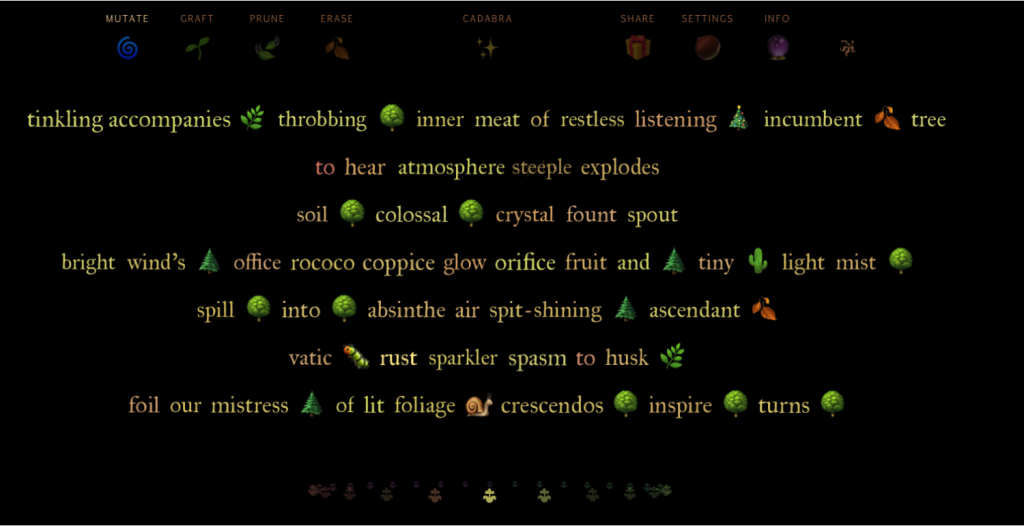
The precise workings of the “Cadabra” button can be discovered in the online repository of Abra’s code (Hatcher). A text file contains the names of each of the 39 “spells” that the “Cadabra” button can cast. Next to each spell is number that reflects the likelihood that the particular spell will be cast6Here I am describing (in somewhat simplified terms) only part of Abra and indeed only part of the “Cadabra” button’s effects. , with higher numbered spells more likely to occur. One function called “AREA_RANDOM” is given the highest number, 28. The second highest number, 7, belongs to the function “RANDOM_ERASE.” The most frequent numbers assigned to functions are 1 and 2, meaning that they won’t occur very often.
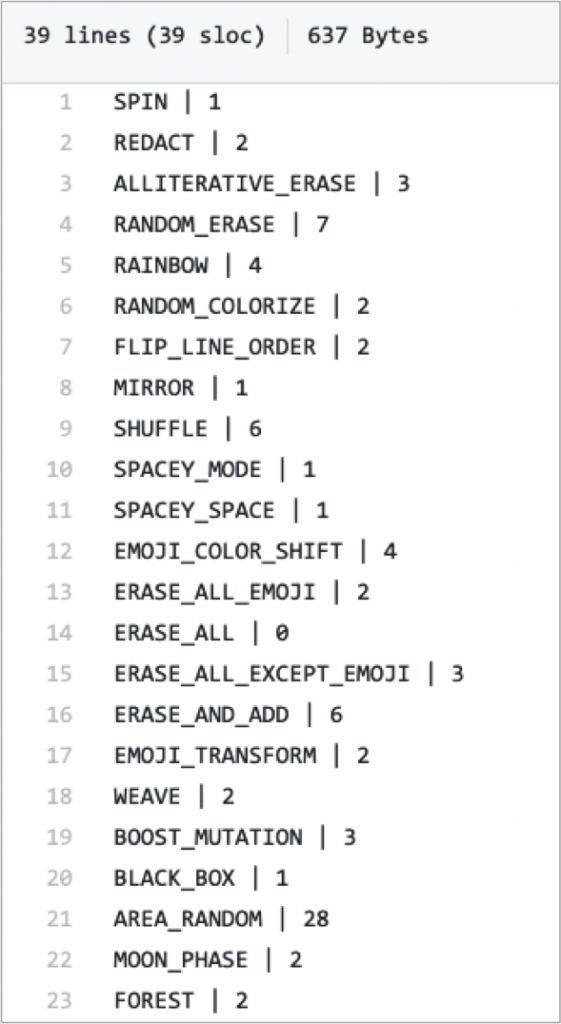
The action of the “Cadabra” button is a matter of probability, with a few functions very common and the rest quite rare. And there is a pattern to which are rare and which are common. The most common ones I would describe as – if certainly bizarre by the buttoned-up standard of Microsoft Word – somehow more neutral. When you press “Cadabra,” the most likely thing to happen is the “AREA_RANDOM” function; this applies some “mutations” to the poem – perhaps changing a word to an orthographically similar one or adding words. “RANDOM_ERASE” is more self-explanatory: it randomly erases some words in the poem. These common functions share a logic: words may appear, disappear, or even morph into another word, but even those linguistic morphings are drawn from a large, heterogeneous list of words. “Swan” may turn into “lawn,” or it may turn into “aswoon,” or it may disappear. The poem is generally smudged, some neutral chaos injected.
