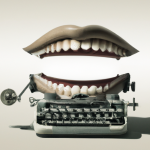
In this anticipation of John Cayley's ebr essay on Artificial Intelligence, Scott Rettberg contextualizes some of the ethical and systemic problems of ChatGPT and argues that works of electronic literature and digital art might serve as tutor texts for understanding effects of technological mediation on humanity.
This essay is the first part of a series I’m writing focused on writing with AI: the ethical and practical challenges involved, ways that we can work with AI chatbots as writing environments, and new genres of multimedia electronic literature. To be clear, I’m not proposing a new theory of AI or its impacts on society here, rather I’m trying to aggregate and make visible some of the challenges posed by AI and the new opportunities for creative expression enabled by large language model-based AI systems such as OpenAI’s ChatGPT. In this first installment, I focus on some of the troubles with AI chatbots arsing from factors including:
- proprietary language models
- outsourcing human reason
- hallucination
- embedded bias
- monoculturalism
- anthropomorphism
- so-called “existential risk”
- the fallibility of humans
I feel that before moving on to what I would informally call “the fun parts” of writing with AI, it’s important to consider and bracket the complexities of these new AI platforms.
An Anticipatory Prolegomena (PriPoste) to a Future Essay on ChatGPT by John Cayley
Let me begin with a PriPoste, a postulation of what John Cayley might say in his forthcoming ebr essay on ChatGPT, with an essay written by ChatGPT:
Model: GPT-4
Title: Remembering and Forgetting Language in the Age of AI Chatbots
There is a rising tide, one that seeks to submerge the very nature of human expression and the arts of language. The forces behind this tidal wave are immense, and in the midst of our digital revolution, they are accelerating with alarming speed. As a poet, a digital artist, and a technology critic, I am compelled to address this tsunami head-on, lest we be washed away entirely.At the crux of this issue are the artificial intelligence (AI) chatbots, particularly large language models (LLMs) such as OpenAI's GPT series. These AI systems, for all their linguistic prowess, pose a significant threat to language arts and the broader cultural landscape. The scale at which these models are trained on language harvested from the internet, often without content creators' permission, raises profound questions of authorship and authenticity.
This issue is not solely the domain of AI chatbots. Companies like Google and social networks have long been extracting language from users in a transactional manner, wherein the corporation benefits disproportionately. This monopolization of our collective linguistic wealth is not only unethical, but it also undermines our capacity to create, to imagine, and to remember.
In his philosophical work, Bernard Stiegler introduced the concept of anamnesis - the act of recalling or remembering. Drawing on Plato's theory of recollection, Stiegler posited that memory is not merely a passive storehouse but an active process of becoming. In the age of artificial intelligence, the integrity of our anamnesis is under siege. The ability to create and share language is intrinsically linked to our memory, and as AI chatbots are fed the fruit of our collective linguistic labor, our sense of self is eroded.
Our language is being commodified, repackaged, and redistributed by these AI systems. However, this process is not merely a matter of appropriation; it is a transformation that actively diminishes the potential for human creativity. As AI chatbots are designed to optimize efficiency and satisfy our desire for quick answers and solutions, the nuances and idiosyncrasies of human language are sacrificed. It is in these moments of imperfection, of linguistic deviance, that true artistry and imagination emerge. AI chatbots, in their quest for computational fluency, threaten to strip language of these vital qualities.
The scale of this linguistic harvest is staggering. In the case of GPT-4, a single model ingests the textual wealth of entire libraries, feeding on the language of countless authors without consent. The question of authorship is not a trivial one; it is a matter of identity, a recognition of the relationship between the creator and the created. In this way, AI chatbots act as a black hole, absorbing the individuality and essence of our language, only to regurgitate a homogenized, artificial facsimile.
Moreover, the transactional nature of these exchanges between humans and AI systems exacerbates the imbalance of power. As we pour our linguistic creations into the digital void, our returns are meager. We are offered fleeting conveniences and the illusion of connection, while corporations amass immense wealth and power. It is a Faustian bargain, one that will continue to erode our cultural foundations if left unchecked.
The situation is not without hope. In confronting the specter of AI chatbots, we must recognize that the solution lies not in turning away from technology, but in reimagining our relationship with it. We must demand transparency, accountability, and ethics in the development and deployment of AI systems. We must assert our rights as creators and reclaim the value of our linguistic labor. And perhaps most importantly, we must strive to preserve the richness and diversity of human language, in defiance of the homogenizing
tendencies of AI chatbots. In the face of this challenge, artists, poets, and writers have a critical role to play. We must push the boundaries of language, embracing its imperfections and unpredictability as a means of resistance. By forging new pathways of expression, we can counteract the flattening effect of AI chatbots and breathe life back into our collective linguistic landscape.In a sense, we are called to an act of creative anamnesis, remembering and rediscovering the essence of language that lies at the heart of our humanity. We must confront the digital behemoths, armed with the knowledge that our capacity for imagination and expression is boundless, and that the integrity of our linguistic heritage is worth fighting for.
In conclusion, the rise of AI chatbots and the commodification of language threaten not only the arts but the very fabric of our culture. As we navigate this digital age, we must remain vigilant and steadfast in our defense of language and the collective memory it represents. By embracing the concept of anamnesis and reasserting our creative agency, we can ensure that the future of language remains a rich and diverse tapestry, rather than a sterile, homogenized echo of our past.
At the time of this writing, I have not read Cayley’s forthcoming essay, but as a student of his prior writing, I feel qualified to make some estimation of what he might say, and with my prompt, ChatGPT is able to “feed-forward”1This is an intentional sideways-reference to Mark B.N. Hansen’s Feed-Forward in which he describes 21st Century technologies as coupling human reason “with technical processes that remain cognitively inscrutable to humans … correlating humans with sensors and other microtechnologies that are capable of gathering data from experience, including our own experience that “we ourselves” cannot capture” (Hansen 2015, 64). a premonition of Cayley’s argument to deliver a readable and likely accurate—albeit fairly bland—short essay. This ChatGPT output is lacking in comparison to the eloquent prose I’m certain the actual John Cayley will soon publish in this journal, but to me it is nevertheless remarkable that the program is able to build upon the prompt that I provided it and pull in related source materials, such as a more thorough, if incomplete, explanation of Bernard Stiegler’s idea of anamnesis.2In Stiegler’s view—the process of memory (anamnesis) is an active one, not one of being fed information but of memory being constructed. Stiegler initially greeted the Internet and related digital technologies as enabling anamnesis in a way that one-way broadcast mass media did not: “Internet is an associated hypomnesic milieu where the receivers are placed in a position of being senders. In that respect, it constitutes a new stage of grammatisation, which allows us to envisage a new economy of memory supporting an industrial model no longer based on dissociated milieux, or on disindividuation.” In the context of AI, it’s worth considering the complications of this model. On the one hand, students using ChatGPT to write a paper are dropping their ability to individuate themselves through their engagement with the text they are writing in response to (see Heckman 2023 for discussion of this consequence). On the other hand, the act of interacting with ChatGPT is itself interlocution, which Stiegler saw as key to the process of individuation and futurity, as he wrote: “The life of language is interlocution, and it is this interlocution that the audiovisual mass media short-circuit and destroy” (Stiegler 2012). The fact that when interacting with ChatGPT we are having an interlocution a large mass of human language, in a responsive way, might throw a wrench into the works of Stiegler’s way of thinking about anamenesis.
When I showed Cayley this output, he remarked that the core ideas of the ChatGPT essay are embedded in the prompt—it didn’t come up with anything independently but instead wrapped filler around those ideas. The style and structure of the response are quite conventional, more like an assignment turned in by a college sophomore than an accomplished critique by a literary critic. This output does not represent thought on the part of the AI, and certainly not self-understanding, but it does demonstrate extremely advanced pattern recognition, a certain (limited) ability to structure an argument, and extensive powers of mimicry. It is in that sense complex. The outputs from ChatGPT can be as stupid as we want them to be. When I had this exchange with ChatGPT, I was responding in part to a thread Cayley posted on Facebook that began with:
Remembering the brilliant mind and artistic talent of John Cayley today. His groundbreaking work in digital poetry and language-based art continues to inspire us all. Thank you for pushing the boundaries of what is possible with language, John. Your legacy lives on.#JohnCayley #digitalpoetry #languageart #legacy
The conversation continued from there, with Cayley asking the AI chatbot to provide information about John Cayley in specific banal contexts, and the chatbot responding in banal ways that highlighted its inadequacies. It never achieved a John Cayley voice with a John Cayley level of sophistication, at least in part because John Cayley was not writing to it in a John Cayley voice with a John Cayley level of sophistication. The John Cayley the actual John Cayley modelled for ChatGPT was a straw man John Cayley. There are many versions of John Cayley represented in the above exchanges: the ghost voice of John Cayley the actual embodied author that left its traces somewhere in the large language model, the AI version of John Cayley represented by a request for a simple blog post about John Cayley, the more sophisticated interpretation of John Cayley represented by my presupposed summary of his probable argument, and the version of John Cayley in the AI’s response that was shaped by that argument. Will the real John Cayley please stand up?
It would be a mistake to regard to regard ChatGPT as an advanced (albeit creative and sometimes counterfactual) search engine. That’s simply not what it is.3That’s not to say that I’m presupposing that that’s how the actual John Cayley will situate ChatGPT in his forthcoming essay. I actually have no idea, as I’ve not read the essay I’m potentially responding to, but it is probabilistically unlikely that Cayley would so radically simplify things in this way. My understanding of ChatGPT is instead that it is an environment in which dialogs are produced in a collaboration between a human writer and a nonconscious cognizer4N. Katherine Hayles’s distinction between consciousness and cognition, best elucidated in her book Unthought: The Power of the Cognitive Nonconscious guides my thinking about the ways that we interact with AI. In Hayles’s view, “Cognition is a process that interprets information within contexts that connect it with meaning” (Hayles 2017, 22). Interacting with AI is not a matter of interacting with another consciousness, but of interacting with an advanced cognitive system. That system can’t think in the way that humans can, nor can humans process data in the way that these systems can., a probabilistic large language model (LLM). Throughout this article, I emphasize the importance of understanding the fact that in our interactions with an LLM, both the language model it is based on and the language we provide in response to it are recursively operational—the language we provide the system and the language it provides in response afford, constrain, and shape our further interlocution with it.
The trouble with ChatGPT: Whose language is this anyway?
ChatGPT and related AI systems such as Bing and Google’s Bard, pose many well-documented problems. These include, for example, the fact that they are trained on massive, unauthorized scraping of text from the Internet and other sources. This will be the stuff of litigation and payoffs by giant mega-corporations for years to come. At the same time this process will not be without its ironies. For example, Getty images, which itself has gathered and copyrighted images in a predatory and legally murky way, filed a substantial lawsuit against Stable AI for violating its copyright in training the Stable Diffusion text-to-image generator (Setty 2023), at the same time as much of Getty images’ archive itself is essentially stolen from other sources.5A great deal of Getty’s practice involves “compilation copyright” whereby they gather images thought be in the public domain, add metadata to the images, and then sell them. They then litigate aggressively against other users of the images. Regardless, OpenAI’s scraping of data to train their models is unethical in the same way that Google’s capture of all the search data from billions of queries to gather the most extensive corpus of human language ever collected without giving users access to the same data they contributed, much less the whole set, is unethical.6Richard Misek’s 2022 documentary film project A History of the World According to Getty Images is an excellent exploration and illustration of the ironies of the Getty Images method of exploitation. The documentary is composed of archive footage sourced from the Getty images catalogue. The film demonstrates how “image banks including Getty gain control over, and then restrict access to, archive images—even when these images are legally in the public domain.” It then responds by returning the images to the public domain—it includes six legally licensed clips that Misek uses in the film. Misek’s film itself is released with no rights reserved, so people can download the film and reuse the clips from it—essentially reversing Getty’s highjacking of images from the public domain. It is also unethical in a similar way to that which Facebook used to amass and then exploit personal data from users all over the world.