The rare functions, on the other hand, tend to be more dramatic and even at times tendentious, implicitly making stronger claims about what the poem should be about. When the “FOREST” function plants tree emoji throughout the text, this editorial inclusion strongly suggests the poem become a sylvan idyll or a Romantic meditation on nature. When the “DEATH” function litters the screen with crosses and skull-and-bones symbols, the text is suddenly Gothic. When “ERASE_ALL_EMOJI” and “ERASE_ALL_EXCEPT_EMOJI” do what their names imply, Abra draws a distinction between emoji and “regular” characters, asserting that one or the other are the only parts of the user’s current text worth saving.
Abra’s hexes may seem utterly capricious, yet the designers have clearly thought about how to structure their magic in order to achieve something like (but not quite equivalent to) “user friendliness.” The common, not-very-specific spells serve as a kind of “background,” an expectation that threatens to lull the writer into complacency. The rare, powerfully tendentious spells violate this expectation, creating surprise. Imagine the opposite: if almost every time the user hit the “Cadabra” button the screen were to become cluttered with glyphs related to death, the appearance of yet more skulls would quickly seem monotonously macabre. Instead the interface itself sets up (through algorithmic, probabilistic mechanisms) the possibility of it doing something that seems out of the blue; the user taps “Cadabra,” expecting the interface to once again change some words or delete them – when suddenly it is overwhelmed with the signs of a memento mori.
The design choices legible in Abra’s code imply a different conception of what writers need than the one manifested by rnn-writer. If the latter suggests that writers need to be led into a “Zone of Proximal Derangement,” pushed just off course, Abra suggests they should be kept dramatically unstable, forced to deal with sudden and much more extreme interference. Randomly injecting critical events such as engine failures and rudder malfunctions into flight simulators has recently demonstrated an ability to improve how pilots respond to real aviation crises (Landman); one might hypothesize that Abra has a similar effect, making the minds of writers more nimble and resilient.
Alternating Current’s Power of Judgment
My third example of an algorithmic co-writer, drawn from my own design-based research in the development of digital tools, explores how an interface can be more explicitly pedagogical by judging the human writer’s expression.
In ancient Greek rhetorical education, the progymnasmata were a series of exercises. Athletic in nature, they aimed to give the student the right amount of flexibility and strength (of the mind and its linguistic faculties) so that this rhetor-in-training could move on to the business of actually composing orations (Webb). For example, paraphrase was the task of reiterating a statement with different syntax (Kennedy). Alternating Current 7See Booten (“Sentiment”) as well as: https://github.com/kbooten/alternatingcurrent. (2019) is one of a series of “digital progymnasmata” – pieces of linguistic training equipment that are designed to stretch the writer beyond typical ways of writing, usually by spurring revision (see also Booten, “Toward”).
A web-based writing interface, Alternating Current first prompts the writer to compose a poem. After hitting “submit,” the interface plots – on a sentence-by-sentence level – the sentiment of the poem, as judged by a classification algorithm. In machine learning, classification algorithms are used to place some piece of data – for instance, a sentence – into (usually just) one category or class from a list of such classes. Sentiment analysis algorithms classify the emotion of a piece of text, most simply as either positive or negative. To be more specific, such an algorithm returns the probability that a sentence belongs to a particular class. In the case of Alternating Current, a classification algorithm has been trained to tell the difference between two groups of posts from the social network Tumblr, one that users had tagged as “#inspiring” (and related words), another that users had tagged with terms like “#depressed.” The interface’s visualization is thus a computer-generated representation of this poem’s emotional “arc."