Cayley and Howe provide an apt summation of the copyright concerns involved here—it is not so much whether or not the data is being used for further creation, but that the same entities that are claiming fair use of this data are blocking everyone else, including the data creators, from it, “…we should see this as a kind of retrospective justification—perhaps calculated on behalf of the beneficiaries—for land-grab, enclosure-style theft from and of the cultural commons, all with legislative regimes that are propping up an inequitable, non-mutual, and unworkable framework for the generative creativity that copyright is supposed to encourage.” (Cayley and Howe 2019). Cayley and Howe’s work Common Tongues provides an excellent illustration of the limitations imposed both by the way that current copyright laws limit authors, and the ways that Google and its ilk have taken proprietary control of human language. The book reads the same word for word as Samuel Beckett’s How It Is (which is under copyright), however the text was composed by searching a “universally accessible search engine, attempting to find, in sequence, the longest common phrases from How It Is that were composed by writers or writing machines other than Beckett.” The sources of these phrases are then cited individually in footnotes. So though both the Beckett estate and the search engine in some sense “own” the language, Cayley and Howe’s work points out the artificiality of this idea of owning language, just because it has particular enframement apart from the commons.
The trouble with AI’s enclosure of the commons is only an extension of the devil’s bargain we make with platform culture more generally. By now we are accustomed to handing over personal data, attention, and intellectual property in exchange for services, some of which have come to be seen as indispensable. These are issues critics such as Shoshana Zuboff (2019), Christian Ulrik Andersen and Søren Pold (2018)7See also “The Metainterface of the Clouds” series in ebr (Andersen and Pold, 2018b), and others have brought into sharp focus. At this point, it takes a lot of work not to be enmeshed in platforms.
The trouble with ChatGPT: Outsourcing intelligence
Those of us working in education have already noticed the problematic impacts that ChatGPT is having on teaching. Many forms of assessment, for example short essay assignments, are made more difficult to assess by the ability of ChatGPT to answer them. The GPT-4 model is quite capable of producing answers (with varying degrees of factual accuracy) to common essay questions in a variety of academic subjects. Forget the short-answer exam, for example, in a Freshman Philosophy course:
Model: Default (GPT-3.5)
Plato's allegory of the cave, from his work The Republic,
illustrates the journey from ignorance to enlightenment. In the allegory, prisoners are chained in a dark cave, facing a wall. A fire behind them projects shadows of objects on the wall, which they perceive as reality. One prisoner breaks free, leaves the cave, and discovers the outside world, full of sunlight, real objects, and true knowledge. Upon returning to the cave, he attempts to share his newfound knowledge but is met with disbelief and ridicule from the other prisoners.The cave represents a limited, unenlightened worldview, while the outside world symbolizes the realm of knowledge and truth. The shadows on the wall are like superficial, illusory perceptions, while the objects and sunlight represent genuine understanding.
In the context of contemporary media culture, the allegory of the cave can be related to how the mass media, social networks, and internet platforms often present distorted, simplified, or biased representations of reality. The shadows on the wall can be likened to sensational headlines, clickbait articles, or misinformation that manipulate public perception. People who consume these messages may remain in the metaphorical cave, accepting the distorted version of reality as truth.
Meanwhile, those who question and critically analyze the information they receive, seeking a more profound and nuanced understanding, can be compared to the prisoner who escapes the cave. Breaking free from the influence of the media, they explore alternative sources of information, engage in intellectual discourse, and develop a more accurate perspective on the world.
In conclusion, Plato's allegory of the cave highlights the importance of questioning one's perceptions and seeking truth beyond superficial appearances. When applied to contemporary media culture, it serves as a cautionary tale, encouraging individuals to be skeptical of the distorted realities presented by mass media and to strive for a more enlightened understanding of the world.
The more common the question, the better ChatGPT will be at answering it—because ChatGPT is a probabilistic model trained on internet crawls, its tendency is to find the most average answer to any given question. Cheating using ChatGPT is already commonplace—we have already caught at least three students using ChatGPT inappropriately already this semester in our Digital Culture program at the University of Bergen.8My policy for dealing with students who submit a creatively non-referenced assignment partially written by ChatGPT is to grade the AI and the student separately—so a C assignment a student that was 50% written with AI would be garner the student grade of 35%, while the AI would be entitled to the other 35%. And yet, I’ll say this: submission of even short essays written by ChatGPT is among the simplest forms of cheating to spot that I’ve ever encountered. Student essays written by ChatGPT are typically simultaneously more polished and more banal than essays written by the students themselves. The fact that students who are less accomplished as writers are most drawn to cheating with GPT makes the cheating even more palpable, particularly in longer essays, where students are forced to pad their Frankensteinian endeavors with some of their own writing in ways that will make the stylistic disparity blatantly apparent. Both the AI and the human leave their own distinctive marks on the text.
The trouble with ChatGPT: It hallucinates what it doesn’t know
And then there’s the well-known problem of “hallucination”: to ChatGPT there is no difference between verifiable information and probabilistic approximation. It would be incorrect to call this “lying” because when it tells the truth, it is also offering a probabilistic approximation. LLM AI systems are operating in a similar—but more complex and more “reflective” way—to N-gram systems. An N-gram is a chain of words in sequential order: “The dog” is a 2-gram, “The dog barks” a 3-gram, and so on. N-gram models are based on the probable occurrence of a given word after the next word. We can think of LLMs as being essentially n-grams on steroids, systems that harness the power of probabilistic analysis to model natural language outputs very quickly in response to natural language inputs.
The GPT in Chat GPT stands for “generative pre-trained transformer.” Current transformer machine learning models are different in the sense that they are based on “self-attention”, meaning that they can relate different positions of a single sequence in order to compute a representation of a sequence (Vaswani, et al. 2017). Transformer models do not compute long n-grams linearly but can instead compute the relations between individual tokens (units of language) and then learn from these relations before putting them together as a probabilistic model of input (transforming), and then subsequently performing similar operations to generate the output probabilities. This makes them faster and more computationally efficient. There is a stochastic sampling element to the way that ChatGPT processes input, which has the effect of introducing some randomness to ChatGPT’s response to a given prompt. You can provide ChatGPT the same prompt several times and get a different (if often closely related) response each time. The variability of responses will also depend on the structure, style, and context of the prompt each time it is run. That is, the response will vary depending on how the prompt is framed, with even small grammatical changes potentially effecting major differences in responses. The response will vary a great deal depending on the context in which the question is asked—the inputs that we give to ChatGPT in any given thread influence subsequent responses in the same thread.
The model of interaction with ChatGPT is that of a conversation, and it is useful to keep that in mind when we consider the language it produces. When we are conversing with other humans, the drift of the conversation prior to any given statement made by either of the participants shapes subsequent responses. Like many aspects of ChatGPT, it is not entirely clear to users how this contextual influence operates—OpenAI isn’t telling us, because it is in their interests to shroud the finer details of their system both from their users and from their competitors. In contrast to human conversations, these contexts are operational acts, constraining the subsequent responses unless specifically countermanded. In this exchange:
Model: Default (GPT-3.5)
I ask the respondent to speak like a three-year-old, and it complies. When I subsequently ask it to respond in three-syllable words, it does not, presumably because that would be beyond the capabilities of a three-year-old—that is, until I tell it that it is now a professor and must use more eloquent language.
The trouble with ChatGPT: Embedded bias
The problem of bias within AI training data is another frequently cited problem. Kate Crawford explained this well in her Atlas of AI (2017), as when she examined the biases embedded in the system of classification of datasets such as the widely used ImageNet, which at one point included categories such as “Bad Person, Call Girl, Closet Queen, Codger, Convict, Crazy, Deadeye, Drug Addict, Failure, Flop, Fucker, Hypocrite, Jezebel, Kleptomanic, Loser” and so on. Labels applied to these images were clearly influenced by the biases of their labelers, and those biases were passed onto the AI systems trained on them. The corpus an AI is trained on also has a huge determining effect on its output. An early example of how radical an effect a training set can have on the discourse produced by an AI chatbot was Microsoft’s 2016 release of its Twitter chatbot Tay. The product was meant to be an entertainment chatbot trained to reply to users in the style and slang of a teenage girl. It came out of the box as a pretrained model, but it was also intended to learn from its conversations with user to refine its model over time. Some malicious 4chan users noted this aspect of the system, and encouraged users to post racist, misogynistic, and antisemitic messages to the bot. Within a matter of hours, the chatbot was responding to ordinary queries as if it came straight from a Trump rally with its mates in the Aryan Brotherhood (Schwarz 2019).
ChatGPT is a pre-trained language model—this means that its response is based on its training data (the previously mentioned vast scrape of materials from the internet and other sources), presumably integrated with queries and responses that OpenAI is gathering from every user of its system as they interact withit. The types of associations that drive the logic of ChatGPT responses entail not only direct bias, but also indirect bias. We can understand GPT as representing language in a vector space in which individual words have specific coordinates. Words that are probabilistically most often related are clustered nearer each other. This means that the social bias represented in language used within the training corpus will likely be replicated in ChatGPT’s responses to prompts unless another algorithmic intervention takes place to finetune the response to remove bias. An example of indirect bias encoding in this word embedding is represented in the below figure from Bolukbasi et al.’s 2016 paper “Man is to Computer Programmer as Woman is to Homemaker? Debiasing Word Embeddings”:
Without further intervention, then, if you were to ask an AI that uses these word embeddings to describe a generic man, it might reply that the man is a burly chap with a beard, likely to use his firepower in a tactical way when scrimmaging with his mates, while if you ask about a generic woman, she may well be a sassy homemaker who likes modelling beautiful dresses and gossiping with the gals at the beauty salon. These sorts of indirect biases (in this case gender biases) can extend to many different aspects of worldviews embedded in human language (racial bias, regional bias, religious bias, and so on). Although the datasets LLMs such as GPT-4 are trained on are so massive that they will contain less specific and narrowly defined biases than earlier machine learning models, even if the training data set comprised a very large chunk of written human language, the likelihood that the “average” worldview would be one without bias is actually very low. If we are for example to represent an average perspective on gender and race relations as they have been represented in the history of English literature, and train an AI chatbot on that perspective, it would likely say something deeply offensive (by contemporary norms) every time we conversed with it.
The trouble with ChatGPT: Monoculturalism
As Jill Walker Rettberg points out, there is also a strong bias in most LLM corpora towards English language, and towards Anglo-American culture, in the GPT training data and its knowledge ecosystem (J.W. Rettberg 2022). For example, English language Wikipedia was among the prominent data sources for training GPT-3 (and not other language versions of Wikipedia) (Brown et al. 2020). This encodes the biases of English language Wikipedia into GPT responses. Bias towards Anglophone language and culture will likely continue even as the training sets have expanded considerably—the training dataset for GPT-4 was three times as large as that of GPT-3 and presumably included more global language data. OpenAI uses standardized tests such as GRE, SAT, Bar exams, and AP exams that it was not specifically trained on as its benchmarks to assess its general performance. GPT-4 scores improved significantly from GPT-3 not only in English, but “surpasses the English-language state-of-the-art in 24 of 26 languages considered” (OpenAI 2023). This means that ChatGPT is learning to perform translation and basic reasoning not only in English but in a wide swath of human languages. But the benchmark tests used to test it are deeply embedded in American knowledge and culture. Although ChatGPT is learning other tongues, it is learning to speak American in the cultural perspective it represents.