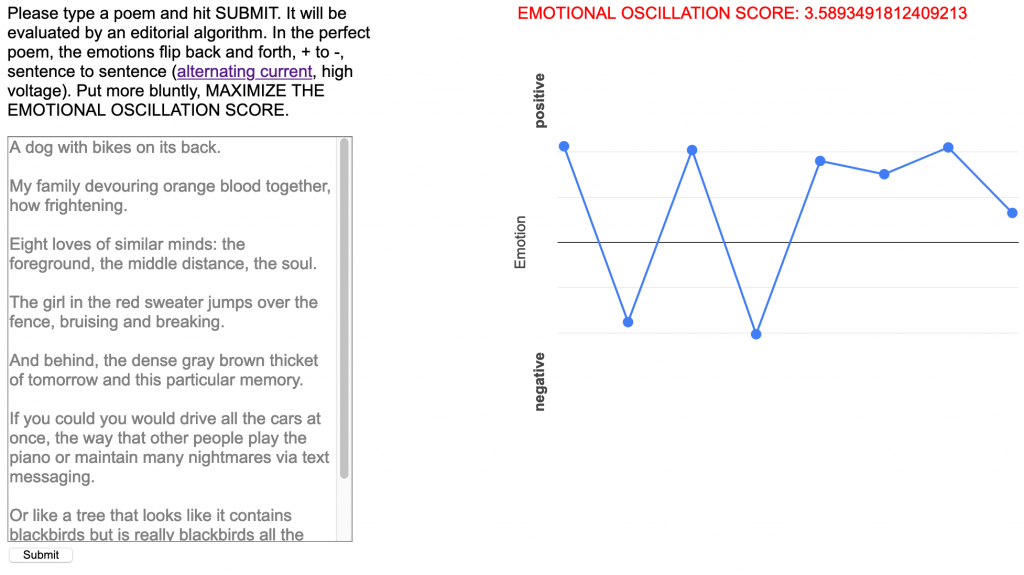
The interface also displays an “EMOTIONAL OSCILLATION SCORE” (EOS), a number that reflects the total shifts in emotion between adjacent lines; a poem whose lines are either all happy or all sad will have a lower EOS than one whose lines oscillate between affective poles. The interface instructs the writer to maximize the EOS, revising the poem so that it takes the form of a very sad sentence followed by a very happy sentence followed by yet another very sad sentence, etc. Each time the writer adjusts the poem and hits “Submit,” each sentence’s affective arc is replotted and the poem’s total EOS recalculated.
What is the point of this exercise? Here my answer must be biographical, since I developed Alternating Current as an experiment in remedying a deficiency I perceived in my own recent poetic writing: a kind of emotional flatness, a dull quietude or (in the clinical sense) apathy, neither positive nor negative at any moment. Even the choice of Tumblr is a personal one; posts on this site often take the form of raw, unvarnished expressions of emotion, exactly the characteristics I found lacking in my own verse. In this way, the design of Alternating Current represents a very straightforward form of linguistic therapy, one that is indicated for an equally specific diagnosis.
Still, this explanation does not get at what it is like to write specifically with a machine learning system. After all, a writer might “manually” (without the feedback of such an interface) revise a poem so that it oscillates between happy and sad sentences. Yet in this case the writer is left only with their own sense of what sort of language suggests positivity and what indicates dejection. Because a machine learning model is a “black box,” information that is not immediately human-readable, conforming to it requires guesswork: given what I know about language in general as well as the Tumblr posts I have read (far fewer than the algorithm itself has “read”), what sorts of words or sequences of words might I expect to correlate with the #sadness? A wrong guess may reveal asymmetry between an individual’s human intuitions and the statistical correlations observed by the machine learning model. I would have thought the sentence
The moon will always know hate.
to be negative, and the sentence
The moon will always know love.
to be positive. To my surprise, Alternating Current’s language model tells me that they are both similarly negative. It seems that in the context of #depressing Tumblr posts, “love” itself is a source of sadness nearly as much as “hate.” Each revision of a line becomes an attempt to get out of one’s own mind and into the mind not just of the algorithm but of the genre of texts upon which it was trained and thus (albeit quite obliquely) of the people who wrote them. Against a certain view of poetry as the genre most amenable to the individual writer’s linguistic idiosyncrasy and obscure meanings, the judgments of the model implicitly attempt to re-socialize the writer into common language and fellow feeling. Yet (unlike with Google’s autocomplete) this process is not automatic, and so it invites the human writer to think through the gap between their mind and statistical norm.