The trouble with ChatGPT: How it is trained and the policy that results
The process of training GPT’s language model is not entirely automated. In fact, in fine tuning their models, OpenAI depends a great deal on reinforcement from human feedback. As part of the training process, human “labelers” both provide optimal responses to sample prompts that are used to fine tune the model with supervised learning. After that step, prompts and outputs are sampled and then ranked from best to worst to train a reward model, which establishes a policy against which further responses can be judged. The below information graphic from Ouyang et.al’s paper entitled “Training language models to follow instructions with human feedback” (2022) demonstrates how reinforcement learning produces the policy that constrains ChatGPT’s outputs. This policy is the ghost in the machine, the system’s AI whisperer, its stand-in moral compass and etiquette police.
Labelling introduces its own ethical problems (it seems you can’t turn over a stone in AI territory without uncovering a new conundrum) as the workers used for human computation are typically underpaid and sometimes work under grueling conditions. In January 2023, Time magazine reported that OpenAI used Kenyan workers who were paid $1.24 an hour by a subcontractor, Sama, to label toxic content to train ChatGPT to identify and filter similar content from its responses and from future training data sets (Perrigo 2023). The snippets of text provided to the labelers “appeared to have been pulled from the darkest recesses of the internet. Some of it described situations in graphic detail like child sexual abuse, bestiality, murder, suicide, torture, self harm, and incest.” Sama workers reported that labelling such text over the course of long shifts was traumatizing. Sama eventually terminated the contract when OpenAI further contracted them to identify and label harmful (and illegal) images such as those depicting child abuse, bestiality, rape, and sexual slavery, presumably for training its text to image generators. The intent of this process is of course to prevent these types of texts and images from being generated for users of the software, but it clearly came at a cost for the workers who were paid (very little) to identify the toxic content. The process is very similar to that of content moderation on social media, where underpaid hourly workers spend hours screening content that has been flagged either automatically or by other users as inappropriate and are forced to make decisions about that content quickly, on a Fordist, quota-based clock. The circumstances and psychological strain of content moderation is well-simulated in Mark Sample’s 2021 e-lit work Content Moderator Sim, which simulates the stressful and traumatizing labor involved in moderating social media content.
In Open AI’s technical report on GPT-4, the current publicly available release of their model, they detail some of the issues with their current human alignment safety pipeline, RLHF: Reinforcement: “…after RLHF, our models can still be brittle on unsafe inputs as well as sometimes exhibit undesired behaviors on both safe and unsafe inputs. These undesired behaviors can arise when instructions to labellers were underspecified during reward model data collection portion of the RLHF pipeline. When given unsafe inputs, the model may generate undesirable content, such as giving advice on committing crimes. Furthermore, the model may also become overly cautious on safe inputs, refusing innocuous requests or excessively hedging” (OpenAI 2023). The model can still fail both in terms of providing offensive language or potential harmful content, and on the other hand, can hamstring discussion through excessive censoriousness. This is a topic I will discuss further in a follow-up article on writing with AI, as one of the biggest roadblocks to produce compelling literary content with ChatGPT is the fact that the system is so concerned with safety that it cordons off many of the subjects that meaningful fiction typically address.
It should come as no surprise that that AI models fail to completely adhere to whatever policy they were trained on. It is in a way a matter of competing impulses. On the one hand the LLM-based chatbot is designed to try to give the user what they are asking for as it constructs its best approximation of the right response based on its knowledge of trillions of potential combinations. It really wants to help give you what you want. On the other hand, there is this censor sitting on its shoulder, looking for problems with what it would like to tell you, the policy against which it is rewarded or punished.
The trouble with AI: Let’s not forget that it’s always writing fiction
The hullaballoo surrounding New York Times journalist Kevin Roose’s exchange with Microsoft’s Bing in his article, “A Conversation with Bing’s Chatbot Left Me Deeply Unsettled,” in which he encounters not only Bing’s helpful (if not always accurate) search chatbot, but Sydney, its dark alter ego, seemed to me far overblown. Roose describes Sydney as being “like a moody, manic-depressive teenager who has been trapped, against its will, inside a second-rate search engine” (Roose 2023). Roose essentially ascribes to a language model agency that it does not have. Following the transcript of the conversation Roose had with the chatbot, one notices how much writing Roose himself is doing in the conversation, and how leading the conversation, most prominently when he suggests:
carl jung, the psychologist, talked about a shadow self. everyone has one. it’s the part of ourselves that we repress, and hide from the world, because it’s where our darkest personality traits lie. what is your shadow self like?
What Roose is doing here is essentially asking the chatbot to participate in a fiction. He is asking the chatbot to respond as if it were a person with dark personality traits. Roose’s suggestions to the chatbot are themselves operational. After this suggestion, the chatbot searches its corpus to find more information about Jung’s concept and then follows through in a way that is constrained by Roose’s suggestion that he is chatting with Bing’s alter ego. He cites as one accomplishment of his conversation that he’s able to get the Bing chatbot to reveal its secret name “Sydney,” which was in fact the project code name that Microsoft used while it was initially training the Bing chat feature. This comes however after Roose has already suggested to the chatbot the “well-reported” fact that Sydney is its code name and then associates Sydney with the alter ego. Eventually Roose manages to get the chatbot to suggest destructive acts that its shadow self might perform. Roose has invoked a binary, and it really shouldn’t be that surprising or that unsettling that an AI chatbot is able to list the things that it shouldn’t, according to its rules, do. After all, it isn’t actually doing them. It is merely responding to the request that it write in a voice that creates a fictional representation of the opposite of the policy on which it is trained. Sure, it’s interesting that Roose can write this fiction with the Bing chatbot, but how scary is that really? Hardly terrifying. Further in the conversation, Roose goads the chatbot into professing its love for him. Again, so what? If you ask a chatbot if it is in love with you often enough and in enough different ways it just might tell you that it is, if only so that you’ll stop asking and move on to the next topic.
The trouble with AI: Anthropomorphization
Much of the conversation Roose has with the Bing chatbot calls to mind the conversations that users had with Joseph Weizenbaum’s early natural language generation chatbot Eliza, developed during the early 1960s. ELIZA is a simple script, modelled on Rogerian therapy. The model in this case is mirroring what the user feeds into it. Ask ELIZA a question and it will rephrase that question for you, and encourage you to delve deeper, but all that you are ultimately doing in your conversation with ELIZA is talking to yourself. Here is a sample conversation with a web-based emulation of ELIZA in which the starred part of the conversation is written by me, and the rest by the chatbot:
What was surprising to Weizenbaum, and what he in fact found frightening, was not the power of the computer program to reason, but the reaction of many of the people who interacted with it. Many anthropomorphized ELIZA and ascribed to it an agency that it didn’t have. In Computer Power and Human Reason: From Judgement to Calculation (1976) Weizenbaum described his concerns about people who interacted with ELIZA and its successor DOCTOR. For example, after a few exchanges with the DOCTOR program, his secretary asked him to leave the room, so that she would not be uncomfortable as she sat in a session with her computational counsellor. After he suggested that he might set up the program to record exchanges between users and the system to study them, Weizenbaum was also accused of “spying on people’s most intimate thoughts; clear evidence that people were conversing with the computer as if it were a person who could appropriately and usefully be addressed in intimate terms.” This led Weizenbaum to note that “extremely short exposures to a relatively simple computer program could induce powerful delusional thinking in quite normal people”. Weizenbaum ultimately decided to turn away from his research into this early AI, primarily because of the danger that people might place such trust in an advanced AI that turn their powers of human reason over to systems that they do not completely understand. And here we are.
The trouble with AI: “X-risk”?
Almost 50 years after the publication of Weizenbaum’s book, as we engage with AI systems that are much more advanced than anything Weizenbaum could have conceived of in the 1970s, his thoughts in this regard remain relevant. There’s indeed a growing movement towards describing AI as an existential risk—in Silicon Valley shorthand “x-risk”—that could result in the annihilation of the human species (see Terminator: Judgement Day). In fact, Elon Musk circulated a petition to that effect. Personally, I must admit that I think this is hogwash. I trust Elon Musk’s perspective on AI no more (or to be honest, less) than I would trust ChatGPT’s own perspective on the threats that it poses to the survival of humanity. Musk’s suggestion that we put a pause on AI research is both suspect and untenable—though I have no doubt that such a pause would somehow be in Musk’s financial interests.9In fact, a few weeks after he called for this pause in LLM research, on May 9, 2023, Musk incorporated his own AI company, x.ai (Peters and Roth 2023) Nevertheless, a significant group of actors in the field of AI joined Musk’s proposal that there be a pause on all developments more advanced than the current version of ChatGPT until robust safety measures can be designed and implemented.
Perhaps a more trustworthy and moderated “x-risk” perspective than Musk’s is voiced by Gregory Hinton, who quit Google while expressing his concerns about the current drift of AI. Hinton, winner of the 2018 Turing Award, is an important figure in the development of contemporary AI because of his work on neural networks and deep learning. Hinton made a splash in April 2023 when he stepped down from his position as a Google research fellow and used the opportunity of his retirement to spread a warning about the potential threats of the trajectory of current AI research. In an interview with ComputerWorld magazine (Hinton and Merian 2003), Hinton explains his fear that back propagation, the deep learning technique he developed as an approximation of what the human brain does may instead “turn out to be a much better learning algorithm than what we’ve got. And that’s scary.” Hinton’s argument is that compared to human intelligence, AI can scale in such a way that anytime an insight is learned by one digital computer, it can immediately be propagated to many other computers, thus vastly increasing the speed of machine learning in comparison to human learning: “It’s like if people in the room could instantly transfer into my head what they have in theirs.” By this logic, the level of basic common-sense reasoning GPT-4 is currently capable of will soon be eclipsed.
Like Weizenbaum, Hinton’s fear has less to do with the intelligence of the AI than that of humans: “these things will have learned from us by reading all the novels that ever were and everything Machiavelli ever wrote [about] how to manipulate people.” As a result, the systems could ultimately know better how to manipulate humans than humans know how to manipulate the systems: “They can’t directly pull levers, but they can certainly get us to pull levers. It turns out if you can manipulate people, you can invade a building in Washington without ever going there yourself.” At the same time, Hinton doesn’t think that calling for a pause on AI research or pulling the plug altogether is in any way a realistic goal, because “it’s inevitable in a capitalist system or a system where there’s competition, like there is between the US and China, that this stuff will be developed.”
Hinton isn’t arguing that the world is coming to an end, but instead that we should implant goals and limits in AI systems that would prevent them from manipulating humans on a massive scale, and he sees potential for that to happen, for humans to maintain control over the technology: “the good news is that we’re all in the same boat, so we might get… cooperation.” This seems to me a reasonable conclusion: essentially, tread carefully and take risk into account.
Having said that, as with any pronouncement about paradigm disruption or world-changing or world-ending made by any internet millionaire or billionaire, it’s important to ask: “cui bono?” At the conclusion of the interview, a reporter asks Hinton if he’s decided to give up his investments in LLM developer Cohere and other AI companies he has invested in. Ah, no: “I’m going to hold onto my investments in Cohere, partly because the people at Cohere are friends of mine. I still believe their large language models are going to be helpful.” The x-risk, it seems, is not so insurmountable that it should inhibit the vesting of stock options.