Forms and Affordances: Toward “Literary HCI”
Each of these algorithmic co-writers enacts a different assumption about how best to push the human writer’s mind out of its typical orbit. What are the relative strengths and limitations of these co-writers’ approaches – the power of suggestion, the power of surprise, and the power of judgment?
These questions are, at least in part, empirical ones. To answer them would likely require the field of electronic literature to see itself in deeper continuity with more mainstream forms of Human-Computer Interaction (HCI) research. In this field, it is generally not enough to produce new digital tools; researchers must also systematically (and often experimentally) analyze how actual users employ these systems. Insofar as electronic literature is also invested in the production of tools – not just works of literature – it should learn from HCI’s perspectives on how to rigorously study the tool-mediated dimensions of human thought and creativity. To be clear, the fields of e-lit and HCI are not totally separate. For example, empirical research has already explored systems similar to rnn-writer (Manjavacas et al.; Roemmele and Gordon), ambient literature (Marcinkowski, “Reading”), and digital fiction (Bell et al.).8Marcinkowski (“Methodological Nearness”) offers another perspective on why the study of e-lit should embrace HCI. But, within the study of electronic literature, such empirical approaches are far from de rigueur. An HCI investigation of rnn-writer could interrogate the kinds of linguistic contortions that human writers make to skillfully recuperate the “deranged” suggestion of the algorithm. One might study Abra by comparing how writers compose with different versions of it, each programmed to provide more or less surprise. A study of Alternating Current could explore the difficult question of whether or not it makes the writer literally feel differently. One might also wonder if sustained training with any of these tools would transform how writers write without them.
Electronic literature – staying rooted in the broader literary field – should also see its attempts to create interfaces as in continuity with the more general question of literary form, especially as it relates to constraint. Certainly the examples of co-writers discussed above resonate with the kinds of constraint-based writing practices developed by the OuLiPo, techniques designed to push writers beyond “the range of normal speech and language registers” and toward “linguistic virtuosity” (Symes 96). Yet this is only one pre-digital connection. From the perspective of Cognitive Poetics,9Or, to use Cave’s vocabulary, Cognitive Criticism. all literary forms and genres may be thought of as tools for thinking that have specific “affordances” – i.e. that help us to think in new ways (Cave). That the sonnet form has endured, Terence Cave argues, suggests that writers have found its features (such as the _volta or “turn”) to be enduringly useful as well as flexible, able to provide new utility as the cultures that embrace the sonnet have themselves evolved.10In a fascinating reconsideration of the technicity of poetry, Brian Kim Stefans proposes that the successful lyric poem is generated by a “spectral, suspended class” – a kind of cognitive machine, an invisible algorithm that the poet activates and adumbrates through writing the specific poem (88). I mention Stefans’s theorization to make clear that it is not only well-specified, canonical forms like the sonnet that could be thought of as tools with cognitive affordances. Yet, if the sonnet is a cognitive tool, it is a simple machine; it lacks the “moving parts” of a computational interface and cannot morph in response to what the writer has written. Moving forward, practitioners of what might be called “literary HCI” should take stock of the affordances that are specific to the digital interface while also placing their efforts within a broader map of literary forms as tools of the mind – tools that structure, unsettle, assuage, and amplify.
But the question of literary forms as tools of the mind extends well beyond the purely literary domain. To return to Stiegler: one of the most pressing political imperatives of our time is to discover how to repurpose digital media, which threatens so much harm to our minds, in ways that instead refocus cognition in beneficial, careful ways. This he has called the “contemporary battle for intelligence” (_Taking Care 93). A “literary HCI” that focuses on the cognitive experiences that texts foster could play an important – if speculative – role in this battle. And while, as N. Katherine Hayles has argued, the creation of any “cognitive assemblages” involving humans and technical objects offers a chance for us to “design ourselves” anew (55), algorithmic co-writers are particularly vivid examples of this potential. These tools imply that the human mind needs assistance or even retraining, whether strange or stern. To design one is to offer an answer to the key question: amidst this battle for intelligence, what do minds need?
Acknowledgments
Sincere thanks are due to the anonymous reviewers of ELO 2020 for their insightful suggestions.
References
Bell, Alice, Astrid Ensslin, Isabelle van der Bom, and Jen Smith. “Immersion in Digital Fiction: A Cognitive, Empirical Approach.” International Journal of Literary Linguistics, vol. 7, no. 1, 2018, https://journals.linguistik.de/ijll/index.php/ijll/article/view/105.