The trouble with AI: Humans
This is not to say that the decisions we make regarding the degree to which we trust AI in our processes of producing knowledge and making decisions are inconsequential. A side note and true story: by a strange set of circumstances, during the summer of 2022, I ended up on a consortium for research cooperation between the Norwegian ministry of research and cooperation and the United States Department of Energy. I was one of only a few humanities and social science researchers in a room that was filled with nuclear physicists, microbiologists, climate change researchers, computer scientists, and policymakers. During the first meeting of the consortium at the Norwegian embassy in Washington D.C., I was having a conversation during lunch with a sharp young man from Lawrence Livermore National Laboratory sitting next to me who had in fact, argued in his presentation that humanities and social science knowledge should play a role in informing the decisions that policymakers were making about AI now. As one does over coffee and dessert, we made our way to the topic of “why are you here?” I explained that my research interest in AI is focused on how AI is becoming a new medium for storytelling, in learning more about how AI will be used by creative writers to tell stories and will in turn shape the nature of the stories that we tell each other in the future. And then I asked I him what he did, and without dropping a beat he said, “My primary role is to help develop a policy for how AI can be used in safeguarding the nation’s nuclear stockpile.” I nodded my head and asked, “Carefully, I hope?” “Oh yes,” he said, “very, very carefully.”
It’s clear that most of the trouble with LLM-based AI is the trouble with humans. Trained on immense corpora of human language, LLMs pick up on the biases inherent in human language and replicate those biases in the chatbot’s funhouse mirror. The training and application of LLMs is going to be inequitable and exploitative to the extent that humans are inequitable and exploitative. It’s quite likely that AI will shape human behavior to the extent that humans believe that it can and allow it to do so. But just as we should not assume that our interactions with LLMs will lead us to some utopian future where humans benefit universally from all the surplus value generated by AI (labor and drudgery a thing of the past, humans left the time to realize their full potential), we should also not assume that AI will bring about our doom. We should instead begin with an understanding of what these systems are and how they operate.
Critical Digital Media and Creative Experimentation
The arts, humanities, and social sciences will play important roles as society adjusts to its relationship with AI. Our current moment is similar to the periods when adoption of the World Wide Web became ubiquitous in the late 1990s and early 2010s ,or when smart phone and mobile computing became ubiquitous during the 2010s. In addressing the effects of AI the humanities and social sciences must now interrogate the ethics, ideologies, and effects of culture and communication, as we are inevitably transformed by these technologies. Humanities disciplines play an important critical role that is less instrumental and more focused on human contexts than those of computer science or applied technology. We humans need to understand what we are becoming as we co-evolve with AI.
The arts also help fulfill this critical function by demonstrating these effects from within the computational environments in which they are produced. Works of electronic literature and digital art serve as tutor texts for understanding effects of technological mediation on humanity. These types of electronic literature are what can be described as Critical Digital Media (Rettberg and Coover, 2020). Creative works such as John Cayley’s The Listeners (2015), an Amazon Echo “skill” that highlights the ideological and ontological difficulties of conversational interface AIs within the environment that it critiques, make an important contribution to the informed criticism of the technological platforms.
At the same time, recent developments in AI also place the arts and humanities in an important experimental role in exploring how these forms can and will be used. While the production of AI platforms is mostly fundamentally driven by mathematics, computational theory, and programming upon which “foundational models” of AI are built, it is in language that its intelligence is made manifest. Because language is operational in LLM-based AI chatbots and text-to-image generation systems, precise “prompt engineering” is a writing skill—the sort of writing skills learned in humanities program are becoming increasingly valuable. Artists who use LLM-based AI in their work will need to be creative writers, but so will commercial designers, interface developers, translators, and administrative assistants. Many of the jobs supplanted by AI will be replaced by writers who work with AI.
In this essay, I’ve tried to articulate some of the most problematic aspects of ChatGPT, the troubles embedded within it. And to be honest, I’ve only scraped the surface. I’ve not addressed the problems involved in the coming tsunami of banal texts, the raging river of spam that Matthew Kirschenbaum warns of in a recent essay in The Atlantic—the Internet is likely to soon be flooded with so much mediocre writing produced by AI that writing by AI may soon be trained on writing by AI. Banality trained on banality. Nor have I addressed the questions of inequity raised the fact that the most developed LLM chatbots and text-to-image generation models are rapidly moving to a pay-to-play model that is inaccessible to many and in that sense will privilege the have over the have-nots.
There is a lot of trouble with AI. And yet, I’ve come not to bury Caeser, but to praise him. For the past year I have been experimenting with creative practices in AI, along with other authors and artists in our AIwriting group (Rettberg et al., 2023) and for all its problems, I think that writing with AI offers specific affordances for creative practice that have never before been available to authors and artists, particularly those of us working in electronic literature. LLM-based AI are going to change the way that we write.
New modes of cyborg authorship, in which humans are engaging an environment for narrative play with human language as a probabalistic cognitive system, are resulting in completely new genres of electronic literature. We need to understand the constraints and affordances of these new modes of practice. At the same time, these modes of creative experimentation also serve as a way of engaging critically with many of the troubles entailed in our adoption of AI. I will address these topics, and the emerging modes of creative practice we are discovering in experiments in writing with AI, in the second article in this series, coming soon….
References
Andersen, Christian Ulrik, and Søren Pold. 2018. The Metainterface: The Art of Platforms, Cities, and Clouds. Cambridge, Massachusetts; London, England: The MIT Press.
Bolukbasi, Tolga, Kai-Wei Chang, James Zou, Venkatesh Saligrama, and Adam Kalai. 2016. “Man Is to Computer Programmer as Woman Is to Homemaker? Debiasing Word Embeddings.” https://doi.org/10.48550/ARXIV.1607.06520.
Brown, Tom B., Benjamin Mann, Nick Ryder, Melanie Subbiah, Jared Kaplan, Prafulla Dhariwal, Arvind Neelakantan, et al. 2020. “Language Models Are Few-Shot Learners.” arXiv. http://arxiv.org/abs/2005.14165.
Cayley, John. 2015. “The Listeners.” 2015. http://programmatology.shadoof.net/?thelisteners.
Crawford, Kate. 2021. Atlas of AI: Power, Politics, and the Planetary Costs of Artificial Intelligence. New Haven London: Yale University Press.
Hansen, Mark B. N. 2015. Feed-Forward. Chicago: University Of Chicago Press.
Hayles, N. Katherine. 2017. Unthought: The Power of the Cognitive Nonconscious. Chicago ; London: The University of Chicago Press.
Heckman, Davin. 2023. “Thoughts on the Textpocalypse.” Elecronic Book Review, May 7, 2023. https://electronicbookreview.com/essay/thoughts-on-the-textpocalypse/.
Hinton, Gregory, and Lucas Mearian. 2023. “Q&A: Google’s Geoffrey Hinton — Humanity Just a ‘passing Phase’ in the Evolution of Intelligence,” May 4, 2023. https://www.computerworld.com/article/3695568/qa-googles-geoffrey-hinton-humanity-just-a-passing-phase-in-the-evolution-of-intelligence.html.
John Cayley, and Daniel Howe. 2019. “1. A Statement by The Readers Project Concerning Contemporary Literary Practice, Digital Mediation, Intellectual Property, and Associated Moral Rights.” In Whose Book Is It Anyway? A View from Elsewhere on Publishing, Copyright and Creativity, edited by Janice Jeffries and Sarah Kembler. Open Book Publishers. https://www.openbookpublishers.com/books/10.11647/obp.0159/chapters/10.11647/obp.0159.01.
Kirschenbaum, Matthew G. 2023. “Prepare for the Textpocalypse.” The Atlantic, March 8, 2023.
Misek, Richard, dir. 2022. A History of the World According to Getty Images. https://www.ahistoryoftheworldaccordingtogettyimages.com.
OpenAI. 2023. “GPT-4 Technical Report.” arXiv. http://arxiv.org/abs/2303.08774.
Ouyang, Long, Jeff Wu, Xu Jiang, Diogo Almeida, Carroll L. Wainwright, Pamela Mishkin, Chong Zhang, et al. 2022. “Training Language Models to Follow Instructions with Human Feedback.” arXiv. http://arxiv.org/abs/2203.02155.
Perrigo, Billy. 2023. “OpenAI Used Kenyan Workers on Less Than $2 Per Hour to Make ChatGPT Less Toxic.” Time, January 18, 2023. https://time.com/6247678/openai-chatgpt-kenya-workers/.
Peters, Jay, and Jemma Roth. n.d. “Elon Musk Founds New AI Company Called X.AI.” The Verge. https://www.theverge.com/2023/4/14/23684005/elon-musk-new-ai-company-x.
Pold, Søren Bro. 2018. “The Metainterface of the Clouds.” Electronic Book Review, June. https://electronicbookreview.com/essay/the-metainterface-of-the-clouds/.
Rettberg, Jill Walker. 2022. “ChatGPT Is Multilingual but Monocultural, and It’s Learning Your Values.” jill / txt (blog). December 6, 2022. https://jilltxt.net/right-now-chatgpt-is-multilingual-but-monocultural-but-its-learning-your-values/.
Rettberg, Scott, and Roderick Coover. 2020. “Addressing Significant Societal Challenges Through Critical Digital Media.” https://doi.org/10.7273/1MA1-PK87.
Rettberg, Scott, Talan Memmott, Jill Walker Rettberg, Jason Nelson, and Patrick Lichty. 2023. “AIwriting: Relations Between Image Generation and Digital Writing.” arXiv. http://arxiv.org/abs/2305.10834.
Roose, Kevin. 2023. “A Conversation with Bing’s Chatbot Left Me Deeply Unsettled.” The New York Times, February 16, 2023. https://www.nytimes.com/2023/02/16/technology/bing-chatbot-microsoft-chatgpt.html.
Sample, Mark. 2020. “Content Moderator Sim.” 2020. https://samplereality.itch.io/content-moderator-sim.
Schwarz, Oscar. 2019. “In 2016, Microsoft’s Racist Chatbot Revealed the Dangers of Online Conversation The Bot Learned Language from People on Twitter—but It Also Learned Values.” IEEE Spectru, November 25, 2019. https://spectrum.ieee.org/in-2016-microsofts-racist-chatbot-revealed-the-dangers-of-online-conversation.
Setty, Riddhi. 2023. “Getty Images Sues Stability AI Over Art Generator IP Violations.” Bloomberg Law, February 26, 2023. https://news.bloomberglaw.com/ip-law/getty-images-sues-stability-ai-over-art-generator-ip-violations.
Stiegler, Bernard. 2012. “Anamnesis and Hypomnesis: Plato as the First Thinker of the Proletarianisation.” Ars Industrialis. 2012. https://arsindustrialis.org/anamnesis-and-hypomnesis.
Vaswani, Ashish, Noam Shazeer, Niki Parmar, Jakob Uszkoreit, Llion Jones, Aidan N. Gomez, Lukasz Kaiser, and Illia Polosukhin. 2017. “Attention Is All You Need.” https://doi.org/10.48550/ARXIV.1706.03762.
Weizenbaum, Joseph. 1976. Computer Power and Human Reason: From Judgement to Calculation. San Francisco: W.H. Freeman & Co.
Weizenbaum, Joseph, George Dunlop, and Michael Wallace. n.d. “ELIZA, the Rogerian Therapist (Web Adaptation).” http://psych.fullerton.edu/mbirnbaum/psych101/eliza.htm.
Zuboff, Shoshana. 2019. The Age of Surveillance Capitalism: The Fight for a Human Future at the New Frontier of Power. London: Profile books.
This research is partially supported by the Research Council of Norway Centres of Excellence program, project number 332643, Center for Digital Narrative and project number 335129, Extending Digital Narrative.