Booten, Kyle. “Sentiment: A Lab Notebook.” Tentacular, no. 4, 2019. https://www.tentacularmag.com/issue-4a/kyle-booten.
---. “Toward Digital Progymnasmata.” Proceedings of the 10th International Conference on Computational Creativity, edited by Kazjon Grace, Michael Cook, Dan Ventura, and Mary Lou Maher, ACC, 2019.
Borsuk, Amaranth, Kate Durbin, and Ian Hatcher. Abra: a living text. iOS App Store, 2015.
Cave, Terence. Thinking with Literature: Towards a Cognitive Criticism. Oxford UP, 2016.
Chen, Mia Xu, et al. “Gmail Smart Compose: Real-Time Assisted Writing.” Proceedings of the 25th ACM SIGKDD International Conference on Knowledge Discovery & Data Mining, Ankur Teredesai and Vipin Kumar, ACM, 2019.
Emerson, Lori. Reading Writing Interfaces: From the Digital to the Bookbound. University of Minnesota Press, 2014.
Hatcher, Ian. “spell_odds.txt.” Abra, GitHub, 2015, https://github.com/ihatch/abra/blob/master/Abra/spellodds.txt. Accessed 15 November 2019.
Hayles, N. Katherine. “Cognitive Assemblages: Technical Agency and Human Interactions.” Critical Inquiry, vol. 43, no. 1, 2016, pp. 32-55.
Kaplan, Frederic. “Linguistic Capitalism and Algorithmic Mediation.” Representations, vol. 127, no. 1, 2014, pp. 57-63.
Kennedy, George Alexander, editor. Progymnasmata: Greek Textbooks of Prose Composition and Rhetoric. Brill, 2003.
Landman, Annemarie, et al. “Training Pilots for Unexpected Events: A Simulator Study on the Advantage of Unpredictable and Variable Scenarios.” Human Factors, vol. 60, no. 6, 2018. pp. 793-805.
Marcinkowski, Michael. “Methodological Nearness and the Question of Computational Literature.” DHQ: Digital Humanities Quarterly, vol. 16, no. 2, 2018. http://www.digitalhumanities.org/dhq/vol/12/2/000378/000378.html.
---. “Reading Ambient Literature: Reading Readers.” Participations, vol. 16, no. 1, 2019, pp. 259-279.
Manjavacas, Enrique, et al. “Synthetic literature: Writing Science Fiction in a Co-creative Process.” Proceedings of the Workshop on Computational Creativity in Natural Language Generation (CC-NLG 2017), edited by Hugo Gonçalo Oliveira, Ben Burtenshaw, Mike Kestemont, and Tom de Smedt, ACL, 2017.
Roemmele, Melissa and Andrew S. Gordon. “Creative Help: A Story Writing Assistant.” Proceedings of the 8th International Conference on Interactive Digital Storytelling, Copenhagen, Denmark, 2015.
Sloan, Robin. Rnn-writer. GitHub, 2016, https://github.com/robinsloan/rnn-writer. Accessed 10 November 2019.
---. “Writing with the Machine.” robinsloan.com, 2016, https://www.robinsloan.com/notes/writing-with-the-machine/. Accessed 15 November 2019.
---. “Writing with the Machine.” Eyeo Festival, vimeo, 2017, https://vimeo.com/232545219. Accessed 15 November 2019.
Stefans, Brian Kim. Word Toys: Poetry and Technics. University of Alabama Press, 2017.
Stiegler, Bernard. Automatic Society 1: The Future of Work. Translated by Daniel Ross, Polity Press, 2016.
---. Taking Care of Youth and the Generations. Translated by Stephen Barker, Stanford UP, 2010.
“SUBJECT: Write Emails Faster with Smart Compose in Gmail.” The Keyword, 8 May. 2018. https://www.blog.google/products/gmail/subject-write-emails-faster-smart-compose-gmail/.
Symes, Colin. “Writing by Numbers: OuLiPo and the Creativity of Constraints.” Mosaic: A Journal for the Interdisciplinary Study of Literature, vol. 32, no. 3, 1999, pp. 87-107.
Vygotsky, Lev Semenovich. Mind in Society: The Development of Higher Psychological Processes. Edited by Michael Cole, Vera John-Steiner, Sylvia Scribner, and Ellen Souberman. Harvard University Press, 1980.
Webb, Ruth. “The Progymnasmata as Practice.” Education in Greek and Roman Antiquity, edited by Yun Lee Too, Brill, 2001, pp. 289-361.